11 CE Credits (credit only available for entire issue). SPECIAL ISSUE: Human Brain Connectivity in the Modern Era. JINS 22:2 (2016)
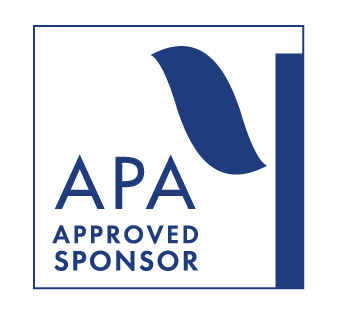
- To identify and understand the primary methods by which one can examine human structural and/or functional brain connectivity.
- To identify and understand the primary benefits and challenges associated with the different methods used to assess human structural and functional brain connectivity.
- To understand relationships between normative variations in structural and/or functional connectivity and behavior.
- To identify the populations, brain systems, and behaviors associated with alterations in functional and structural connectivity.
- To describe the specificity across clinical domains of associations and the potential mechanisms by which functional and/or structural connectivity influences behavior.
- To describe similarities and differences in structural versus functional connectivity abnormalities across clinical populations.
Target Audience: | Intermediate |
---|---|
Availability: | Date Available: 2016-02-17 |
You may obtain CE for this JINS package at any time. | |
Offered for CE | Yes |
Cost | Members $110 |
Non-Members $165 | |
Refund Policy | This JINS package is not eligible for refunds |
CE Credits | 11 |
Last year (2015) commemorated the 50th anniversary of Norman Geschwind’s seminal papers in Brain on “Disconnexion syndromes in animals and man” (Geschwind, 1965a, 1965b). In the past 50 years, huge advances have occurred in the tools and technologies available for the in vivo assessment of both structural and functional connectivity in the human brain, including diffusion imaging for examining structural brain connectivity, and functional magnetic resonance imaging (fMRI), electroencephalogram (EEG), and magneto-encephalogram (MEG) approaches to understanding functional brain connectivity. This has led to a dramatic increase in our understanding of the core principles of human brain connectivity and their relationship to cognitive, emotional, motor, and sensory function in health, and more recently, in clinical populations.
Facilitated by the availability of novel imaging techniques, this enhanced understanding of brain–behavior relationships reflects a fundamental conceptual shift. Basic and translational research examining task-related brain activation has been remarkably informative in terms of our understanding of the neural substrates of particular cognitive and affective processes and how these may go awry in conditions associated with impaired brain function. However, over time, it has become clear that rarely does any particular cognitive or affective process require only a single brain region, and rarely is any particular form of cognitive or behavioral dysfunction associated with disruption of only a single brain region.
Furthermore, basic neuroscience research has long made it clear that activity in any individual brain region (or any individual neuron!) is the result of inputs from and outputs to different areas of the brain. Such realizations have led to a shift in focus on neural circuits rather than on specific brain regions. More specifically, this shift has been to questions about the relationship between and among different brain regions in producing successful cognitive and affective function in health, and the ways in which abnormalities at the level of circuits contribute to the development and maintenance of specific neuropsychological impairments. The growing work on the role of brain oscillations in coordinating activity within and between neutral networks (Canavier, 2015; Ketz, Jensen, & O’Reilly, 2015; Pittman-Polletta, Kocsis, Vijayan, Whittington, & Kopell, 2015; Uhlhaas & Singer, 2015) is consistent with such hypotheses that localize neuropsychological impairments at the circuit level of function rather than within specific individual brain regions.
Aimed at highlighting this conceptual shift, this special issue has three specific goals. The first is to provide a brief overview of the current methodological and analytic tools available for understanding both normative and dysfunctional human brain connectivity. As outlined in the article by Lowe and colleagues, and to some extent in the article by Hayes and colleagues, we have seen major advances in our ability to image white matter connections in the human brain, including state-of-the art techniques that now allow researchers to follow the path of white matter connections through areas where many different fiber tracts merge, dramatically improving our ability to understand the structural basis of both short and long range communication within brain circuits.
Furthermore, as also described in the article by Lowe and colleagues, the last 30 years have also seen the emergence of methods for studying functional brain connectivity, or the covariance of spontaneous brain activity across brain regions. Originally, the concept of functional connectivity was applied to simultaneous recordings of neuronal spike trains (Gerstein & Perkel, 1969; Gerstein, Perkel, & Subramanian, 1978; Perkel, Gerstein, & Moore, 1967), which are thought to contribute to the functional connectivity observed in humans using non-invasive neuroimaging methods. A main inference of functional connectivity is that, if two regions have highly correlated neuronal activity (i.e., have high functional connectivity), then they are more likely to engage in a common set of processing mechanisms. As such, functional connectivity provides a tool for understanding what brain regions may be communicating while engaging in specific cognitive or affective processes, and therefore what brain circuits support performance and ability in different domains of cognition, emotion and/or social processing.
A major breakthrough in the development and application of functional connectivity methods for humans came in 1995, when Biswal and colleagues reported that spontaneous activity from regions in the right and left motor cortices was highly correlated even while an individual was resting (Biswal, Yetkin, Haughton, & Hyde, 1995). Of interest, this correlated activity was observed in the spontaneous low-frequency fluctuations of the BOLD signal (0.01–0.10 Hz), a frequency band that has often been discarded as noise in task-based studies, although such correlations can be seen at other frequencies as well. This work spurred a major field of exploration of what is now referred to as resting state functional connectivity. Importantly, this resting brain state activity is thought to consume a major portion of the body’s energy (~20%), despite the brain only being 2% of the body’s total mass (Fox & Raichle, 2007).
Furthermore, changes in metabolism due to engagement in a specific task are typically less than 5%. Thus, ongoing resting state activity may provide a rich source of pathology-related variability over and above changes observed in the context of task performance (Fox & Raichle, 2007). In addition, there is also robust work demonstrating that a large portion of the trial-to-trial variability in task-related activity reflects coherent and organized spontaneous fluctuations in brain activity (Fox, Snyder, Zacks, & Raichle, 2006), providing another piece of evidence that this is a meaningful source of variation in human brain function.
One of the key aspects of resting state functional connectivity that has spurred interest in this aspect of brain function is the realization that it reveals intrinsically organized networks of brain regions that are consistently functionally connected, even in the absence of task-induced perturbations in ongoing brain activity (Fox et al., 2005). This has been supported by numerous “network” mapping studies that have identified consistent, robust and reproducible networks of brain regions that show coordinated activity at rest (Buckner, Krienen, Castellanos, Diaz, & Yeo, 2011; Choi, Yeo, & Buckner, 2012; Craddock, James, Holtzheimer, Hu, & Mayberg, 2012; Gordon et al., 2016; Laird et al., 2011; Power et al., 2011; Smith et al., 2012; Wig et al., 2014; Yeo et al., 2011). These include the default mode network, the frontal parietal network, the cingulo-opercular network, and the dorsal attention network, to name a few.
Importantly, many of these networks map closely or at least partially, to networks that have been identified in task-based studies, providing validation to the functional meaningfulness of this coordinated activity (Laird et al., 2011). Such functional connectivity networks have their basis in part in structural connectivity (Betzel et al., 2014; Hermundstad et al., 2013; Horn & Blankenburg, 2015; Horn, Ostwald, Reisert, & Blankenburg, 2014; Messe, Rudrauf, Giron, & Marrelec, 2015; Miranda-Dominguez et al., 2014), but are not isomorphic with regions that show direct structural connectivity. As such, the mapping of resting state networks to known task networks has led to the hypothesis that resting state networks reflect in part organized interactions that arise from a history of co-activation over development (Power et al., 2011). Such networks are now frequently referred to as “intrinsic” connectivity networks, given that they are not dependent on performance of a particular task for identification, are present even at rest and are relatively consistent across task and environmental states. Many of the articles in this special issue focus on these networks to understand the source of pathology in various forms of brain dysfunction.
A second goal of this special issue is to provide integrative and synthetic summaries of the state-of-the field in our current understanding of brain connectivity impairments associated with both neurological and neuropsychiatric disorders. As such, the article by Hayes and colleagues reviews the current state of the literature on understanding traumatic brain injury (TBI) as a disorder of brain connectivity. Much of the work on TBI has long focused on the impairments in white matter that may occur as a result of the different traumas that can lead to TBI, with the recognition that damage to white matter connections may be occurring even when focal lesions are not found. The article by Hayes et al. provides evidence that TBI is indeed associated with white matter damage. The corpus callosum seems to be particularly vulnerable to the forces that lead to TBI, but the existing evidence suggest that the damage associated with TBI can be quite diffuse and present in many different tracts, perhaps dependent on the nature and severity of the causal trauma. This white matter damage persists even into chronic phases of TBI and has been associated with cognitive and functional impairments. Furthermore, TBI is also associated with alterations in functional connectivity, with several studies highlighting disrupted connectivity of the default mode network and its contribution to post concussion symptoms and impairments in attention focus.
The review article by Teipel and colleagues highlights the potentially important roles of both structural and functional connectivity in the evolution of Alzheimer’s disease (AD). As described in this review article, there are now numerous cross-sectional and longitudinal studies relevant to AD documenting impairments in white matter, including abnormalities in limbic tracts such as the fornix, the uncinate fasciculus and the posterior and parahippocampal fibers of the cingulum. Furthermore, there is growing evidence of abnormalities in resting state connectivity, with several studies demonstrating abnormalities of the default mode network. Abnormalities in connectivity have also been documented using coherence measures in EEG. Importantly, there is increasing evidence of links between structural and functional connectivity impairments in the default mode network in AD. As noted by Teipel et al., these findings are helping us to understand the potential etiological mechanisms of AD relevant pathology, as well as the longitudinal evolution of the disease. However, more work is needed for such findings to have direct clinical application. On the opposite end of the life span, Koyama and colleagues also argue for the importance of connectivity in understanding the developmental trajectory of children who may be at risk for the development of a range of disorders that impact brain function.
The third goal of this special issue is to present cutting-edge empirical findings on the nature and role of connectivity impairments in understanding variations in cognitive and emotional function, as well as in a range of clinical populations. To this end, the special issue includes several novel empirical findings on ways in which either structural or functional connectivity contributes to cognitive and affective function in both health and disease. Several papers provide data on the relationships between behavior and brain connectivity in healthy individuals, providing evidence for the functional significance of individual variation in brain connectivity. For example, Unger and colleagues demonstrate that individual differences in the integrity of the inferior longitudinal fasciculus (ILF) and the inferior fronto-occipital fasciculus (IFOF) predict emotion recognition performance as well as memory for emotional faces. Ly and colleagues provide evidence for a relationship between the microstructure of the fornix and both task-related connectivity and performance during episodic recognition in healthy aging, providing an interesting model of potential pathways to individual differences in memory.
The other empirical papers in this special issue demonstrate how abnormalities in structural and or functional connectivity may contribute to behavioral and affective impairments in various forms of brain pathology. For example, Putcha and colleagues provide intriguing evidence that altered coupling of the salience and default mode networks relates to cognitive function in both healthy individuals and those with Parkinson’s disease. The salience and default mode networks are typically “anti-correlated,” and it is thought that the ability to down-regulate activity of the default mode network during cognitive tasks is important for effective performance. The finding by Putcha et al. that a lack of anti-correlation was associated with impaired function in executive, memory, and psychomotor speed domains is consistent with this hypothesis.
Relatedly, Dobryakova and colleagues provide evidence that altered connectivity among frontal-parietal regions predicts impaired processing speed in multiple sclerosis. Rao and colleagues examined connectivity of hippocampus to both fronto-temporal and fronto-parietal regions among individuals with remitted major depression compared to controls during semantically cued episodic memory performance. They found evidence for impaired memory performance and altered hippocampal connectivity among the previously depressed individuals, along with evidence for disrupted relationships between hippocampal connectivity and memory.
Finally, three articles bring to bear a graph theoretic approach to understanding how alterations in neural networks contribute to the development of brain pathology and associated impairments in cognitive function. Graph theory is a branch of mathematics that provides algorithms for determining metrics that characterize networks at both global and local levels of function. One major advantage of graph theory is its flexibility; algorithms can be applied to functional connectivity data, structural connectivity data, as well as to data obtained using MEG, EEG, or fMRI, allowing for a convergence of findings across differing modalities.
Furthermore, network science allows for the characterization of dynamic processes through single metrics, arguably providing more powerful and parsimonious descriptions of heterogeneous data than the previously discussed approaches. Although much work is needed to further validate the use of graph theory for interpreting brain connectivity data, network science represents an exciting new field of research that is increasingly showing associations between network metrics and behavior, as well as abnormalities in networks metrics in individuals with psychiatric and neurological disorders.
Yeo and colleagues use the power of a graph theory approach to show that the structural brain networks of individuals with schizophrenia are characterized by longer characteristic path lengths (suggesting a longer transit time for information) and reduced overall connectivity. These graph theory metrics predicted overall cognitive ability in healthy individuals as well as in individuals with schizophrenia, and the overall connectivity reduction mediated the relationship between diagnosis and overall cognitive ability.
Furthermore, Yeo et al. found that a genetic measure reflecting mutation load predicted both longer characteristic path length and global cognitive ability. Sedeno and colleagues also took a graph theory approach to understanding the mechanisms that might contribute to cognitive and social impairments in fronto-temporal dementia. Used resting state functional connectivity, they showed decreased network centrality, a measure of the importance of a node or brain region’s role in a network, in the fronto-temporal–insular network. Furthermore, this network centrality was associated with social cognition and executive function in individuals with fronto-temporal dementia and healthy individuals. Finally, Han and colleagues examined networks associated with goal-directed behavior in individuals with chronic TBI. They observed markedly disrupted long-range interhemispheric and between-network connectivity between the default mode network, the dorsal attention network, and the frontoparietal control network, with as result reduced global and local efficiency.
In summary, we believe that the articles presented in this special issue provide an important entryway into the burgeoning literature on the role of neural networks in cognition and the nature of alterations in circuit level structural and functional connectivity associated with brain pathology. As noted in many of the articles, this field continues to evolve as both the acquisition and analyses methods develop and expand, but the existing evidence points to the functional relevance of these networks to understanding variations in normal development and the critical importance of their structural and functional brain disruption as a means of understanding pathophysiology and potential pathways for intervention or even prevention.
Connectionist theories of brain function took hold with the seminal contributions of Norman Geschwind a half century ago. Modern neuroimaging techniques have expanded the scientific interest in the study of brain connectivity to include the intact as well as disordered brain.
In this review, we describe the most common techniques used to measure functional and structural connectivity, including resting state functional MRI, diffusion MRI, and electroencephalography and magnetoencephalography coherence. We also review the most common analytical approaches used for examining brain interconnectivity associated with these various imaging methods.
This review presents a critical analysis of the assumptions, as well as methodological limitations, of each imaging and analysis approach.
The overall goal of this review is to provide the reader with an introduction to evaluating the scientific methods underlying investigations that probe the human connectome. (JINS, 2016,22, 105–119)
- Abou-Elseoud, A., Starck, T., Remes, J., Nikkinen, J., Tervonen, O., & Kiviniemi, V. (2010). The effect of model order selection in group PICA. Human Brain Mapping, 31, 1207–1216. Google Scholar PubMed
- Achard, S., Salvador, R., Whitcher, B., Suckling, J., & Bullmore, E. (2006). A resilient, low-frequency, small-world human brain functional network with highly connected association cortical hubs. The Journal of Neuroscience, 26(1), 63–72. CrossRef Google Scholar
- Alexander-Bloch, A., Giedd, J.N., & Bullmore, E. (2013). Imaging structural co-variance between human brain regions. [Review]. Nature Reviews Neuroscience, 14(5), 322–336. CrossRef Google Scholar
- Allen, E.A., Damaraju, E., Plis, S.M., Erhardt, E.B., Eichele, T., & Calhoun, V.D. (2014). Tracking Whole-brain connectivity dynamics in the resting state. Cerebral Cortex, 24(3), 663–676. CrossRef Google Scholar PubMed
- Allen, E.A., Erhardt, E.B., Damaraju, E., Gruner, W., Segall, J.M., Silva, R.F., & Calhoun, V.D. (2011). A baseline for the multivariate comparison of resting-state networks. Frontiers in Systems Neuroscience, 5, 2. CrossRef Google Scholar PubMed
- Amunts, K., Lepage, C., Borgeat, L., Mohlberg, H., Dickscheid, T., Rousseau, M.-É., & Evans, A.C. (2013). BigBrain: An ultrahigh-resolution 3D human brain model. Science, 340(6139), 1472–1475. CrossRef Google Scholar PubMed
- Assaf, Y., Blumenfeld-Katzir, T., Yovel, Y., & Basser, P.J. (2008). AxCaliber: A method for measuring axon diameter distribution from diffusion MRI. Magnetic Resonance in Medicine, 59(6), 1347–1354. CrossRef Google Scholar PubMed
- Assemlal, H.E., Tschumperle, D., Brun, L., & Siddiqi, K. (2011). Recent advances in diffusion MRI modeling: Angular and radial reconstruction. Medical Image Analysis, 15(4), 369–396. CrossRef Google Scholar PubMed
- Barazany, D., Basser, P.J., & Assaf, Y. (2009). In vivo measurement of axon diameter distribution in the corpus callosum of rat brain. Brain, 132(Pt 5), 1210–1220. CrossRef Google Scholar
- Basser, P.J., Mattiello, J., & LeBihan, D. (1994). MR diffusion tensor spectroscopy and imaging. Biophysical Journal, 66(1), 259–267. CrossRef Google Scholar
- Basser, P.J., Pajevic, S., Pierpaoli, C., Duda, J., & Aldroubi, A. (2000). In vivo fiber tractography using DT-MRI data. Magnetic Resonance in Medicine, 44(4), 625–632.3.0.CO;2-O>CrossRef Google Scholar
- Bassett, D.S., & Bullmore, E. (2006). Small-world brain networks. Neuroscientist, 12(6), 512–523. CrossRef Google Scholar PubMed
- Bassett, D.S., Meyer-Lindenberg, A., Achard, S., Duke, T., & Bullmore, E. (2006). Adaptive reconfiguration of fractal small-world human brain functional networks. Proceedings of the National Academy of Sciences of the United States of America, 103(51), 19518–19523. CrossRef Google Scholar
- Beall, E.B., & Lowe, M.J. (2007). Isolating physiologic noise sources with independently determined spatial measures. Neuroimage, 37(4), 1286–1300. CrossRef Google Scholar
- Beall, E.B., & Lowe, M.J. (2010). The non-separability of physiologic noise in functional connectivity MRI with spatial ICA at 3T. Journal of Neuroscience Methods, 191(2), 263–276. CrossRef Google Scholar PubMed
- Beall, E.B., & Lowe, M.J. (2014). SimPACE: Generating simulated motion corrupted BOLD data with synthetic-navigated acquisition for the development and evaluation of SLOMOCO: A new, highly effective slicewise motion correction. Neuroimage, 101, 21–34. CrossRef Google Scholar PubMed
- Beckmann, C.F., & Smith, S.M. (2005). Tensorial extensions of independent component analysis for multisubject FMRI analysis. Neuroimage, 25(1), 294–311. CrossRef Google Scholar PubMed
- Behrens, T.E., Berg, H.J., Jbabdi, S., Rushworth, M.F., & Woolrich, M.W. (2007). Probabilistic diffusion tractography with multiple fibre orientations: What can we gain? Neuroimage, 34(1), 144–155. CrossRef Google Scholar PubMed
- Behrens, T.E., & Sporns, O. (2012). Human connectomics. Current Opinion in Neurobiology, 22(1), 144–153. CrossRef Google Scholar PubMed
- Bendat, J.S., & Piersol, A.G. (2000). Random data. Analysis and measurement procedures (3rd ed.). New York: John Wiley & Sons. Google Scholar
- Biswal, B.B., & Hudetz, A.G. (1996). Synchronous oscillations in cerebrocortical capillary red blood cell velocity after nitric oxide synthase inhibition. Microvascular Research, 52(1), 1–12. CrossRef Google Scholar PubMed
- Biswal, B.B., Hudetz, A.G., Yetkin, F.Z., Haughton, V.M., & Hyde, J.S. (1997). Hypercapnia reversibly suppresses low-frequency fluctuations in the human motor cortex during rest using echo-planar MRI. Journal of Cerebral Blood Flow & Metabolism, 17(3), 301–308. CrossRef Google Scholar PubMed
- Biswal, B.B., Van Kylen, J., & Hyde, J.S. (1997). Simultaneous assessment of flow and BOLD signals in resting-state. NMR in Biomedicine, 10(4-5), 165–170.3.0.CO;2-7>CrossRef Google Scholar PubMed
- Biswal, B.B., Yetkin, F.Z., Haughton, V.M., & Hyde, J.S. (1995). Functional connectivity in the motor cortex of resting human brain. Magnetic Resonance in Medicine, 34(4), 537–541. CrossRef Google Scholar PubMed
- Boccaletti, S., Latora, V., Moreno, Y., Chavez, M., & Hwang, D.U. (2006). Complex networks: Structure and dynamics. Physics Reports, 424(4–5), 175–308. CrossRef Google Scholar
- Boubela, R.N., Kalcher, K., Huf, W., Kronnerwetter, C., Filzmoser, P., & Moser, E. (2013). Beyond noise: Using temporal ICA to extract meaningful information from high-frequency fMRI signal fluctuations during rest. Frontiers in Human Neuroscience, 7, 168. CrossRef Google Scholar
- Brookes, M.J., Hale, J.R., Zumer, J.M., Stevenson, C.M., Francis, S.T., Barnes, G.R., & Nagarajan, S.S. (2011). Measuring functional connectivity using MEG: Methodology and comparison with fcMRI. Neuroimage, 56(3), 1082–1104. CrossRef Google Scholar PubMed
- Budde, M.D., Kim, J.H., Liang, H.F., Schmidt, R.E., Russell, J.H., Cross, A.H., && Song, S.K. (2007). Toward accurate diagnosis of white matter pathology using diffusion tensor imaging. Magnetic Resonance in Medicine, 57(4), 688–695. CrossRef Google Scholar PubMed
- Bullmore, E.T., Brammer, M.J., Rabe-Hesketh, S., Curtis, V.A., Morris, R.G., Williams, S.C., & McGuire, P.K. (1999). Methods for diagnosis and treatment of stimulus-correlated motion in generic brain activation studies using fMRI. Human Brain Mapping, 7(1), 38–48.3.0.CO;2-Q>CrossRef Google Scholar
- Bullmore, E.T., & Sporns, O. (2009). Complex brain networks: Graph theoretical analysis of structural and functional systems. Nature Reviews Neuroscience, 10(3), 186–198. CrossRef Google Scholar PubMed
- Buzsaki, G. (2006). Rhythms of the brain. New York: Oxford University Press. CrossRef Google Scholar
- Calhoun, V.D., & Adali, T. (2012). Multi-subject independent component analysis of fMRI: A decade of intrinsic networks, default mode, and neurodiagnostic discovery. IEEE Reviews in Biomedical Engineering, 5, 60–72. CrossRef Google Scholar
- Calhoun, V.D., Adali, T., Pearlson, G.D., & Pekar, J.J. (2001). A method for making group inferences from functional MRI data using independent component analysis. Human Brain Mapping, 14(3), 140–151. CrossRef Google Scholar PubMed
- Calhoun, V.D., & Allen, E. (2013). Extracting intrinsic functional networks with feature-based group independent component analysis. Psychometrika, 78(2), 243–259. CrossRef Google Scholar PubMed
- Calhoun, V.D., Kiehl, K.A., & Pearlson, G.D. (2008). Modulation of temporally coherent brain networks estimated using ICA at rest and during cognitive tasks. Human Brain Mapping, 29(7), 828–838. CrossRef Google Scholar
- Calhoun, V.D., Miller, R., Pearlson, G., & Adalı, T. (2014). The chronnectome: Time-varying connectivity networks as the next frontier in fMRI data discovery. Neuron, 84(2), 262–274. CrossRef Google Scholar PubMed
- Catani, M., Howard, R.J., Pajevic, S., & Jones, D.K. (2002). Virtual in vivo interactive dissection of white matter fasciculi in the human brain. Neuroimage, 17(1), 77–94. CrossRef Google Scholar
- Chang, C., & Glover, G.H. (2010). Time–frequency dynamics of resting-state brain connectivity measured with fMRI. Neuroimage, 50(1), 81–98. CrossRef Google Scholar PubMed
- Cohen, D., & Cuffin, B.N. (1983). Demonstration of useful differences between magnetoencephalogram and electroencephalogram. Electroencephalography and Clinical Neurophysiology, 56(1), 38–51. CrossRef Google Scholar PubMed
- Cole, D.M., Smith, S.M., & Beckmann, C.F. (2010). Advances and pitfalls in the analysis and interpretation of resting-state FMRI data. Frontiers in Systems Neuroscience, 4, 8. Google Scholar PubMed
- Colizza, V., Flammini, A., Serrano, M.A., & Vespignani, A. (2006). Detecting rich-club ordering in complex networks. [10.1038/nphys209]. Nature Physics, 2(2), 110–115. CrossRef Google Scholar
- Conturo, T.E., Lori, N.F., Cull, T.S., Akbudak, E., Snyder, A.Z., Shimony, J.S., & Raichle, M.E. (1999). Tracking neuronal fiber pathways in the living human brain. Proceedings of the National Academy of Sciences of the United States of America, 96(18), 10422–10427. CrossRef Google Scholar
- Cooper, R., Crow, H.J., Walter, W.G., & Winter, A.L. (1966). Regional control of cerebral vascular reactivity and oxygen supply in man. Brain Research, 3(2), 174–191. CrossRef Google Scholar PubMed
- Cordes, D., Haughton, V.M., Arfanakis, K., Carew, J.D., Turski, P.A., Moritz, C.H., & Meyerand, M.E. (2001). Frequencies contributing to functional connectivity in the cerebral cortex in “resting-state” data. AJNR American Journal of Neuroradiology, 22(7), 1326–1333. Google Scholar
- Cordes, D., Haughton, V.M., Arfanakis, K., Wendt, G.J., Turski, P.A., Moritz, C.H., & Meyerand, M.E. (2000). Mapping functionally related regions of brain with functional connectivity MR imaging. AJNR American Journal of Neuroradiology, 21(9), 1636–1644. Google Scholar
- Cordes, D., Nandy, R.R., Schafer, S., & Wager, T.D. (2014). Characterization and reduction of cardiac- and respiratory-induced noise as a function of the sampling rate (TR) in fMRI. Neuroimage, 89, 314–330. CrossRef Google Scholar
- Cox, R.W. (1996). AFNI: Software for analysis and visualization of functional magnetic resonance neuroimages. Computers and Biomedical Research, 29(3), 162–173. CrossRef Google Scholar PubMed
- Craddock, R.C., Jbabdi, S., Yan, C.-G., Vogelstein, J.T., Castellanos, F.X., Di Martino, A., & Milham, M.P. (2013). Imaging human connectomes at the macroscale. Nature Methods, 10(6), 524–539. CrossRef Google Scholar
- Crossley, N.A., Mechelli, A., Scott, J., Carletti, F., Fox, P.T., McGuire, P., && Bullmore, E.T. (2014). The hubs of the human connectome are generally implicated in the anatomy of brain disorders. Brain, 137(Pt 8), 2382–2395. CrossRef Google Scholar PubMed
- Csermely, P., London, A., Wu, L.-Y., & Uzzi, B. (2013). Structure and dynamics of core/periphery networks. Journal of Complex Networks, 1(2), 93–123. CrossRef Google Scholar
- Damaraju, E., Allen, E.A., Belger, A., Ford, J.M., McEwen, S., Mathalon, D.H., & Calhoun, V.D. (2014). Dynamic functional connectivity analysis reveals transient states of dysconnectivity in schizophrenia. Neuroimage. Clinical, 5, 298–308. CrossRef Google Scholar
- Dora, E., & Kovach, A.G. (1981). Metabolic and vascular volume oscillations in the cat brain cortex. Acta Physiology of the Academy of Science of Hungary, 57(3), 261–275. Google Scholar PubMed
- Douaud, G., Jbabdi, S., Behrens, T.E., Menke, R.A., Gass, A., Monsch, A.U., & Smith, S. (2011). DTI measures in crossing-fibre areas: Increased diffusion anisotropy reveals early white matter alteration in MCI and mild Alzheimer’s disease. Neuroimage, 55(3), 880–890. CrossRef Google Scholar PubMed
- Eguiluz, V.M., Chialvo, D.R., Cecchi, G.A., Baliki, M., & Apkarian, A.V. (2005). Scale-free brain functional networks. Physical Review Letters, 94(1), 018102. CrossRef Google Scholar PubMed
- Eichele, T., Calhoun, V.D., & Debener, S. (2009). Mining EEG–fMRI using independent component analysis. International Journal of Psychophysiology, 73(1), 53–61. CrossRef Google Scholar PubMed
- Engel, A.K., Fries, P., & Singer, W. (2001). Dynamic predictions: Oscillations and synchrony in top-down processing. Nature Reviews Neuroscience, 2, 704–716. CrossRef Google Scholar PubMed
- Erhardt, E.B., Rachakonda, S., Bedrick, E.J., Allen, E.A., Adali, T., & Calhoun, V.D. (2011). Comparison of multi-subject ICA methods for analysis of fMRI data. Human Brain Mapping, 32(12), 2075–2095. CrossRef Google Scholar PubMed
- Esposito, F., Scarabino, T., Hyvarinen, A., Himberg, J., Formisano, E., Comani, S., & Salle, F. (2005). Independent component analysis of fMRI group studies by self-organizing clustering. Neuroimage, 25(1), 193–205. CrossRef Google Scholar PubMed
- Evans, A.C. (2013). Networks of anatomical covariance. Neuroimage, 80, 489–504. CrossRef Google Scholar
- Fortunato, S. (2010). Community detection in graphs. Physics Reports, 486(3-5), 75–174. CrossRef Google Scholar
- Fox, M.D., & Raichle, M.E. (2007). Spontaneous fluctuations in brain activity observed with functional magnetic resonance imaging. Nature Reviews Neuroscience, 8(9), 700–711. CrossRef Google Scholar PubMed
- Friston, K.J., Frith, C.D., Liddle, P.F., & Frackowiak, R.S. (1993). Functional connectivity: The principal-component analysis of large (PET) data sets. Journal of Cerebral Blood Flow & Metabolism, 13(1), 5–14. CrossRef Google Scholar
- Geschwind, N. (1965a). Disconnexion syndromes in animals and man. I. Brain, 88(2), 237–294. CrossRef Google Scholar
- Geschwind, N. (1965b). Disconnexion syndromes in animals and man. II. Brain, 88(3), 585–644. CrossRef Google Scholar PubMed
- Girvan, M., & Newman, M.E.J. (2002). Community structure in social and biological networks. Proceedings of the National Academy of Sciences of the United States of America, 99(12), 7821–7826. CrossRef Google Scholar PubMed
- Glover, G.H., Li, T.Q., & Ress, D. (2000). Image-based method for retrospective correction of physiological motion effects in fMRI: RETROICOR. Magnetic Resonance in Medicine, 44(1), 162–167.3.0.CO;2-E>CrossRef Google Scholar PubMed
- Golanov, E.V., Yamamoto, S., & Resi, D.J. (1994). Spontaneous waves of cerebral blood flow associated with patterns of electrocortical activity. American Journal of Physiology, 266, R204–R214. Google Scholar PubMed
- Gong, G., He, Y., Concha, L., Lebel, C., Gross, D.W., Evans, A.C., &&Beaulieu, C. (2009). Mapping anatomical connectivity patterns of human cerebral cortex using in vivo diffusion tensor imaging tractography. Cerebral Cortex, 19(3), 524–536. CrossRef Google Scholar
- Greicius, M.D., Krasnow, B., Reiss, A.L., & Menon, V. (2003). Functional connectivity in the resting brain: A network analysis of the default mode hypothesis. Proceedings of the National Academy of Sciences of the United States of America, 100(1), 253–258. CrossRef Google Scholar PubMed
- Guo, Y., & Pagnoni, G. (2008). A unified framework for group independent component analysis for multi-subject fMRI data. Neuroimage, 42(3), 1078–1093. CrossRef Google Scholar
- Gusnard, D.A., Akbudak, E., Shulman, G.L., & Raichle, M.E. (2001). Medial prefrontal cortex and self-referential mental activity: Relation to a default mode of brain function. Proceedings of the National Academy of Sciences of the United States of America, 98(7), 4259–4264. CrossRef Google Scholar
- Hagmann, P., Cammoun, L., Gigandet, X., Gerhard, S., Grant, P.E., Wedeen, V.J., & Sporns, O. (2010). MR connectomics: Principles and challenges. Journal of Neuroscience Methods, 194(1), 34–45. CrossRef Google Scholar
- Hagmann, P., Cammoun, L., Gigandet, X., Meuli, R., Honey, C.J., Wedeen, V.J., && Sporns, O. (2008). Mapping the structural core of human cerebral cortex. PLoS Biology, 6(7), e159. CrossRef Google Scholar
- Hagmann, P., Kurant, M., Gigandet, X., Thiran, P., Wedeen, V.J., Meuli, R., && Thiran, J.P. (2007). Mapping human whole-brain structural networks with diffusion MRI. PLoS One, 2(7), e597. CrossRef Google Scholar PubMed
- Halsey, J.H. Jr., & McFarland, S. (1974). Oxygen cycles and metabolic autoregulation. Stroke, 5(2), 219–225. CrossRef Google Scholar PubMed
- Harrington, D.L., Rubinov, M., Durgerian, S., Mourany, L., Reece, C., Koenig, K., & Rao, S.M. (2015). Network topology and functional connectivity disturbances precede the onset of Huntington’s disease. Brain, 138(Pt 8), 2332–2346. CrossRef Google Scholar PubMed
- He, Y., Chen, Z.J., & Evans, A.C. (2007). Small-world anatomical networks in the human brain revealed by cortical thickness from MRI. Cerebral Cortex, 17(10), 2407–2419. CrossRef Google Scholar PubMed
- Heinrichs, H.R., & Zakzanis, K.K. (1998). Neurocognitive deficit in schizophrenia: A quantitative review of the evidence. Neuropsychology, 12(3), 426–445. CrossRef Google Scholar
- Hudetz, A.G., Smith, J.J., Lee, J.G., Bosnjak, Z.J., & Kampine, J.P. (1995). Modification of cerebral laser-Doppler flow oscillations by halothane, PCO2, and nitric oxide synthase blockade. American Journal of Physiology, 269(1 Pt 2), H114–H120. Google Scholar PubMed
- Humphries, M.D., & Gurney, K. (2008). Network ‘Small-World-Ness’: A quantitative method for determining canonical network equivalence. PLoS One, 3(4), e0002051. CrossRef Google Scholar PubMed
- Hutchison, R.M., Womelsdorf, T., Allen, E.A., Bandettini, P.A., Calhoun, V.D., Corbetta, M., & Chang, C. (2013). Dynamic functional connectivity: Promise, issues, and interpretations. Neuroimage, 80, 360–378. CrossRef Google Scholar PubMed
- Hyvarinen, A., Karhunen, J., & Oja, E. (2001). Independent component analysis. New York: John Wiley & Sons. CrossRef Google Scholar PubMed
- Insel, T.R., Landis, S.C., & Collins, F.S. (2013). Research priorities. The NIH BRAIN Initiative. Science, 340(6133), 687–688. CrossRef Google Scholar PubMed
- Iturria-Medina, Y., Sotero, R.C., Canales-Rodriguez, E.J., Aleman-Gomez, Y., & Melie-Garcia, L. (2008). Studying the human brain anatomical network via diffusion-weighted MRI and Graph Theory. Neuroimage, 40(3), 1064–1076. CrossRef Google Scholar PubMed
- Jafri, M.J., Pearlson, G.D., Stevens, M., & Calhoun, V.D. (2008). A method for functional network connectivity among spatially independent resting-state components in schizophrenia. Neuroimage, 39(4), 1666–1681. CrossRef Google Scholar
- Johansen-Berg, H., & Behrens, T.E.J. (2009). Diffusion MRI: From quantitative measurement to in-vivo neuroanatomy(1st ed.). Boston: Elsevier/Academic Press. Google Scholar
- Jones, D.K. (2004). The effect of gradient sampling schemes on measures derived from diffusion tensor MRI: A Monte Carlo study. Magnetic Resonance in Medicine, 51(4), 807–815. CrossRef Google Scholar PubMed
- Jones, D.K. (2010). Diffusion MRI: Theory, methods, and applications. New York: Oxford University Press. Google Scholar
- Kalcher, K., Boubela, R.N., Huf, W., Bartova, L., Kronnerwetter, C., Derntl, B., & Moser, E. (2014). The spectral diversity of resting-state fluctuations in the human brain. PLoS One, 9(4), e93375. CrossRef Google Scholar PubMed
- Kenet, T., Bibitchkov, D., Tsodyks, M., Grinvald, A., & Arieli, A. (2003). Spontaneously emerging cortical representations of visual attributes. Nature, 425(6961), 954–956. CrossRef Google Scholar PubMed
- Kopell, N.J., Gritton, H.J., Whittington, M.A., & Kramer, M.A. (2014). Beyond the connectome: The dynome. Neuron, 83(6), 1319–1328. CrossRef Google Scholar PubMed
- Laufs, H., Krakow, K., Sterzer, P., Eger, E., Beyerle, A., Salek-Haddadi, A., && Kleinschmidt, A. (2003). Electroencephalographic signatures of attentional and cognitive default modes in spontaneous brain activity fluctuations at rest. Proceedings of the National Academy of Sciences of the United States of America, 100(19), 11053–11058. CrossRef Google Scholar PubMed
- Leonardi, N., Shirer, W.R., Greicius, l.D., & Van De Ville, D. (2014). Disentangling dynamic networks: Separated and joint expressions of functional connectivity patterns in time: Disentangling dynamic networks. Human Brain Mapping, 35(12), 5984–5995. CrossRef Google Scholar PubMed
- Leopold, D.A., Murayama, Y., & Logothetis, N.K. (2003). Very slow activity fluctuations in monkey visual cortex: Implications for functional brain imaging. Cerebral Cortex, 13(4), 422–433. CrossRef Google Scholar
- Liu, Y., Liang, M., Zhou, Y., He, Y., Hao, Y., Song, M., & Jiang, T. (2008). Disrupted small-world networks in schiz+ophrenia. Brain, 131(Pt 4), 945–961. CrossRef Google Scholar
- Logothetis, N.K. (2002). The neural basis of the blood-oxygen-level-dependent functional magnetic resonance imaging signal. Philosophical Transactions of the Royal Society B: Biological Sciences, 357(1424), 1003–1037. CrossRef Google Scholar PubMed
- Logothetis, N.K., Pauls, J., Augath, M., Trinath, T., & Oeltermann, A. (2001). Neurophysiological investigation of the basis of the fMRI signal. Nature, 412(6843), 150–157. CrossRef Google Scholar PubMed
- Lowe, M.J., Beall, E.B., Sakaie, K.E., Koenig, K.A., Stone, L., Marrie, R.A., && Phillips, M.D. (2008). Resting state sensorimotor functional connectivity in multiple sclerosis inversely correlates with transcallosal motor pathway transverse diffusivity. Human Brain Mapping, 29(7), 818–827. CrossRef Google Scholar PubMed
- Lowe, M.J., Dzemidzic, M., Lurito, J.T., Mathews, V.P., & Phillips, M.D. (2000). Correlations in low-frequency BOLD fluctuations reflect cortico-cortical connections. Neuroimage, 12(5), 582–587. CrossRef Google Scholar PubMed
- Lowe, M.J., Horenstein, C., Hirsch, J.G., Marrie, R.A., Stone, L., Bhattacharyya, P.K., et al. (2006). Functional pathway-defined MRI diffusion measures reveal increased transverse diffusivity of water in multiple sclerosis. Neuroimage, 32(3), 1127–1133. CrossRef Google Scholar PubMed
- Lowe, M.J., Koenig, K.A., Beall, E.B., Sakaie, K., Stone, L., Bermel, R., && Phillips, M.D. (2014). Anatomic connectivity assessed using pathway radial diffusivity is related to functional connectivity in monosynaptic pathways. Brain Connectivity, 4(7), 558–565. CrossRef Google Scholar PubMed
- Lowe, M.J., Mock, B.J., & Sorenson, J.A. (1998). Functional connectivity in single and multislice echoplanar imaging using resting-state fluctuations. Neuroimage, 7(2), 119–132. CrossRef Google Scholar PubMed
- Lowe, M.J., & & Russell, D.P. (1999). Treatment of baseline drifts in fMRI time series analysis. Journal of Computer Assisted Tomography, 23(3), 463–473. CrossRef Google Scholar PubMed
- Lowe, M.J., Rutecki, P., Woodard, A., Turski, P., & Sorenson, J.A. (1997). Auditory cortex fMRI noise correlations in callosal agenesis. Human Brain Mapping, 5(4), S194. Google Scholar
- Lowe, M.J., & Sorenson, J.A. (1997). Spatially filtering functional magnetic resonance imaging data. Magnetic Resonance in Medicine, 37(5), 723–729. CrossRef Google Scholar
- Makeig, S., Debener, S., Onton, J., & Delorme, A. (2004). Mining event-related brain dynamics. Trends in Cognitive Sciences, 8(5), 204–210. CrossRef Google Scholar
- McKeown, M.J., Makeig, S., Brown, G.G., Jung, T.P., Kindermann, S.S., Bell, A.J., && Sejnowski, T.J. (1998). Analysis of fMRI data by blind separation into independent spatial components. Human Brain Mapping, 6, 160–629.3.0.CO;2-1>CrossRef Google Scholar
- Mesholam-Gately, R.I., Giuliano, A.J., Goff, K.P., Faraone, S.V., & Seidman, L.J. (2009). Neurocognition in first-episode schizophrenia: A meta-analytic review. Neurophysiology, 23(3), 315–336. Google Scholar
- Meunier, D., Lambiotte, R., & Bullmore, E.T. (2010). Modular and hierarchically modular organization of brain networks. Frontiers in Neuroscience, 4, 200. CrossRef Google Scholar PubMed
- Michel, C.M., Murray, M.M., Lantz, G., Gonzalez, S., Spinelli, L., & Grave de Peralta, R. (2004). EEG source imaging. Clinical Neurophysiology, 115(10), 2195–2222. CrossRef Google Scholar PubMed
- Mori, S., Crain, B.J., Chacko, V.P., & van Zijl, P.C. (1999). Three-dimensional tracking of axonal projections in the brain by magnetic resonance imaging. Annals of Neurology, 45(2), 265–269.3.0.CO;2-3>CrossRef Google Scholar PubMed
- Moskalenko, Y.E. (1980). Biophysical aspects of cerebral circulation. Oxford: Pergamon Press. Google Scholar
- Newman, M. (2003). The structure and function of complex networks. SIAM Review, 45(2), 167–256. CrossRef Google Scholar
- Newman, M. (2010). Networks: An introduction. Oxford: Oxford University Press. CrossRef Google Scholar
- Nunez, P.L., Silberstein, R.B., Shi, Z., Carpenter, M.R., Srinivasan, R., Tucker, D.M., & Wijesinghe, R.S. (1999). EEG coherency II: Experimental comparisons of multiple measures. Clinical Neurophysiology, 110(3), 469–486. CrossRef Google Scholar
- Nunez, P.L., & Srinivasan, R. (2006). Electric fields of the brain: The neurophysics of EEG (2nd ed.). New York: Oxford University Press. CrossRef Google Scholar
- Nunez, P.L., Srinivasan, R., Westdorp, A.F., Wijesinghe, R.S., Tucker, D.M., Silberstein, R. B., && Cadusch, P. J. (1997). EEG coherency: I: Statistics, reference electrode, volume conduction, Laplacians, cortical imaging, and interpretation at multiple scales. Electroencephalography and Clinical Neurophysiology, 103(5), 499–515. CrossRef Google Scholar
- Obrig, H., Neufang, M., Wenzel, R., Kohl, M., Steinbrink, J., Einhaupl, K., et al. (2000). Spontaneous low frequency oscillations of cerebral hemodynamics and metabolism in human adults. Neuroimage, 12(6), 623–639. CrossRef Google Scholar
- Oguz, I., Farzinfar, M., Matsui, J., Budin, F., Liu, Z., Gerig, G., & Styner, M. (2014). DTIPrep: Quality control of diffusion-weighted images. Frontiers in Neuroinformatics, 8, 4. CrossRef Google Scholar PubMed
- Peltier, S.J., & Noll, D.C. (2002). T(2)(*) dependence of low frequency functional connectivity. Neuroimage, 16(4), 985–992. CrossRef Google Scholar
- Perlbarg, V., Bellec, P., Anton, J.L., Pelegrini-Issac, M., Doyon, J., & Benali, H. (2007). CORSICA: Correction of structured noise in fMRI by automatic identification of ICA components. Magnetic Resonance Imaging, 25(1), 35–46. CrossRef Google Scholar PubMed
- Pierpaoli, C., Barnett, A., Pajevic, S., Chen, R., Penix, L.R., Virta, A., && Basser, P. (2001). Water diffusion changes in Wallerian degeneration and their dependence on white matter architecture. Neuroimage, 13(6 Pt 1), 1174–1185. CrossRef Google Scholar
- Power, J.D., Mitra, A., Laumann, T.O., Snyder, A.Z., Schlaggar, B.L., & Petersen, S.E. (2012). Methods to detect, characterize, and remove motion artifact in resting state fMRI. Neuroimage, 84C, 320–341. Google Scholar
- Power, J.D., Schlaggar, B.L., & Petersen, S.E. (2015). Recent progress and outstanding issues in motion correction in resting state fMRI. Neuroimage, 105, 536–551. CrossRef Google Scholar
- Press, W., Teukolsky, S., Vetterling, W., & Flannery, B. (1993). Numerical recipes in C: The art of scientific computing. Cambridge: Cambridge University Press. Google Scholar
- Pruim, R.H., Mennes, M., Buitelaar, J.K., & Beckmann, C.F. (2015). Evaluation of ICA-AROMA and alternative strategies for motion artifact removal in resting state fMRI. Neuroimage, 112, 278–287. CrossRef Google Scholar PubMed
- Quigley, M., Cordes, D., Turski, P., Moritz, C., Haughton, V., Seth, R., &&Meyerand, M.E. (2003). Role of the corpus callosum in functional connectivity. AJNR American Journal of Neuroradiology, 24(2), 208–212. Google Scholar PubMed
- Raichle, M.E., MacLeod, A. M., Snyder, A. Z., Powers, W.J., Gusnard, D.A., & Shulman, G.L. (2001). A default mode of brain function. Proceedings of the National Academy of Sciences of the United States of America, 98(2), 676–682. CrossRef Google Scholar PubMed
- Rashid, B., Damaraju, E., Pearlson, G.D., & Calhoun, V.D. (2014). Dynamic connectivity states estimated from resting fMRI Identify differences among Schizophrenia, bipolar disorder, and healthy control subjects. Frontiers in Human Neuroscience, 8, 897. CrossRef Google Scholar PubMed
- Rubinov, M., & Bullmore, E. (2013). Fledgling pathoconnectomics of psychiatric disorders. Trends in Cognitive Sciences, 17(12), 641–647. CrossRef Google Scholar PubMed
- Rubinov, M., & Sporns, O. (2010). Complex network measures of brain connectivity: Uses and interpretations. Neuroimage, 52(3), 1059–1069. CrossRef Google Scholar PubMed
- Rubinov, M., & Sporns, O. (2011). Weight-conserving characterization of complex functional brain networks. Neuroimage, 56(4), 2068–2079. CrossRef Google Scholar PubMed
- Sakoğlu, Ü., Pearlson, G.D., Kiehl, K.A., Wang, Y.M., Michael, A.M., & Calhoun, V.D. (2010). A method for evaluating dynamic functional network connectivity and task-modulation: Application to schizophrenia. Magnetic Resonance Materials in Physics, Biology and Medicine, 23(5-6), 351–366. CrossRef Google Scholar
- Schmithorst, V.J., & Holland, S.K. (2004). Comparison of three methods for generating group statistical inferences from independent component analysis of functional magnetic resonance imaging data. Journal of Magnetic Resonance Imaging, 19(3), 365–368. CrossRef Google Scholar PubMed
- Schoffelen, J.-M., & Gross, J. (2009). Source connectivity analysis with MEG and EEG. Human Brain Mapping, 30(6), 1857–1865. CrossRef Google Scholar PubMed
- Seghier, M.L., & Friston, K.J. (2013). Network discovery with large DCMs. Neuroimage, 68(C), 181–191. CrossRef Google Scholar
- Siegel, M., Donner, T.H., & Engel, A.K. (2012). Spectral fingerprints of large-scale neuronal interactions. Nature Reviews Neuroscience, 12, 121–134. Google Scholar
- Skare, S., & Andersson, J.L. (2001). On the effects of gating in diffusion imaging of the brain using single shot EPI. Magnetic Resonance in Medicine, 19(8), 1125–1128. Google Scholar
- Smith, S.M., Fox, P.T., Miller, K.L., Glahn, D.C., Fox, P.M., Mackay, C.E., & Beckmann, C.F. (2009). Correspondence of the brain’s functional architecture during activation and rest. Proceedings of the National Academy of Sciences of the United States of America, 106(31), 13040–13045. CrossRef Google Scholar
- Smith, S.M., Jenkinson, M., Johansen-Berg, H., Rueckert, D., Nichols, T.E., Mackay, C.E., & Behrens, T.E. (2006). Tract-based spatial statistics: Voxelwise analysis of multi-subject diffusion data. Neuroimage, 31(4), 1487–1505. CrossRef Google Scholar PubMed
- Smith, S.M., Miller, K.L., Salimi-Khorshidi, G., Webster, M., Beckmann, C.F., Nichols, T.E., & Woolrich, M.W. (2011). Network modelling methods for FMRI. Neuroimage, 54(2), 875–891. CrossRef Google Scholar
- Song, S.K., Sun, S.W., Ju, W.K., Lin, S.J., Cross, A.H., & Neufeld, A.H. (2003). Diffusion tensor imaging detects and differentiates axon and myelin degeneration in mouse optic nerve after retinal ischemia. Neuroimage, 20(3), 1714–1722. CrossRef Google Scholar PubMed
- Sporns, O. (2012). Discovering the human connectome. Cambridge, MA: MIT Press. Google Scholar
- Sporns, O., Tononi, G., & Kötter, R. (2005). The human connectome: A structural description of the human brain. PLoS Computational Biology, 1(4), e42. CrossRef Google Scholar PubMed
- Srinivasan, R., Winter, W.R., Ding, J., & Nunez, P.L. (2007). EEG and MEG coherence: Measures of functional connectivity at distinct spatial scales of neocortical dynamics. Journal of Neuroscience Methods, 166(1), 41–52. CrossRef Google Scholar PubMed
- Stam, C.J. (2004). Functional connectivity patterns of human magnetoencephalographic recordings: A ‘small-world’ network? Neuroscience Letters, 355(1–2), 25–28. CrossRef Google Scholar PubMed
- Stam, C.J. (2014). Modern network science of neurological disorders. [Review]. Nature Reviews Neuroscience, 15(10), 683. CrossRef Google Scholar
- Stam, C.J., Jones, B.F., Nolte, G., Breakspear, M., & Scheltens, P. (2007). Small-world networks and functional connectivity in Alzheimer’s disease. Cerebral Cortex, 17(1), 92–99. CrossRef Google Scholar PubMed
- Stanisz, G.J., Szafer, A., Wright, G.A., & Henkelman, R.M. (1997). An analytical model of restricted diffusion in bovine optic nerve. Magnetic Resonance in Medicine, 37(1), 103–111. CrossRef Google Scholar
- Stone, J.V. (2004). Independent component analysis: A tutorial introduction. Cambridge, MA: MIT press. Google Scholar
- Strother, S.C., Anderson, J.R., Schaper, K.A., Sidtis, J.J., Liow, J.S., Woods, R.P., & Rottenberg, D.A. (1995). Principal component analysis and the scaled subprofile model compared to intersubject averaging and statistical parametric mapping: I. “Functional connectivity” of the human motor system studied with [15O]water PET. Journal of Cerebral Blood Flow & Metabolism, 15(5), 738–753. CrossRef Google Scholar
- Thirion, B., Varoquaux, G., Dohmatob, E., & Poline, J.-B. (2014). Which fMRI clustering gives good brain parcellations? [Original Research]. Frontiers in Neuroscience, 8, 167. CrossRef Google Scholar
- Tournier, J.D., Mori, S., & Leemans, A. (2011). Diffusion tensor imaging and beyond. Magnetic Resonance in Medicine, 65(6), 1532–1556. CrossRef Google Scholar PubMed
- van den Heuvel, M.P., & Sporns, O. (2011). Rich-club organization of the human connectome. The Journal of Neuroscience, 31(44), 15775–15786. CrossRef Google Scholar PubMed
- van den Heuvel, M.P., & Sporns, O. (2013). Network hubs in the human brain. Trends in Cognitive Sciences, 17(12), 683–696. CrossRef Google Scholar PubMed
- Van Dijk, K.R., Hedden, T., Venkataraman, A., Evans, K.C., Lazar, S.W., & Buckner, R.L. (2010). Intrinsic functional connectivity as a tool for human connectomics: Theory, properties, and optimization. Journal of Neurophysiology, 103, 297–321. CrossRef Google Scholar
- Varela, F., Lachaux, J.P., Rodriguez, E., & Martinerie, J. (2001). The brainweb: Phase synchronization and large-scale integration. Nature Reviews. Neuroscience, 2(4), 229–239. CrossRef Google Scholar PubMed
- Vern, B.A., Schuette, W.H., Leheta, B., Juel, V.C., & Radulovacki, M. (1988). Low-frequency oscillations of cortical oxidative metabolism in waking and sleep. Journal of Cerebral Blood Flow & Metabolism, 8(2), 215–226. CrossRef Google Scholar PubMed
- Wang, Y., Wang, Q., Haldar, J.P., Yeh, F.C., Xie, M., Sun, P., & Song, S.K. (2011). Quantification of increased cellularity during inflammatory demyelination. Brain, 134(Pt 12), 3590–3601. CrossRef Google Scholar PubMed
- Watts, D.J., & Strogatz, S.H. (1998). Collective dynamics of /“small-world/” networks. Nature, 393(6684), 440–442. CrossRef Google Scholar PubMed
- Wheeler-Kingshott, C.A., & Cercignani, M. (2009). About “axial” and “radial” diffusivities. Magnetic Resonance in Medicine, 61(5), 1255–1260. CrossRef Google Scholar PubMed
- Yaesoubi, M., Miller, R.L., & Calhoun, V.D. (2015). Mutually temporally independent connectivity patterns: A new framework to study the dynamics of brain connectivity at rest with application to explain group difference based on gender. Neuroimage, 107, 85–94. CrossRef Google Scholar PubMed
- Yang, H., Long, X.Y., Yang, Y., Yan, H., Zhu, C.Z., Zhou, X.P., & Gong, Q, Y. (2007). Amplitude of low frequency fluctuation within visual areas revealed by resting-state functional MRI. Neuroimage, 36(1), 144–152. CrossRef Google Scholar PubMed
- Yendiki, A., Koldewyn, K., Kakunoori, S., Kanwisher, N., & Fischl, B. (2013). Spurious group differences due to head motion in a diffusion MRI study. Neuroimage, 88C, 79–90. Google Scholar
- Yendiki, A., Panneck, P., Srinivasan, P., Stevens, A., Zollei, L., Augustinack, J., & Fischl, B. (2011). Automated probabilistic reconstruction of white-matter pathways in health and disease using an atlas of the underlying anatomy. Frontiers in Neuroinformatics, 5, 23. CrossRef Google Scholar
- Zang, Y., Jiang, T., Lu, Y., He, Y., & Tian, L. (2004). Regional homogeneity approach to fMRI data analysis. Neuroimage, 22(1), 394–400. CrossRef Google Scholar PubMed
Recent advances in neuroimaging methodologies sensitive to axonal injury have made it possible to assess in vivo the extent of traumatic brain injury (TBI) -related disruption in neural structures and their connections. The objective of this paper is to review studies examining connectivity in TBI with an emphasis on structural and functional MRI methods that have proven to be valuable in uncovering neural abnormalities associated with this condition.
We review studies that have examined white matter integrity in TBI of varying etiology and levels of severity, and consider how findings at different times post-injury may inform underlying mechanisms of post-injury progression and recovery. Moreover, in light of recent advances in neuroimaging methods to study the functional connectivity among brain regions that form integrated networks, we review TBI studies that use resting-state functional connectivity MRI methodology to examine neural networks disrupted by putative axonal injury.
The findings suggest that TBI is associated with altered structural and functional connectivity, characterized by decreased integrity of white matter pathways and imbalance and inefficiency of functional networks. These structural and functional alterations are often associated with neurocognitive dysfunction and poor functional outcomes.
TBI has a negative impact on distributed brain networks that lead to behavioral disturbance. (JINS, 2016,22, 120–137)
- Adams, J.H., Doyle, D., Ford, I., Gennarelli, T.A., Graham, D.I., & McLellan, D.R. (1989). Diffuse axonal injury in head injury: Definition, diagnosis and grading. Histopathology, 15, 49–59. CrossRef Google Scholar PubMed
- Aoki, Y., Inokuchi, R., Gunshin, M., Yahagi, N., & Suwa, H. (2012). Diffusion tensor imaging studies of mild traumatic brain injury: A meta-analysis. Journal of Neurology, Neurosurgery, & Psychiatry, 83, 870–876. CrossRef Google Scholar PubMed
- Arenivas, A., Diaz-Arrastia, R., Spence, J., Cullum, C., Krishnan, K., Bosworth, C., & Marquez de la Plata, C. (2014). Three approaches to investigating functional compromise to the default mode network after traumatic axonal injury. Brain Imaging and Behavior, 8, 407–419. CrossRef Google Scholar PubMed
- Arfanakis, K., Haughton, V.M., Carew, J.D., Rogers, B.P., Dempsey, R.J., & Meyerand, M.E. (2002). Diffusion tensor MR imaging in diffuse axonal injury. AJNR: American Journal of Neuroradiology, 23, 794–802. Google Scholar
- Arnemann, K.L., Chen, A.J.W., Novakovic-Agopian, T., Gratton, C., Nomura, E.M., & D’Esposito, M. (2015). Functional brain network modularity predicts response to cognitive training after brain injury. Neurology, 84, 1568–1574. CrossRef Google Scholar PubMed
- Ashwal, S., Tong, K.A., Ghosh, N., Bartnik-Olson, B., & Holshouser, B.A. (2014). Application of advanced neuroimaging modalities in pediatric traumatic brain injury. Journal of Child Neurology, 29, 1704–1717. CrossRef Google Scholar PubMed
- Bazarian, J.J., Donnelly, K., Peterson, D.R., Warner, G.C., Zhu, T., & Zhong, J. (2013). The relation between posttraumatic stress disorder and mild traumatic brain injury acquired during Operations Enduring Freedom and Iraqi Freedom. Journal of Head Trauma Rehabilitation, 28, 1–12. CrossRef Google Scholar
- Bazarian, J.J., Zhong, J., Blyth, B., Zhu, T., Kavcic, V., & Peterson, D. (2007). Diffusion tensor imaging detects clinically important axonal damage after mild traumatic brain injury: A pilot study. Journal of Neurotrauma, 24, 1447–1459. CrossRef Google Scholar PubMed
- Bazarian, J.J., Zhu, T., Blyth, B., Borrino, A., & Zhong, J. (2012). Subject-specific changes in brain white matter on diffusion tensor imaging after sports-related concussion. Magnetic Resonance Imaging, 30, 171–180. CrossRef Google Scholar PubMed
- Begonia, M., Prabhu, R., Liao, J., Whittington, W., Claude, A., Willeford, B., Wardlaw, J., &... Williams, L. (2014). Quantitative analysis of brain microstructure following mild blunt and blast trauma. Journal of Biomechanics, 47(15), 3704–3711. CrossRef Google Scholar PubMed
- Bendlin, B.B., Ries, M.L., Lazar, M., Alexander, A.L., Dempsey, R.J., Rowley, H.A., & Johnson, S.C. (2008). Longitudinal changes in patients with traumatic brain injury assessed with diffusion-tensor and volumetric imaging. Neuroimage, 42, 503–514. CrossRef Google Scholar PubMed
- Bigler, E.D. (2001). The lesion (s) in traumatic brain injury: Implications for clinical neuropsychology. Archives of Clinical Neuropsychology, 16, 95–131. CrossRef Google Scholar PubMed
- Bigler, E.D. (2013a). Neuroinflammation and the dynamic lesion in traumatic brain injury. Brain, 136, 9–11. CrossRef Google Scholar
- Bigler, E.D. (2013b). Traumatic brain injury, neuroimaging, and neurodegeneration. Frontiers in Human Neuroscience, 7, 395. CrossRef Google Scholar PubMed
- Bigler, E.D., Abildskov, T.J., Wilde, E.A., McCauley, S.R., Li, X., Merkley, T.L., & Levin, H.S. (2010). Diffuse damage in pediatric traumatic brain injury: A comparison of automated versus operator-controlled quantification methods. Neuroimage, 50, 1017–1026. CrossRef Google Scholar PubMed
- Bigler, E.D., & Maxwell, W.L. (2012). Neuropathology of mild traumatic brain injury: Relationship to neuroimaging findings. Brain Imaging and Behavior, 6, 108–136. CrossRef Google Scholar PubMed
- Blumbergs, P.C., Scott, G., Vis, J.M., Wainwright, H., Simpson, D.A., & McLean, A.J. (1995). Topography of axonal injury as defined by amyloid precursor protein and the sector scoring method in mild and severe closed head injury. Journal of Neurotrauma, 12(4), 565–572. CrossRef Google Scholar PubMed
- Bonelle, V., Ham, T., Leech, R., Kinnunen, K., Mehta, M., Greenwood, R., & Sharp, D. (2012). Salience network integrity predicts default mode network function after traumatic brain injury. Proceedings of the National Academy of Sciences of the United States of America, 109, 4690–4695. CrossRef Google Scholar
- Bonelle, V., Leech, R., MKinnunen, K., Ham, T., Beckmann, C., De Boissezon, X., & Sharp, D. (2011). Default mode network connectivity predicts sustained attention deficits after traumatic brain injury. The Journal of Neuroscience, 31, 13442–13451. CrossRef Google Scholar
- Bouix, S., Pasternak, O., Rathi, Y., Pelavin, P.E., Zafonte, R., & Shenton, M.E. (2013). Increased gray matter diffusion anisotropy in patients with persistent post-concussive symptoms following mild traumatic brain injury. PLoS One, 8(6), e66205. CrossRef Google Scholar PubMed
- Bramlett, H.M., & Dietrich, W.D. (in press). Long-term consequences of traumatic brain injury: Current status of potential mechanisms of injury and neurological outcomes. Journal of Neurotrauma. doi: 10.1089/neu.2014.3352. Google Scholar PubMed
- Caeyenberghs, K., Leemans, A., Geurts, M., Taymans, T., Vander Linden, C., Smits-Engelsman, B.C.M., & Swinnen, S.P. (2010). Brain-behavior relationships in young traumatic brain injury patients: Fractional anisotropy measures are highly correlated with dynamic visuomotor tracking performance. Neuropsychologia, 48(5), 1472–1482. CrossRef Google Scholar PubMed
- Caeyenberghs, K., Leemans, A., Heitger, M.H., Leunissen, I., Dhollander, T., Sunaert, S., & Swinnen, S.P. (2012). Graph analysis of functional brain networks for cognitive control of action in traumatic brain injury. Brain, 135(4), 1293–1307. CrossRef Google Scholar PubMed
- Catani, M. (2005). The rises and falls of disconnection syndromes. Brain, 128(10), 2224–2239. CrossRef Google Scholar PubMed
- Catani, M., Dell’Acqua, F., & Thiebaut de Schotten, M. (2013). A revised limbic system model for memory, emotion and behaviour. Neuroscience & Biobehavioral Reviews, 37, 1724–1737. CrossRef Google Scholar PubMed
- Catani, M., & Thiebaut de Schotten, M. (2008). A diffusion tensor imaging tractography atlas for virtual in vivo dissections. Cortex, 44, 1105–1132. CrossRef Google Scholar PubMed
- Choudhri, A.F., Chin, E.M., Blitz, A.M., & Gandhi, D. (2014). Diffusion tensor imaging of cerebral white matter. Radiologic Clinics of North America, 52, 413–425. CrossRef Google Scholar PubMed
- Cole, D.M., Smith, S.M., & Beckmann, C.F. (2010). Advances and pitfalls in the analysis and interpretation of resting-state FMRI data. Frontiers in Systems Neuroscience, 4, 8. Google Scholar PubMed
- Corbetta, M. (2012). Functional connectivity and neurological recovery. Developmental Psychobiology, 54, 239–253. CrossRef Google Scholar
- Costanzo, M.E., Chou, Y.-Y., Leaman, S., Pham, D.L., Keyser, D., Nathan, D.E., & Roy, M.J. (2014). Connecting combat-related mild traumatic brain injury with posttraumatic stress disorder symptoms through brain imaging. Neuroscience Letters, 577, 11–15. CrossRef Google Scholar PubMed
- Davenport, N.D., Lim, K.O., Armstrong, M.T., & Sponheim, S.R. (2012). Diffuse and spatially variable white matter disruptions are associated with blast-related mild traumatic brain injury. Neuroimage, 59, 2017–2024. CrossRef Google Scholar PubMed
- Davenport, N.D., Lim, K.O., & Sponheim, S.R. (2015). White matter abnormalities associated with military PTSD in the context of blast TBI. Human Brain Mapping, 36(3), 1053–1064. CrossRef Google Scholar PubMed
- Fagerholm, E.D., Hellyer, P.J., Scott, G., Leech, R., & Sharp, D.J. (2015). Disconnection of network hubs and cognitive impairment after traumatic brain injury. Brain, 138(6), 1696–1709. CrossRef Google Scholar PubMed
- Fakhran, S., Yaeger, K., & Alhilali, L. (2013). Symptomatic white matter changes in mild traumatic brain injury resemble pathologic features of early Alzheimer dementia. Radiology, 269, 249–257. CrossRef Google Scholar PubMed
- Farbota, K.D., Bendlin, B.B., Alexander, A.L., Rowley, H.A., Dempsey, R.J., & Johnson, S.C. (2012). Longitudinal diffusion tensor imaging and neuropsychological correlates in traumatic brain injury patients. Frontiers in Human Neuroscience, 6, 160. CrossRef Google Scholar PubMed
- Gale, S.D., Johnson, S.C., Bigler, E.D., & Blatter, D.D. (1995). Nonspecific white matter degeneration following traumatic brain injury. Journal of the International Neuropsychological Society, 1(1), 17–28. CrossRef Google Scholar PubMed
- Gardner, A., Kay-Lambkin, F., Stanwell, P., Donnelly, J., Williams, W.H., Hiles, A., & Jones, D.K. (2012). A systematic review of diffusion tensor imaging findings in sports-related concussion. Journal of Neurotrauma, 29, 2521–2538. CrossRef Google Scholar PubMed
- Geary, E.K., Kraus, M.F., Pliskin, N.H., & Little, D.M. (2010). Verbal learning differences in chronic mild traumatic brain injury. Journal of the International Neuropsychological Society, 16, 506. CrossRef Google Scholar
- Gentry, L.R., Thompson, B., & Godersky, J.C. (1988). Trauma to the corpus callosum: MR features. AJNR: American Journal of Neuroradiology, 9(6), 1129–1138. Google Scholar PubMed
- Geschwind, N. (1965). Disconnexion syndromes in animals and man. Brain, 88(3), 585–585. CrossRef Google Scholar PubMed
- Goetz, P., Blamire, A., Rajagopalan, B., Cadoux-Hudson, T., Young, D., & Styles, P. (2004). Increase in apparent diffusion coefficient in normal appearing white matter following human traumatic brain injury correlates with injury severity. Journal of Neurotrauma, 21, 645–654. CrossRef Google Scholar PubMed
- Greenberg, G., Mikulis, D.J., Ng, K., DeSouza, D., & Green, R.E. (2008). Use of diffusion tensor imaging to examine subacute white matter injury progression in moderate to severe traumatic brain injury. Archives of Physical Medicine and Rehabilitation, 89, S45–S50. CrossRef Google Scholar PubMed
- Grossman, E.J., Jensen, J.H., Babb, J.S., Chen, Q., Tabesh, A., Fieremans, E., & Grossman, R.I. (2013). Cognitive impairment in mild traumatic brain injury: A longitudinal diffusional kurtosis and perfusion imaging study. AJNR: American Journal of Neuroradiology, 34, 951–957. CrossRef Google Scholar PubMed
- Håberg, A., Olsen, A., Moen, K., Schirmer-Mikalsen, K., Visser, E., Finnanger, T., & Eikenes, L. (2015). White matter microstructure in chronic moderate-to-severe traumatic brain injury: Impact of acute-phase injury-related variables and associations with outcome measures. Journal of Neuroscience Research, 93, 1109–1126. CrossRef Google Scholar
- Ham, T.E., Bonnelle, V., Hellyer, P., Jilka, S., Robertson, I.H., Leech, R., & Sharp, D.J. (2014). The neural basis of impaired self-awareness after traumatic brain injury. Brain, 137(2), 586–597. CrossRef Google Scholar PubMed
- Han, K., Mac Donald, C., Johnson, A., Barnes, Y., Wierzechowski, L., Zonies, D., & Brody, D. (2014). Disrupted modular organization of resting-state cortical functional connectivity in U.S. military personnel following concussive ‘mild’ blast-related traumatic brain injury. Neuroimage, 84, 76–96. CrossRef Google Scholar PubMed
- Hartikainen, K.M., Wäljas, M., Isoviita, T., Dastidar, P., Liimatainen, S., Solbakk, A.-K., & Öhman, J. (2010). Persistent symptoms in mild to moderate traumatic brain injury associated with executive dysfunction. Journal of Clinical and Experimental Neuropsychology, 32, 767–774. CrossRef Google Scholar PubMed
- Hayes, J.P., Miller, D.R., Lafleche, G., Salat, D.H., & Verfaellie, M. (2015). The nature of white matter abnormalities in blast-related mild traumatic brain injury. Neuroimage: Clinical, 8, 148–156. CrossRef Google Scholar PubMed
- Hayes, J.P., Morey, R.A., & Tupler, L.A. (2012). A case of frontal neuropsychological and neuroimaging signs following multiple primary-blast exposure. Neurocase, 18, 258–269. CrossRef Google Scholar PubMed
- Hellyer, P.J., Leech, R., Ham, T.E., Bonnelle, V., & Sharp, D.J. (2013). Individual prediction of white matter injury following traumatic brain injury: Multivariate analysis of white matter after TBI. Annals of Neurology, 73, 489–499. CrossRef Google Scholar
- Hellyer, P.J., Scott, G., Shanahan, M., Sharp, D.J., & Leech, R. (2015). Cognitive flexibility through metastable neural dynamics is disrupted by damage to the structural connectome. The Journal of Neuroscience, 35(24), 9050–9063. CrossRef Google Scholar PubMed
- Henry, L.C., Tremblay, J., Tremblay, S., Lee, A., Brun, C., Lepore, N., & Lassonde, M. (2011). Acute and chronic changes in diffusivity measures after sports concussion. Journal of Neurotrauma, 28, 2049–2059. CrossRef Google Scholar PubMed
- Hillary, F., Rajtmajer, S., Roman, C., Medaglia, J., Slocomb-Dluzen, J., Calhoun, V., & Wylie, G. (2014). The rich get richer: Brain injury elicits hyperconnectivity in core subnetworks. PLoS One, 9, 1–15. CrossRef Google Scholar PubMed
- Hillary, F., Roman, C., Venkatesan, U., & Rajtmajer, S. (2015). Hyperconnectivity is a fundamental response to neurological disruption. Neuropsychology, 29, 59–75. CrossRef Google Scholar PubMed
- Hillary, F., Slocomb, J., Hills, E., Fitzpatrick, N., Medaglia, J., Wang, J., & Wylie, G. (2011). Changes in resting connectivity during recovery from severe traumatic brain injury. International Journal of Psychophysiology, 82, 115–123. CrossRef Google Scholar PubMed
- Hou, D.J., Tong, K.A., Ashwal, S., Oyoyo, U., Joo, E., Shutter, L. &Obenaus, A. (2007). Diffusion-weighted magnetic resonance imaging improves outcome prediction in adult traumatic brain injury. Journal of Neurotrauma, 24, 1558–1569. CrossRef Google Scholar PubMed
- Huisman, T.A., Schwamm, L.H., Schaefer, P.W., Koroshetz, W.J., Shetty-Alva, N., Ozsunar, Y., & Sorensen, A.G. (2004). Diffusion tensor imaging as potential biomarker of white matter injury in diffuse axonal injury. AJNR: American Journal of Neuroradiology, 25(3), 370–376. Google Scholar PubMed
- Hulkower, M.B., Poliak, D.B., Rosenbaum, S.B., Zimmerman, M.E., & Lipton, M.L. (2013). A decade of DTI in traumatic brain injury: 10 years and 100 articles later. AJNR: American Journal of Neuroradiology, 34, 2064–2074. CrossRef Google Scholar PubMed
- Ilvesmaki, T., Luoto, T.M., Hakulinen, U., Brander, A., Ryymin, P., Eskola, H., & Ohman, J. (2014). Acute mild traumatic brain injury is not associated with white matter change on diffusion tensor imaging. Brain, 137, 1876–1882. CrossRef Google Scholar
- Inglese, M., Makani, S., Johnson, G., Cohen, B.A., Silver, J.A., Gonen, O., & Grossman, R.I. (2005). Diffuse axonal injury in mild traumatic brain injury: A diffusion tensor imaging study. Journal of Neurosurgery, 103, 298–303. CrossRef Google Scholar PubMed
- Irimia, A., Chambers, M.C., Torgerson, C.M., Filippou, M., Hovda, D.A., Alger, J.R., & Kikinis, R. (2012). Patient-tailored connectomics visualization for the assessment of white matter atrophy in traumatic brain injury. Frontiers in Neurology, 3, 10. CrossRef Google Scholar PubMed
- Irimia, A., Goh, S.-Y., Torgerson, C., Vespa, P., & Van Horn, J. (2014). Structural and connectomic neuroimaging for the personalized study of longitudinal alterations in cortical shape, thickness and connectivity after traumatic brain injury. Journal of Neurosurgical Sciences, 58(3), 129. Google Scholar PubMed
- Jilka, S., Scott, G., Ham, T., Pickering, A., Bonelle, V., Braga, R., & Sharp, D. (2014). Damage to the salience network and interactions with the default mode network. The Journal of Neuroscience, 34, 10798–10807. CrossRef Google Scholar PubMed
- Johnson, B., Zhang, K., Gay, M., Horovitz, S., Hallett, M., Sebastianelli, W., & Slobounov, S. (2012). Alteration of brain default network in subacute phase of injury in concussed individuals: Resting-state fMRI study. Neuroimage, 59, 511–518. CrossRef Google Scholar PubMed
- Johnson, V.E., Stewart, J.E., Begbie, F.D., Trojanowski, J.Q., Smith, D.H., & Stewart, W. (2013). Inflammation and white matter degeneration persist for years after a single traumatic brain injury. Brain, 136, 28–42. CrossRef Google Scholar PubMed
- Jones, D. (2008). Studying connections in the living human brain with diffusion MRI. Cortex, 44, 936–952. CrossRef Google Scholar PubMed
- Jones, D.K., & Cercignani, M. (2010). Twenty‐five pitfalls in the analysis of diffusion MRI data. NMR in Biomedicine, 23(7), 803–820. CrossRef Google Scholar PubMed
- Jorge, R.E., Acion, L., White, T., Tordesillas-Gutierrez, D., Pierson, R., Crespo-Facorro, B., & Magnotta, V.A. (2012). White matter abnormalities in veterans with mild traumatic brain injury. American Journal of Psychiatry, 169, 1284–1291. CrossRef Google Scholar PubMed
- Kennedy, M.R.T., Wozniak, J.R., Muetzel, R.L., Mueller, B.A., Chiou, H.-H., Pantekoek, K., & Lim, K.O. (2009). White matter and neurocognitive changes in adults with chronic traumatic brain injury. Journal of the International Neuropsychological Society, 15, 130–136. CrossRef Google Scholar PubMed
- Kim, N., Branch, C.A., Kim, M., & Lipton, M.L. (2013). Whole brain approaches for identification of microstructural abnormalities in individual patients: Comparison of techniques applied to mild traumatic brain injury. PLoS One, 8, e59382. CrossRef Google Scholar PubMed
- Kinnunen, K.M., Greenwood, R., Powell, J.H., Leech, R., Hawkins, P.C., Bonnelle, V., & Sharp, D.J. (2011). White matter damage and cognitive impairment after traumatic brain injury. Brain, 134, 449–463. CrossRef Google Scholar PubMed
- Koerte, I.K., Ertl-Wagner, B., Reiser, M., Zafonte, R., & Shenton, M.E. (2012). White matter integrity in the brains of professional soccer players without a symptomatic concussion. Journal of the American Medical Association, 308(18), 1859–1861. CrossRef Google Scholar PubMed
- Koerte, I.K., Kaufmann, D., Hartl, E., Bouix, S., Pasternak, O., Kubicki, M., & Shenton, M.E. (2012). A prospective study of physician-observed concussion during a varsity university hockey season: White matter integrity in ice hockey players. Part 3 of 4. Neurosurgical Focus, 33, E3. CrossRef Google Scholar PubMed
- Kraus, M.F., Susmaras, T., Caughlin, B.P., Walker, C.J., Sweeney, J.A., & Little, D.M. (2007). White matter integrity and cognition in chronic traumatic brain injury: A diffusion tensor imaging study. Brain, 130, 2508–2519. CrossRef Google Scholar PubMed
- Kumar, R., Saksena, S., Husain, M., Srivastava, A., Rathore, R.K., Agarwal, S., & Gupta, R.K. (2010). Serial changes in diffusion tensor imaging metrics of corpus callosum in moderate traumatic brain injury patients and their correlation with neuropsychometric tests: A 2-year follow-up study. Journal of Head Trauma Rehabilitation, 25, 31–42. CrossRef Google Scholar PubMed
- Lange, R.T., Iverson, G.L., Brubacher, J.R., Mädler, B., & Heran, M.K. (2012). Diffusion tensor imaging findings are not strongly associated with postconcussional disorder 2 months following mild traumatic brain injury. Journal of Head Trauma Rehabilitation, 27(3), 188–198. CrossRef Google Scholar
- Lange, R.T., Panenka, W.J., Shewchuk, J.R., Heran, M.K.S., Brubacher, J.R., Bioux, S., & Iverson, G.L. (2015). Diffusion tensor imaging findings and postconcussion symptom reporting six weeks following mild traumatic brain injury. Archives of Clinical Neuropsychology, 30(1), 7–25. CrossRef Google Scholar PubMed
- Levin, H.S., Wilde, E., Troyanskaya, M., Petersen, N.J., Scheibel, R., Newsome, M., & Li, X. (2010). Diffusion tensor imaging of mild to moderate blast-related traumatic brain injury and its sequelae. Journal of Neurotrauma, 27, 683–694. CrossRef Google Scholar PubMed
- Ling, J.M., Pena, A., Yeo, R.A., Merideth, F.L., Klimaj, S., Gasparovic, C., & Mayer, A.R. (2012). Biomarkers of increased diffusion anisotropy in semi-acute mild traumatic brain injury: A longitudinal perspective. Brain, 135, 1281–1292. CrossRef Google Scholar PubMed
- Lipton, M.L., Gellella, E., Lo, C., Gold, T., Ardekani, B.A., Shifteh, K., & Branch, C.A. (2008). Multifocal white matter ultrastructural abnormalities in mild traumatic brain injury with cognitive disability: A voxel-wise analysis of diffusion tensor imaging. Journal of Neurotrauma, 25, 1335–1342. CrossRef Google Scholar PubMed
- Lipton, M.L., Gulko, E., Zimmerman, M.E., Friedman, B.W., Kim, M., Gellella, E., & Branch, C.A. (2009). Diffusion-tensor imaging implicates prefrontal axonal injury in executive function impairment following very mild traumatic brain injury. Radiology, 252, 816–824. CrossRef Google Scholar PubMed
- Lipton, M.L., Kim, N., Park, Y.K., Hulkower, M.B., Gardin, T.M., Shifteh, K., & Branch, C.A. (2012). Robust detection of traumatic axonal injury in individual mild traumatic brain injury patients: Intersubject variation, change over time and bidirectional changes in anisotropy. Brain Imaging and Behavior, 6, 329–342. CrossRef Google Scholar PubMed
- Little, D.M., Kraus, M.F., Joseph, J., Geary, E.K., Susmaras, T., Zhou, X.J., Pliskin, N., & Gorelick, P.B. (2010). Thalamic integrity underlies executive dysfunction in traumatic brain injury. Neurology, 74, 558–564. CrossRef Google Scholar PubMed
- Ljungqvist, J., Nilsson, D., Ljungberg, M., Sörbo, A., Esbjörnsson, E., Eriksson-Ritzén, C., & Skoglund, T. (2011). Longitudinal study of the diffusion tensor imaging properties of the corpus callosum in acute and chronic diffuse axonal injury. Brain Injury, 25, 370–378. CrossRef Google Scholar PubMed
- Lo, C., Shifteh, K., Gold, T., Bello, J.A., & Lipton, M.L. (2009). Diffusion tensor imaging abnormalities in patients with mild traumatic brain injury and neurocognitive impairment. Journal of Computer Assisted Tomography, 33(2), 293–297. CrossRef Google Scholar
- Mac Donald, C.L., Adam, O.R., Johnson, A.M., Nelson, E.C., Werner, N.J., Rivet, D.J., & Brody, D.L. (2015). Acute post-traumatic stress symptoms and age predict outcome in military blast concussion. Brain, 138(5), 1314–1326. CrossRef Google Scholar
- Mac Donald, C.L., Dikranian, K., Song, S.K., Bayly, P.V., Holtzman, D.M., & Brody, D.L. (2007). Detection of traumatic axonal injury with diffusion tensor imaging in a mouse model of traumatic brain injury. Experimental Neurology, 205(1), 116–131. CrossRef Google Scholar
- Mac Donald, C.L., Johnson, A.M., Cooper, D., Nelson, E.C., Werner, N.J., Shimony, J.S., & Brody, D.L. (2011). Detection of blast–related traumatic brain injury in U.S. military personnel. New England Journal of Medicine, 364, 2091–2100. CrossRef Google Scholar PubMed
- Marmarou, A., Signoretti, S., Fatouros, P.P., Portella, G., Aygok, G.A., & Bullock, M.R. (2006). Predominance of cellular edema in traumatic brain swelling in patients with severe head injuries. Journal of Neurosurgery, 104, 720–730. CrossRef Google Scholar PubMed
- Marquez de la Plata, C., Garces, J., Kojori, E., Grinnan, J., Krishnan, K., Pidikiti, R., & Diaz-Arrastia, R. (2011). Deficits in functional connectivity of hippocampal and frontal lobe circuits after traumatic axonal injury. Archives of Neurology, 68, 74–84. CrossRef Google Scholar PubMed
- Matsukawa, H., Shinoda, M., Fujii, M., Takahashi, O., Yamamoto, D., Murakata, A., & Ishikawa, R. (2011). Genu of corpus callosum in diffuse axonal injury induces a worse 1-year outcome in patients with traumatic brain injury. Acta Neurochirurgica, 153(8), 1687–1694. CrossRef Google Scholar PubMed
- Matthews, S.C., Strigo, I.A., Simmons, A.N., O’Connell, R.M., Reinhardt, L.E., & Moseley, S.A. (2011). A multimodal imaging study in US veterans of Operations Iraqi and Enduring Freedom with and without major depression after blast-related concussion. Neuroimage, 54, S69–S75. CrossRef Google Scholar
- Mayer, A.R., Ling, J., Mannell, M.V., Gasparovic, C., Phillips, J.P., Doezema, D., & Yeo, R.A. (2010). A prospective diffusion tensor imaging study in mild traumatic brain injury. Neurology, 74, 643–650. CrossRef Google Scholar
- Mayer, A.R., Mannell, M., Ling, J., Gasparovic, C., & Yeo, R. (2011). Functional connectivity in mild traumatic brain injury. Human Brain Mapping, 32, 1825–1835. CrossRef Google Scholar PubMed
- McCrea, M., Iverson, G.L., McAllister, T.W., Hammeke, T.A., Powell, M.R., Barr, W.B., & Kelly, J.P. (2009). An integrated review of recovery after mild traumatic brain injury (MTBI): Implications for clinical management. The Clinical Neuropsychologist, 23(8), 1368–1390. CrossRef Google Scholar PubMed
- McKee, A.C., Stein, T.D., Nowinski, C.J., Stern, R.A., Daneshvar, D.H., Alvarez, V.E., & Baugh, C.M. (2013). The spectrum of disease in chronic traumatic encephalopathy. Brain, 136(1), 43–64. CrossRef Google Scholar PubMed
- Messé, A., Caplain, S., Paradot, G., Garrigue, D., Mineo, J.-F., Soto Ares, G., & Lehéricy, S. (2011). Diffusion tensor imaging and white matter lesions at the subacute stage in mild traumatic brain injury with persistent neurobehavioral impairment. Human Brain Mapping, 32, 999–1011. CrossRef Google Scholar PubMed
- Messé, A., Caplain, S., Pélégrini-Issac, M., Blancho, S., Lévy, R., Aghakhani, N., & Lehéricy, S. (2013). Specific and evolving resting-state network alterations in post-concussion syndrome following mild traumatic brain injury. PLoS One, 8, 1–10. CrossRef Google Scholar PubMed
- Messé, A., Caplain, S., Pélégrini-Issac, M., Blancho, S., Montreuil, M., Lévy, R., & Benali, H. (2012). Structural integrity and postconcussion syndrome in mild traumatic brain injury patients. Brain Imaging and Behavior, 6, 283–292. CrossRef Google Scholar PubMed
- Meythaler, J.M., Peduzzi, J.D., Eleftheriou, E., & Novack, T.A. (2001). Current concepts: Diffuse axonal injury–associated traumatic brain injury. Archives of Physical Medicine and Rehabilitation, 82, 1461–1471. CrossRef Google Scholar PubMed
- Miles, L., Grossman, R.I., Johnson, G., Babb, J.S., Diller, L., & Inglese, M. (2008). Short-term DTI predictors of cognitive dysfunction in mild traumatic brain injury. Brain Injury, 22(2), 115–122. CrossRef Google Scholar PubMed
- Miller, B.T., & D’Esposito, M. (2005). Searching for “the top” in top-down control. Neuron, 48(4), 535–538. CrossRef Google Scholar
- Moen, K.G., Håberg, A.K., Skandsen, T., Finnanger, T.G., & Vik, A. (2014). A longitudinal magnetic resonance imaging study of the apparent diffusion coefficient values in corpus callosum during the first year after traumatic brain injury. Journal of Neurotrauma, 31, 56–63. CrossRef Google Scholar PubMed
- Morey, R.A., Haswell, C.C., Selgrade, E.S., Massoglia, D., Liu, C., Weiner, J., & McCarthy, G. (2013). Effects of chronic mild traumatic brain injury on white matter integrity in Iraq and Afghanistan war veterans: Effects of TBI on white matter integrity. Human Brain Mapping, 34, 2986–2999. CrossRef Google Scholar PubMed
- Nakamura, T., Hillary, F., & Biswal, B. (2009). Resting network plasticity following brain injury. PLoS One, 4, 1–9. CrossRef Google Scholar PubMed
- Nakayama, N. (2006). Evidence for white matter disruption in traumatic brain injury without macroscopic lesions. Journal of Neurology, Neurosurgery, & Psychiatry, 77, 850–855. CrossRef Google Scholar PubMed
- Newcombe, V.F., Correia, M.M., Ledig, C., Abate, M.G., Outtrim, J.G., Chatfield, D., & Pickard, J.D. (2015). Dynamic changes in white matter abnormalities correlate with late improvement and deterioration following TBI: A diffusion tensor imaging study. Neurorehabilitation and Neural Repair. doi: 10.1177/1545968315584004. Google Scholar PubMed
- Newcombe, V.F.J., Williams, G.B., Nortje, J., Bradley, P.G., Harding, S.G., Smielewski, P., & Menon, D.K. (2007). Analysis of acute traumatic axonal injury using diffusion tensor imaging. British Journal of Neurosurgery, 21, 340–348. CrossRef Google Scholar PubMed
- Niogi, S.N., Mukherjee, P., Ghajar, J., Johnson, C.E., Kolster, R., Lee, H., & McCandliss, B.D. (2008). Structural dissociation of attentional control and memory in adults with and without mild traumatic brain injury. Brain, 131, 3209–3221. CrossRef Google Scholar PubMed
- Ommaya, A.K., & Gennarelli, T.A. (1974). Cerebral concussion and traumatic unconsciousness. Correlation of experimental and clinical observations of blunt head injuries. Brain, 97, 633–654. CrossRef Google Scholar PubMed
- Pal, D., Gupta, R.K., Agarwal, S., Yadav, A., Ojha, B.K., Awasthi, A., & Narayana, P.A. (2012). Diffusion tensor tractography indices in patients with frontal lobe injury and its correlation with neuropsychological tests. Clinical Neurology and Neurosurgery, 114, 564–571. CrossRef Google Scholar PubMed
- Palacios, E., Sala-Llonch, R., Junque, C., Roig, T., Tormos, J., Bargallo, N., & Vendrell, P. (2012). White matter integrity related to functional working memory networks in traumatic brain injury. Neurology, 78, 852–860. CrossRef Google Scholar PubMed
- Palacios, E., Sala-Llonch, R., Junque, C., Roig, T., Tormos, J., Bargallo, N., & Vendrell, P. (2013). Resting-state functional magnetic resonance imaging activity and connectivity and cognitive outcome in traumatic brain injury. JAMA Neurology, 70, 845–851. CrossRef Google Scholar PubMed
- Pandit, A., Expert, P., Lambiotte, R., Bonelle, V., Leech, R., Turkheimer, F., & Sharp, D. (2013). Traumatic brain injury impairs small-world topology. Neurology, 80, 1826–1833. CrossRef Google Scholar PubMed
- Peerless, S.J., & Rewcastle, N.B. (1967). Shear injuries of the brain. Canadian Medical Association Journal, 96, 577. Google Scholar
- Perlbarg, V., Puybasset, L., Tollard, E., Lehéricy, S., Benali, H., & Galanaud, D. (2009). Relation between brain lesion location and clinical outcome in patients with severe traumatic brain injury: A diffusion tensor imaging study using voxel-based approaches. Human Brain Mapping, 30, 3924–3933. CrossRef Google Scholar PubMed
- Petrie, E.C., Cross, D.J., Yarnykh, V.L., Richards, T., Martin, N.M., Pagulayan, K., & Peskind, E.R. (2014). Neuroimaging, behavioral, and psychological sequelae of repetitive combined blast/impact mild traumatic brain injury in Iraq and Afghanistan War Veterans. Journal of Neurotrauma, 31, 425–436. CrossRef Google Scholar PubMed
- Povlishock, J.T., Becker, D.P., Cheng, C.L.Y., & Vaughan, G.W. (1983). Axonal change in minor head injury. Journal of Neuropathology & Experimental Neurology, 42(3), 225–242. CrossRef Google Scholar PubMed
- Povlishock, J.T., & Christman, C.W. (1995). The pathobiology of traumatically induced axonal injury in animals and humans: A review of current thoughts. Journal of Neurotrauma, 12, 555–564. CrossRef Google Scholar PubMed
- Reinarz, S.J., Coffman, C.E., Smoker, W.R., & Godersky, J.C. (1988). MR imaging of the corpus callosum: Normal and pathologic findings and correlation with CT. AJNR: American Journal of Neuroradiology, 9, 649–656. Google Scholar
- Ruff, R.L., Riechers, R.G., Wang, X.-F., Piero, T., & Ruff, S.S. (2012). A case-control study examining whether neurological deficits and PTSD in combat veterans are related to episodes of mild TBI. BMJ Open, 2, 1–12. CrossRef Google Scholar PubMed
- Ruppin, E., & Reggia, J.. (1995). Patterns of functional damage in neural network models of associative memory. Neural Computation, 7, 1105–1127. CrossRef Google Scholar PubMed
- Ryu, J., Horkayne-Szakaly, I., Xu, L., Pletnikova, O., Leri, F., Eberhart, C., & Koliatsos, V.E. (2014). The problem of axonal injury in the brains of veterans with histories of blast exposure. Acta Neuropathologica Communications, 2, 1–14. CrossRef Google Scholar PubMed
- Salmond, C.H., Menon, D.K., Chatfield, D.A., Williams, G.B., Pena, A., Sahakian, B.J., & Pickard, J.D. (2006). Diffusion tensor imaging in chronic head injury survivors: Correlations with learning and memory indices. Neuroimage, 29, 117–124. CrossRef Google Scholar PubMed
- Sharp, D., Beckmann, C., Greenwood, R., Kinnunen, K., Bonelle, V., De Boissezon, X., & Leech, R. (2011). Default mode network functional and structural connectivity after traumatic brain injury. Brain, 134, 2233–2247. CrossRef Google Scholar PubMed
- Sharp, D., Scott, G., & Leech, R. (2014). Network dysfunction after traumatic brain injury. Nature Reviews Neurology, 10, 156–166. CrossRef Google Scholar PubMed
- Shenton, M.E., Hamoda, H.M., Schneiderman, J.S., Bouix, S., Pasternak, O., Rathi, Y., & Zafonte, R. (2012). A review of magnetic resonance imaging and diffusion tensor imaging findings in mild traumatic brain injury. Brain Imaging and Behavior, 6, 137–192. CrossRef Google Scholar PubMed
- Shumskaya, E., Andriessen, T., Norris, D., & Vos, P. (2012). Abnormal whole-brain functional networks in homogeneous acute mild traumatic brain injury. Neurology, 79, 175–182. CrossRef Google Scholar PubMed
- Sidaros, A., Engberg, A.W., Sidaros, K., Liptrot, M.G., Herning, M., Petersen, P., & Rostrup, E. (2008). Diffusion tensor imaging during recovery from severe traumatic brain injury and relation to clinical outcome: A longitudinal study. Brain, 131, 559–572. CrossRef Google Scholar PubMed
- Skoglund, T.S., Nilsson, D., Ljungberg, M., Jönsson, L., & Rydenhag, B. (2008). Long-term follow-up of a patient with traumatic brain injury using diffusion tensor imaging. Acta Radiologica, 49, 98–100. CrossRef Google Scholar PubMed
- Smith, D.H., Meaney, D.F., & Shull, W.H. (2003). Diffuse axonal injury in head trauma. Journal of Head Trauma Rehabilitation, 18, 307–316. CrossRef Google Scholar PubMed
- Smith, S.M. (2012). The future of FMRI connectivity. Neuroimage, 62, 1257–1266. CrossRef Google Scholar PubMed
- Smits, M., Houston, G.C., Dippel, D.W.J., Wielopolski, P.A., Vernooij, M.W., Koudstaal, P.J., & van Der Lugt, A. (2011). Microstructural brain injury in post-concussion syndrome after minor head injury. Neuroradiology, 53(8), 553–563. CrossRef Google Scholar PubMed
- Sorg, S.F., Delano-Wood, L., Luc, N., Schiehser, D.M., Hanson, K.L., Nation, D.A., & Meloy, M. (2013). White matter integrity in veterans with mild traumatic brain injury: Associations with executive function and loss of consciousness. The Journal of Head Trauma Rehabilitation, 29(1), 21–32. CrossRef Google Scholar PubMed
- Sours, C., Zhuo, J., Janowich, J., Aarabi, B., Shanmuganathan, K., & Gullapalli, R. (2013). Default mode network interference in mild traumatic brain injury – A pilot resting state study. Brain Research, 1537, 201–215. CrossRef Google Scholar PubMed
- Sporns, O. (2014). Contributions and challenges for network models in cognitive neuroscience. Nature Neuroscience, 17, 652–660. CrossRef Google Scholar PubMed
- Stevens, M., Lovejoy, D., Kim, J., Oakes, H., Kureshi, I., & Witt, S. (2012). Multiple resting state network functional connectivity abnormalities in mild traumatic brain injury. Brain Imaging and Behavior, 6, 293–318. CrossRef Google Scholar PubMed
- Strich, S.J. (1956). Diffuse degeneration of the cerebral white matter in severe dementia following head injury. Journal of Neurology, Neurosurgery, and Psychiatry, 19, 163. CrossRef Google Scholar PubMed
- Suskauer, S.J., & Huisman, T.A. (2009). Neuroimaging in pediatric traumatic brain injury: Current and future predictors of functional outcome. Developmental Disabilities Research Reviews, 15(2), 117–123. CrossRef Google Scholar PubMed
- Taber, K.H., & Hurley, R.A. (2013). Update on mild traumatic brain injury: Neuropathology and structural imaging. The Journal of Neuropsychiatry and Clinical Neurosciences, 25(1), 1–5. CrossRef Google Scholar PubMed
- Taber, K.H., Hurley, R.A., Haswell, C.C., Rowland, J.A., Hurt, S.D., Lamar, C.D., & Morey, R.A. (2015). White matter compromise in veterans exposed to primary blast forces. Journal of Head Trauma Rehabilitation, 30(1), E15–E25. CrossRef Google Scholar PubMed
- Tang, L., Ge, Y., Sodickson, D., Miles, L., Zhou, Y., Reaume, J., & Grossman, R. (2011). Thalamic resting-state functional networks: Disruption in patients with mild traumatic brain injury. Radiology, 260, 831–840. CrossRef Google Scholar PubMed
- Tate, D.F., & Bigler, E.D. (2000). Fornix and hippocampal atrophy in traumatic brain injury. Learning & Memory, 7(6), 442–446. CrossRef Google Scholar PubMed
- Tomaszczyk, J.C., Green, N.L., Frasca, D., Colella, B., Turner, G.R., Christensen, B.K., & Green, R.E.A. (2014). Negative neuroplasticity in chronic traumatic brain injury and implications for neurorehabilitation. Neuropsychology Review, 24, 409–427. Google Scholar PubMed
- Tournier, J.D., Mori, S., & Leemans, A. (2011). Diffusion tensor imaging and beyond. Magnetic Resonance in Medicine, 65(6), 1532–1556. CrossRef Google Scholar PubMed
- van den Heuvel, M.P., & Sporns, O. (2011). Rich-club organization of the human connectome. The Journal of Neuroscience, 31(44), 15775–15786. CrossRef Google Scholar PubMed
- Venkatesan, U., Dennis, N., & Hillary, F. (2015). Chronology and chronicity of altered resting-state functional connectivity after traumatic brain injury. Journal of Neurotrauma, 32, 252–264. CrossRef Google Scholar PubMed
- Voss, H.U., Uluç, A.M., Dyke, J.P., Watts, R., Kobylarz, E.J., McCandliss, B.D., & Vallabhajosula, S. (2006). Possible axonal regrowth in late recovery from the minimally conscious state. Journal of Clinical Investigation, 116(7), 2005. CrossRef Google Scholar PubMed
- Wäljas, M., Lange, R.T., Hakulinen, U., Huhtala, H., Dastidar, P., Hartikainen, K., & Iverson, G.L. (2014). Biopsychosocial outcome after uncomplicated mild traumatic brain injury. Journal of Neurotrauma, 31(1), 108–124. CrossRef Google Scholar PubMed
- Wang, J.Y., Bakhadirov, K., Abdi, H., Devous, M.D., Marquez de la Plata, C.D., Moore, C., & Diaz-Arrastia, R. (2011). Longitudinal changes of structural connectivity in traumatic axonal injury. Neurology, 77, 818–826. CrossRef Google Scholar PubMed
- Warden, D.L., French, L.M., Shupenko, L., Fargus, J., Riedy, G., Erickson, M.E., & Moore, D.F. (2009). Case report of a soldier with primary blast brain injury. Neuroimage, 47, T152–T153. CrossRef Google Scholar
- Watts, R., Thomas, A., Filippi, C.G., Nickerson, J.P., & Freeman, K. (2014). Potholes and molehills: Bias in the diagnostic performance of diffusion-tensor imaging in concussion. Radiology, 272(1), 217–223. CrossRef Google Scholar PubMed
- Wilde, E.A., Hunter, J.V., & Bigler, E.D. (2012). Neuroimaging in neurorehabilitation. NeuroRehabilitation, 31, 223–226. Google Scholar PubMed
- Xu, J., Rasmussen, I.-A., Lagopoulos, J., & Håberg, A. (2007). Diffuse axonal injury in severe traumatic brain injury visualized using high-resolution diffusion tensor imaging. Journal of Neurotrauma, 24(5), 753–765. CrossRef Google Scholar PubMed
- Yeh, P.H., Wang, B., Oakes, T.R., French, L.M., Pan, H., Graner, J., & Riedy, G. (2014). Postconcussional disorder and PTSD symptoms of military-related traumatic brain injury associated with compromised neurocircuitry: Neurocircuitry in military TBI and clinical symptoms. Human Brain Mapping, 35, 2652–2673. CrossRef Google Scholar
- Yeo, B., Krienen, F., Sepulcre, J., Sabuncu, M., Lashkari, D., Hollinshead, M., & Buckner, R. (2011). The organization of the human cerebral cortex estimated by intrinsic functional connectivity. Journal of Neurophysiology, 106, 1125–1165. Google Scholar PubMed
- Yuh, E.L., Cooper, S.R., Mukherjee, P., Yue, J.K., Lingsma, H.F., Gordon, W.A., & Vassar, M.J. (2014). Diffusion tensor imaging for outcome prediction in mild traumatic brain injury: A TRACK-TBI study. Journal of Neurotrauma, 31(17), 1457–1477. CrossRef Google Scholar PubMed
- Zhang, S., & Li, C.S. (2012). Functional connectivity mapping of the human precuneus by resting state fMRI. Neuroimage, 59, 3548–3562. CrossRef Google Scholar PubMed
- Zhou, Y., Lui, Y., Zuo, X., Milham, M., Reaume, J., Grossman, R., & Ge, Y. (2014). Characterization of thalamo-cortical association using amplitude and connectivity of functional MRI in mild traumatic brain injury. Journal of Magnetic Resonance Imaging, 39, 1558–1568. CrossRef Google Scholar PubMed
- Zhou, Y., Milham, M., Lui, Y., Miles, L., Reaume, J., Sodickson, D., & Ge, Y. (2012). Default-mode network disruption in mild traumatic brain injury. Radiology, 265, 882–892. CrossRef Google Scholar PubMed
The objective was to review the literature on diffusion tensor imaging as well as resting-state functional magnetic resonance imaging and electroencephalography (EEG) to unveil neuroanatomical and neurophysiological substrates of Alzheimer’s disease (AD) as a brain neural network pathology affecting structural and functional cortical connectivity underlying human cognition.
We reviewed papers registered in PubMed and other scientific repositories on the use of these techniques in amnesic mild cognitive impairment (MCI) and clinically mild AD dementia patients compared to cognitively intact elderly individuals (Controls).
Hundreds of peer-reviewed (cross-sectional and longitudinal) papers have shown in patients with MCI and mild AD compared to Controls (1) impairment of callosal (splenium), thalamic, and anterior–posterior white matter bundles; (2) reduced correlation of resting state blood oxygen level-dependent activity across several intrinsic brain circuits including default mode and attention-related networks; and (3) abnormal power and functional coupling of resting state cortical EEG rhythms. Clinical applications of these measures are still limited.
Structural and functional (in vivo) cortical connectivity measures represent a reliable marker of cerebral reserve capacity and should be used to predict and monitor the evolution of AD and its relative impact on cognitive domains in pre-clinical, prodromal, and dementia stages of AD. (JINS, 2016,22, 138–163)
- Acosta-Cabronero, J., Williams, G.B., Pengas, G., & Nestor, P.J. (2010). Absolute diffusivities define the landscape of white matter degeneration in Alzheimer’s disease. Brain, 133(Pt 2), 529–539. CrossRef Google Scholar
- Adriaanse, S.M., Sanz-Arigita, E.J., Binnewijzend, M.A., Ossenkoppele, R., Tolboom, N., van Assema, D.M., & van Berckel, B.N. (2014). Amyloid and its association with default network integrity in Alzheimer’s disease. Human Brain Mapping, 35(3), 779–791. CrossRef Google Scholar PubMed
- Agosta, F., Pievani, M., Geroldi, C., Copetti, M., Frisoni, G.B., & Filippi, M. (2012). Resting state fMRI in Alzheimer’s disease: Beyond the default mode network. Neurobiology of Aging, 33(8), 1564–1578. CrossRef Google Scholar PubMed
- Agosta, F., Rocca, M.A., Pagani, E., Absinta, M., Magnani, G., Marcone, A., & Filippi, M. (2010). Sensorimotor network rewiring in mild cognitive impairment and Alzheimer’s disease. Human Brain Mapping, 31(4), 515–525. Google Scholar PubMed
- Ahmed, Z., Cooper, J., Murray, T.K., Garn, K., McNaughton, E., Clarke, H., & O’Neill, M.J. (2014). A novel in vivo model of tau propagation with rapid and progressive neurofibrillary tangle pathology: The pattern of spread is determined by connectivity, not proximity. Acta Neuropathologica, 127(5), 667–683. CrossRef Google Scholar
- Arenaza-Urquijo, E.M., Landeau, B., La Joie, R., Mevel, K., Mezenge, F., Perrotin, A., & Chetelat, G. (2013). Relationships between years of education and gray matter volume, metabolism and functional connectivity in healthy elders. Neuroimage, 83C, 450–457. CrossRef Google Scholar
- Babiloni, C., Carducci, F., Lizio, R., Vecchio, F., Baglieri, A., Bernardini, S., & Frisoni, G.B. (2013). Resting state cortical electroencephalographic rhythms are related to gray matter volume in subjects with mild cognitive impairment and Alzheimer’s disease. Human Brain Mapping, 34(6), 1427–1446. CrossRef Google Scholar PubMed
- Babiloni, C., Del Percio, C., Boccardi, M., Lizio, R., Lopez, S., Carducci, F., & Frisoni, G.B. (2015). Occipital sources of resting-state alpha rhythms are related to local gray matter density in subjects with amnesic mild cognitive impairment and Alzheimer’s disease. Neurobiology of Aging, 36(2), 556–570. CrossRef Google Scholar PubMed
- Babiloni, C., Ferri, R., Binetti, G., Vecchio, F., Frisoni, G.B., Lanuzza, B., & Rossini, P.M. (2009). Directionality of EEG synchronization in Alzheimer’s disease subjects. Neurobiology of Aging, 30(1), 93–102. CrossRef Google Scholar PubMed
- Babiloni, C., Frisoni, G., Steriade, M., Bresciani, L., Binetti, G., Del Percio, C., & Rossini, P.M. (2006). Frontal white matter volume and delta EEG sources negatively correlate in awake subjects with mild cognitive impairment and Alzheimer’s disease. Clinical Neurophysiology, 117(5), 1113–1129. CrossRef Google Scholar PubMed
- Babiloni, C., Frisoni, G.B., Pievani, M., Vecchio, F., Lizio, R., Buttiglione, M., & Rossini, P.M. (2009). Hippocampal volume and cortical sources of EEG alpha rhythms in mild cognitive impairment and Alzheimer disease. Neuroimage, 44(1), 123–135. CrossRef Google Scholar PubMed
- Babiloni, C., Pievani, M., Vecchio, F., Geroldi, C., Eusebi, F., Fracassi, C., & Frisoni, G.B. (2009). White-matter lesions along the cholinergic tracts are related to cortical sources of EEG rhythms in amnesic mild cognitive impairment. Human Brain Mapping, 30(5), 1431–1443. CrossRef Google Scholar PubMed
- Bai, F., Liao, W., Yue, C., Pu, M., Shi, Y., Yu, H., & Zhang, Z. (2014). Genetics pathway-based imaging approaches in Chinese Han population with Alzheimer’s disease risk. Brain Structure & Function. [Epub ahead of print]. Google Scholar PubMed
- Bai, F., Watson, D.R., Shi, Y., Wang, Y., Yue, C., Yuhuan, Teng, & Zhang, Z. (2011). Specifically progressive deficits of brain functional marker in amnestic type mild cognitive impairment. PLoS One, 6(9), e24271. CrossRef Google Scholar PubMed
- Bai, F., Watson, D.R., Yu, H., Shi, Y., Yuan, Y., & Zhang, Z. (2009). Abnormal resting-state functional connectivity of posterior cingulate cortex in amnestic type mild cognitive impairment. Brain Research, 1302, 167–174. CrossRef Google Scholar PubMed
- Balachandar, R., John, J.P., Saini, J., Kumar, K.J., Joshi, H., Sadanand, S., & Bharath, S. (2015). A study of structural and functional connectivity in early Alzheimer’s disease using rest fMRI and diffusion tensor imaging. International Journal of Geriatric Psychiatry, 30(5), 497–504. CrossRef Google Scholar PubMed
- Balthazar, M.L., Pereira, F.R., Lopes, T.M., da Silva, E.L., Coan, A.C., Campos, B.M., & Cendes, F. (2013). Neuropsychiatric symptoms in Alzheimer’s disease are related to functional connectivity alterations in the salience network. Human Brain Mapping. Google Scholar PubMed
- Basser, P.J., Mattiello, J., & LeBihan, D. (1994). MR diffusion tensor spectroscopy and imaging. Biophysical Journal, 66(1), 259–267. CrossRef Google Scholar
- Beckmann, C.F., DeLuca, M., Devlin, J.T., & Smith, S.M. (2005). Investigations into resting-state connectivity using independent component analysis. Philosophical Transactions of the Royal Society of London. Series B, Biological Sciences, 360(1457), 1001–1013. CrossRef Google Scholar PubMed
- Bendlin, B.B., Carlsson, C.M., Johnson, S.C., Zetterberg, H., Blennow, K., Willette, A.A., & Sager, M.A. (2012). CSF T-Tau/Abeta42 predicts white matter microstructure in healthy adults at risk for Alzheimer’s disease. PLoS One, 7(6), e37720. CrossRef Google Scholar PubMed
- Bendlin, B.B., Ries, M.L., Canu, E., Sodhi, A., Lazar, M., Alexander, A.L., & Johnson, S.C. (2010). White matter is altered with parental family history of Alzheimer’s disease. Alzheimers & Dementia, 6(5), 394–403. CrossRef Google Scholar PubMed
- Binnewijzend, M.A., Schoonheim, M.M., Sanz-Arigita, E., Wink, A.M., van der Flier, W.M., Tolboom, N., & Barkhof, F. (2012). Resting-state fMRI changes in Alzheimer’s disease and mild cognitive impairment. Neurobiology of Aging, 33(9), 2018–2028. CrossRef Google Scholar PubMed
- Biswal, B., Yetkin, F.Z., Haughton, V.M., & Hyde, J.S. (1995). Functional connectivity in the motor cortex of resting human brain using echo-planar MRI. Magnetic Resonance in Medicine, 34(4), 537–541. CrossRef Google Scholar PubMed
- Biswal, B.B., Mennes, M., Zuo, X.N., Gohel, S., Kelly, C., Smith, S.M., & Milham, M.P. (2010). Toward discovery science of human brain function. Proceedings of the National Academy of Sciences of the United States of America, 107(10), 4734–4739. CrossRef Google Scholar PubMed
- Bosch, B., Arenaza-Urquijo, E.M., Rami, L., Sala-Llonch, R., Junque, C., Sole-Padulles, C., & Bartres-Faz, D. (2012). Multiple DTI index analysis in normal aging, amnestic MCI and AD. Relationship with neuropsychological performance. Neurobiology of Aging, 33(1), 61–74. CrossRef Google Scholar
- Bozoki, A.C., Korolev, I.O., Davis, N.C., Hoisington, L.A., & Berger, K.L. (2012). Disruption of limbic white matter pathways in mild cognitive impairment and Alzheimer’s disease: A DTI/FDG-PET study. Human Brain Mapping, 33(8), 1792–1802. CrossRef Google Scholar PubMed
- Bozzali, M., Dowling, C., Serra, L., Spano, B., Torso, M., Marra, C., & Cercignani, M. (2014). The impact of cognitive reserve on brain functional connectivity in Alzheimer’s Disease. Journal of Alzheimers Disease, 44, 243–250. Google Scholar PubMed
- Bozzali, M., Falini, A., Franceschi, M., Cercignani, M., Zuffi, M., Scotti, G., & Filippi, M. (2002). White matter damage in Alzheimer’s disease assessed in vivo using diffusion tensor magnetic resonance imaging. Journal of Neurology, Neurosurgery, and Psychiatry, 72(6), 742–746. CrossRef Google Scholar
- Bozzali, M., Franceschi, M., Falini, A., Pontesilli, S., Cercignani, M., Magnani, G., & Filippi, M. (2001). Quantification of tissue damage in AD using diffusion tensor and magnetization transfer MRI. Neurology, 57(6), 1135–1137. CrossRef Google Scholar PubMed
- Braak, H., & Del Tredici, K. (2011). Alzheimer’s pathogenesis: Is there neuron-to-neuron propagation? Acta Neuropathologica, 121(5), 589–595. CrossRef Google Scholar PubMed
- Braskie, M.N., Jahanshad, N., Stein, J.L., Barysheva, M., Johnson, K., McMahon, K.L., & Thompson, P.M. (2012). Relationship of a variant in the NTRK1 gene to white matter microstructure in young adults. The Journal of Neuroscience, 32(17), 5964–5972. CrossRef Google Scholar PubMed
- Braskie, M.N., Jahanshad, N., Stein, J.L., Barysheva, M., McMahon, K.L., de Zubicaray, G.I., & Thompson, P.M. (2011). Common Alzheimer’s disease risk variant within the CLU gene affects white matter microstructure in young adults. The Journal of Neuroscience, 31(18), 6764–6770. CrossRef Google Scholar PubMed
- Brier, M.R., Thomas, J.B., Snyder, A.Z., Benzinger, T.L., Zhang, D., Raichle, M.E., & Ances, B.M. (2012). Loss of intranetwork and internetwork resting state functional connections with Alzheimer’s disease progression. The Journal of Neuroscience, 32(26), 8890–8899. CrossRef Google Scholar PubMed
- Brown, J.A., Terashima, K.H., Burggren, A.C., Ercoli, L.M., Miller, K.J., Small, G.W., & Bookheimer, S.Y. (2011). Brain network local interconnectivity loss in aging APOE-4 allele carriers. Proceedings of the National Academy of Sciences of the United States of America, 108(51), 20760–20765. CrossRef Google Scholar PubMed
- Buckner, R.L., Andrews-Hanna, J.R., & Schacter, D.L. (2008). The brain’s default network: Anatomy, function, and relevance to disease. Annals of the New York Academy of Sciences, 1124, 1–38. CrossRef Google Scholar PubMed
- Buckner, R.L., Sepulcre, J., Talukdar, T., Krienen, F.M., Liu, H., Hedden, T., & Johnson, K.A. (2009). Cortical hubs revealed by intrinsic functional connectivity: Mapping, assessment of stability, and relation to Alzheimer’s disease. The Journal of Neuroscience, 29(6), 1860–1873. CrossRef Google Scholar
- Buckner, R.L., Snyder, A.Z., Shannon, B.J., LaRossa, G., Sachs, R., Fotenos, A.F., & Mintun, M.A. (2005). Molecular, structural, and functional characterization of Alzheimer’s disease: Evidence for a relationship between default activity, amyloid, and memory. The Journal of Neuroscience, 25(34), 7709–7717. CrossRef Google Scholar PubMed
- Bullmore, E., & Sporns, O. (2009). Complex brain networks: Graph theoretical analysis of structural and functional systems. Nature Reviews. Neuroscience, 10(3), 186–198. CrossRef Google Scholar PubMed
- Chao, L.L., Decarli, C., Kriger, S., Truran, D., Zhang, Y., Laxamana, J., & Weiner, M.W. (2013). Associations between white matter hyperintensities and beta amyloid on integrity of projection, association, and limbic fiber tracts measured with diffusion tensor MRI. PLoS One, 8(6), e65175. CrossRef Google Scholar
- Chen, T.F., Chen, Y.F., Cheng, T.W., Hua, M.S., Liu, H.M., & Chiu, M.J. (2009). Executive dysfunction and periventricular diffusion tensor changes in amnesic mild cognitive impairment and early Alzheimer’s disease. Human Brain Mapping, 30(11), 3826–3836. CrossRef Google Scholar PubMed
- Chen, Y., Chen, K., Zhang, J., Li, X., Shu, N., Wang, J., & Reiman, E.M. (2014). Disrupted functional and structural networks in cognitively normal elderly subjects with the APOE varepsilon4 Allele. Neuropsychopharmacology, 40, 1181–1191. CrossRef Google Scholar PubMed
- Chetelat, G., Desgranges, B., De La Sayette, V., Viader, F., Eustache, F., & Baron, J.C. (2002). Mapping gray matter loss with voxel-based morphometry in mild cognitive impairment. Neuroreport, 13(15), 1939–1943. CrossRef Google Scholar PubMed
- Chetelat, G., Landeau, B., Salmon, E., Yakushev, I., Bahri, M.A., Mezenge, F., & Fellgiebel, A. (2013). Relationships between brain metabolism decrease in normal aging and changes in structural and functional connectivity. Neuroimage, 76, 167–177. CrossRef Google Scholar PubMed
- Choo, I.H., Lee, D.Y., Youn, J.C., Jhoo, J.H., Kim, K.W., Lee, D.S., & Woo, J.I. (2007). Topographic patterns of brain functional impairment progression according to clinical severity staging in 116 Alzheimer disease patients: FDG-PET study. Alzheimer Disease and Associated Disorders, 21(2), 77–84. CrossRef Google Scholar PubMed
- Cirrito, J.R., Kang, J.E., Lee, J., Stewart, F.R., Verges, D.K., Silverio, L.M., & Holtzman, D.M. (2008). Endocytosis is required for synaptic activity-dependent release of amyloid-beta in vivo. Neuron, 58(1), 42–51. CrossRef Google Scholar PubMed
- Cirrito, J.R., Yamada, K.A., Finn, M.B., Sloviter, R.S., Bales, K.R., May, P.C., & Holtzman, D.M. (2005). Synaptic activity regulates interstitial fluid amyloid-beta levels in vivo. Neuron, 48(6), 913–922. CrossRef Google Scholar
- Corbetta, M., & Shulman, G.L. (2002). Control of goal-directed and stimulus-driven attention in the brain. Nature Reviews. Neuroscience, 3(3), 201–215. CrossRef Google Scholar
- Cross, D.J., Anzai, Y., Petrie, E.C., Martin, N., Richards, T.L., Maravilla, K.R., & Minoshima, S. (2013). Loss of olfactory tract integrity affects cortical metabolism in the brain and olfactory regions in aging and mild cognitive impairment. Journal of Nuclear Medicine, 54(8), 1278–1284. CrossRef Google Scholar
- Crossley, N.A., Mechelli, A., Scott, J., Carletti, F., Fox, P.T., McGuire, P., & Bullmore, E.T. (2014). The hubs of the human connectome are generally implicated in the anatomy of brain disorders. Brain, 137(Pt 8), 2382–2395. CrossRef Google Scholar PubMed
- Crossley, N.A., Mechelli, A., Vertes, P.E., Winton-Brown, T.T., Patel, A.X., Ginestet, C.E., & Bullmore, E.T. (2013). Cognitive relevance of the community structure of the human brain functional coactivation network. Proceedings of the National Academy of Sciences of the United States of America, 110(28), 11583–11588. CrossRef Google Scholar PubMed
- Daianu, M., Dennis, E.L., Jahanshad, N., Nir, T.M., Toga, A.W., Jack, C.R., & Thompson, P.M. (2013). Alzheimer’s disease disrupts rich club organization in brain connectivity networks. IEEE International Symposium on Biomedical Imaging, 2013, 266–269. Google Scholar
- Daianu, M., Jahanshad, N., Nir, T.M., Jack, C.R., Weiner, M.W., Bernstein, M.A., & Thompson, P.M. (2015). Rich club analysis in the Alzheimer’s disease connectome reveals a relatively undisturbed structural core network. Human Brain Mapping, 36(8), 3087–3103. CrossRef Google Scholar PubMed
- Damoiseaux, J.S., & Greicius, M.D. (2009). Greater than the sum of its parts: A review of studies combining structural connectivity and resting-state functional connectivity. Brain Structure & Function, 213(6), 525–533. CrossRef Google Scholar
- Damoiseaux, J.S., Rombouts, S.A., Barkhof, F., Scheltens, P., Stam, C.J., Smith, S.M., & Beckmann, C.F. (2006). Consistent resting-state networks across healthy subjects. Proceedings of the National Academy of Sciences of the United States of America, 103(37), 13848–13853. CrossRef Google Scholar PubMed
- Damoiseaux, J.S., Seeley, W.W., Zhou, J., Shirer, W.R., Coppola, G., Karydas, A., & Greicius, M.D. (2012). Gender modulates the APOE {varepsilon}4 effect in healthy older adults: Convergent evidence from functional brain connectivity and spinal fluid Tau levels. The Journal of Neuroscience, 32(24), 8254–8262. CrossRef Google Scholar
- Daunizeau, J., David, O., & Stephan, K.E. (2011). Dynamic causal modelling: A critical review of the biophysical and statistical foundations. Neuroimage, 58(2), 312–322. CrossRef Google Scholar
- Dauwels, J., Vialatte, F., Musha, T., & Cichocki, A. (2010). A comparative study of synchrony measures for the early diagnosis of Alzheimer’s disease based on EEG. Neuroimage, 49(1), 668–693. CrossRef Google Scholar PubMed
- Deco, G., & Corbetta, M. (2011). The dynamical balance of the brain at rest. The Neuroscientist, 17(1), 107–123. CrossRef Google Scholar PubMed
- Dell’Acqua, F., & Catani, M. (2012). Structural human brain networks: Hot topics in diffusion tractography. Current Opinion in Neurology, 25(4), 375–383. Google Scholar
- Dennis, E.L., & Thompson, P.M. (2014). Functional brain connectivity using fMRI in aging and Alzheimer’s disease. Neuropsychology Review, 24(1), 49–62. CrossRef Google Scholar PubMed
- Doria, V., Beckmann, C.F., Arichi, T., Merchant, N., Groppo, M., Turkheimer, F.E., & Edwards, A.D. (2010). Emergence of resting state networks in the preterm human brain. Proceedings of the National Academy of Sciences of the United States of America, 107(46), 20015–20020. CrossRef Google Scholar PubMed
- Douaud, G., Jbabdi, S., Behrens, T.E., Menke, R.A., Gass, A., Monsch, A.U., & Smith, S. (2011). DTI measures in crossing-fibre areas: Increased diffusion anisotropy reveals early white matter alteration in MCI and mild Alzheimer’s disease. Neuroimage, 55(3), 880–890. CrossRef Google Scholar PubMed
- Douaud, G., Menke, R.A., Gass, A., Monsch, A.U., Rao, A., Whitcher, B., & Smith, S. (2013). Brain microstructure reveals early abnormalities more than two years prior to clinical progression from mild cognitive impairment to Alzheimer’s disease. The Journal of Neuroscience, 33(5), 2147–2155. CrossRef Google Scholar PubMed
- Drzezga, A., Becker, J.A., Van Dijk, K.R., Sreenivasan, A., Talukdar, T., Sullivan, C., & Sperling, R.A. (2011). Neuronal dysfunction and disconnection of cortical hubs in non-demented subjects with elevated amyloid burden. Brain, 134(Pt 6), 1635–1646. CrossRef Google Scholar
- Dubois, B., Feldman, H.H., Jacova, C., Hampel, H., Molinuevo, J.L., Blennow, K., & Cummings, J.L. (2014). Advancing research diagnostic criteria for Alzheimer’s disease: The IWG-2 criteria. The Lancet. Neurology, 13(6), 614–629. CrossRef Google Scholar PubMed
- Dyrba, M., Barkhof, F., Fellgiebel, A., Filippi, M., Hausner, L., Hauenstein, K., & Teipel, S.J. (2015). Predicting prodromal Alzheimer’s disease in subjects with mild cognitive impairment using machine learning classification of multimodal multicenter diffusion-tensor and magnetic resonance imaging data. J Neuroimaging, 25, 738–747. CrossRef Google Scholar
- Dyrba, M., Ewers, M., Wegrzyn, M., Kilimann, I., Plant, C., Oswald, A., & Teipel, S.J. (2013). Robust automated detection of microstructural white matter degeneration in Alzheimer’s disease using machine learning classification of multicenter DTI data. PLoS One, 8(5), e64925. CrossRef Google Scholar
- Dyrba, M., Grothe, M., Kirste, T., & Teipel, S.J. (2015). Multimodal analysis of functional and structural disconnection in Alzheimer’s disease using multiple kernel SVM. Human Brain Mapping, 36(6), 2118–2131. CrossRef Google Scholar
- Dziewczapolski, G., Glogowski, C.M., Masliah, E., & Heinemann, S.F. (2009). Deletion of the alpha 7 nicotinic acetylcholine receptor gene improves cognitive deficits and synaptic pathology in a mouse model of Alzheimer’s disease. The Journal of Neuroscience, 29(27), 8805–8815. CrossRef Google Scholar
- Fellgiebel, A., Dellani, P.R., Greverus, D., Scheurich, A., Stoeter, P., & Muller, M.J. (2006). Predicting conversion to dementia in mild cognitive impairment by volumetric and diffusivity measurements of the hippocampus. Psychiatry Research, 146(3), 283–287. CrossRef Google Scholar PubMed
- Fellgiebel, A., Muller, M.J., Wille, P., Dellani, P.R., Scheurich, A., Schmidt, L.G., & Stoeter, P. (2005). Color-coded diffusion-tensor-imaging of posterior cingulate fiber tracts in mild cognitive impairment. Neurobiology of Aging, 26(8), 1193–1198. CrossRef Google Scholar PubMed
- Fellgiebel, A., Schermuly, I., Gerhard, A., Keller, I., Albrecht, J., Weibrich, C., & Stoeter, P. (2008). Functional relevant loss of long association fibre tracts integrity in early Alzheimer’s disease. Neuropsychologia, 46(6), 1698–1706. CrossRef Google Scholar PubMed
- Fischer, F.U., Scheurich, A., Wegrzyn, M., Schermuly, I., Bokde, A.L., Kloppel, S., & Fellgiebel, A. (2012). Automated tractography of the cingulate bundle in Alzheimer’s disease: A multicenter DTI study. Journal of Magnetic Resonance Imaging, 36, 84–91. CrossRef Google Scholar PubMed
- Fischer, F.U., Wolf, D., Scheurich, A., & Fellgiebel, A. (2015). Altered whole-brain white matter networks in preclinical Alzheimer’s disease. Neuroimage: Clinical, 8, 660–666. CrossRef Google Scholar PubMed
- Fletcher, E., Raman, M., Huebner, P., Liu, A., Mungas, D., Carmichael, O., & DeCarli, C. (2013). Loss of fornix white matter volume as a predictor of cognitive impairment in cognitively normal elderly individuals. JAMA Neurology, 70(11), 1389–1395. CrossRef Google Scholar PubMed
- Forde, N.J., Ronan, L., Suckling, J., Scanlon, C., Neary, S., Holleran, L., & Cannon, D.M. (2014). Structural neuroimaging correlates of allelic variation of the BDNF val66met polymorphism. Neuroimage, 90, 280–289. CrossRef Google Scholar PubMed
- Fox, M.D., & Greicius, M. (2010). Clinical applications of resting state functional connectivity. Frontiers in Systems Neuroscience, 4, 19. Google Scholar
- Fox, M.D., & Raichle, M.E. (2007). Spontaneous fluctuations in brain activity observed with functional magnetic resonance imaging. Nature Reviews. Neuroscience, 8(9), 700–711. CrossRef Google Scholar PubMed
- Fox, M.D., Snyder, A.Z., Vincent, J.L., Corbetta, M., Van Essen, D.C., & Raichle, M.E. (2005). The human brain is intrinsically organized into dynamic, anticorrelated functional networks. Proceedings of the National Academy of Sciences of the United States of America, 102(27), 9673–9678. CrossRef Google Scholar PubMed
- Friese, U., Meindl, T., Herpertz, S.C., Reiser, M.F., Hampel, H., & Teipel, S.J. (2010). Diagnostic utility of novel MRI-based biomarkers for Alzheimer’s disease: Diffusion tensor imaging and deformation-based morphometry. Journal of Alzheimer’s Disease, 20(2), 477–490. CrossRef Google Scholar
- Friston, K.J., Harrison, L., & Penny, W. (2003). Dynamic causal modelling. Neuroimage, 19(4), 1273–1302. CrossRef Google Scholar
- Gili, T., Cercignani, M., Serra, L., Perri, R., Giove, F., Maraviglia, B., & Bozzali, M. (2011). Regional brain atrophy and functional disconnection across Alzheimer’s disease evolution. Journal of Neurology, Neurosurgery, and Psychiatry, 82(1), 58–66. CrossRef Google Scholar PubMed
- Glodzik, L., Kuceyeski, A., Rusinek, H., Tsui, W., Mosconi, L., Li, Y., & de Leon, M.J. (2014). Reduced glucose uptake and Abeta in brain regions with hyperintensities in connected white matter. Neuroimage, 100, 684–691. CrossRef Google Scholar PubMed
- Goebel, R., Roebroeck, A., Kim, D.-S., & Formisano, E. (2003). Investigating directed cortical interactions in time-resolved fMRI data using vector autoregressive modeling and Granger causality mapping. Magnetic Resonance Imaging, 21(10), 1251–1261. CrossRef Google Scholar PubMed
- Gold, B.T., Zhu, Z., Brown, C.A., Andersen, A.H., LaDu, M.J., Tai, L., & Smith, C.D. (2014). White matter integrity is associated with cerebrospinal fluid markers of Alzheimer’s disease in normal adults. Neurobiology of Aging, 35(10), 2263–2271. CrossRef Google Scholar PubMed
- Goncalves, S.I., de Munck, J.C., Pouwels, P.J., Schoonhoven, R., Kuijer, J.P., Maurits, N.M., & Lopes da Silva, F.H. (2006). Correlating the alpha rhythm to BOLD using simultaneous EEG/fMRI: Inter-subject variability. Neuroimage, 30(1), 203–213. CrossRef Google Scholar PubMed
- Gonzalez-Escamilla, G., Atienza, M., Garcia-Solis, D., & Cantero, J.L. (2014). Cerebral and blood correlates of reduced functional connectivity in mild cognitive impairment. Brain Structure & Function. [Epub ahead of print]. Google Scholar PubMed
- Grambaite, R., Selnes, P., Reinvang, I., Aarsland, D., Hessen, E., Gjerstad, L., & Fladby, T. (2011). Executive dysfunction in mild cognitive impairment is associated with changes in frontal and cingulate white matter tracts. Journal of Alzheimer’s Disease, 27(2), 453–462. Google Scholar PubMed
- Grana, M., Termenon, M., Savio, A., Gonzalez-Pinto, A., Echeveste, J., Perez, J.M., & Besga, A. (2011). Computer aided diagnosis system for Alzheimer disease using brain diffusion tensor imaging features selected by Pearson’s correlation. Neurosci Letters, 502(3), 225–229. CrossRef Google Scholar PubMed
- Granger, C.W.J. (1969). Investigating causal relations by econometric models and cross-spectral methods. Econometrica, 37(3), 424. CrossRef Google Scholar
- Greicius, M.D., Krasnow, B., Reiss, A.L., & Menon, V. (2003). Functional connectivity in the resting brain: A network analysis of the default mode hypothesis. Proceedings of the National Academy of Sciences of the United States of America, 100(1), 253–258. CrossRef Google Scholar PubMed
- Greicius, M.D., & Menon, V. (2004). Default-mode activity during a passive sensory task: Uncoupled from deactivation but impacting activation. Journal of Cognitive Neuroscience, 16(9), 1484–1492. CrossRef Google Scholar PubMed
- Greicius, M.D., Srivastava, G., Reiss, A.L., & Menon, V. (2004). Default-mode network activity distinguishes Alzheimer’s disease from healthy aging: Evidence from functional MRI. Proceedings of the National Academy of Sciences of the United States of America, 101(13), 4637–4642. CrossRef Google Scholar PubMed
- Greicius, M.D., Supekar, K., Menon, V., & Dougherty, R.F. (2009). Resting-state functional connectivity reflects structural connectivity in the default mode network. Cerebral Cortex, 19(1), 72–78. CrossRef Google Scholar PubMed
- Hahn, K., Myers, N., Prigarin, S., Rodenacker, K., Kurz, A., Forstl, H., & Sorg, C. (2013). Selectively and progressively disrupted structural connectivity of functional brain networks in Alzheimer’s disease - revealed by a novel framework to analyze edge distributions of networks detecting disruptions with strong statistical evidence. Neuroimage, 81, 96–109. CrossRef Google Scholar PubMed
- Hedden, T., Van Dijk, K.R., Becker, J.A., Mehta, A., Sperling, R.A., Johnson, K.A., & Buckner, R.L. (2009). Disruption of functional connectivity in clinically normal older adults harboring amyloid burden. The Journal of Neuroscience, 29(40), 12686–12694. CrossRef Google Scholar PubMed
- Heise, V., Filippini, N., Trachtenberg, A.J., Suri, S., Ebmeier, K.P., & Mackay, C.E. (2014). Apolipoprotein E genotype, gender and age modulate connectivity of the hippocampus in healthy adults. Neuroimage, 98, 23–30. CrossRef Google Scholar PubMed
- Horn, A., Ostwald, D., Reisert, M., & Blankenburg, F. (2014). The structural-functional connectome and the default mode network of the human brain. Neuroimage, 102(Pt 1), 142–151. CrossRef Google Scholar PubMed
- Hsieh, H.J., Kao, P.F., Huang, H.L., & Chou, Y.H. (2010). Cardiac stab injury: A fixed perfusion defect on Tc-99m sestamibi SPECT. Clinical Nuclear Medicine, 35(2), 121–122. CrossRef Google Scholar PubMed
- Huang, H., Fan, X., Weiner, M., Martin-Cook, K., Xiao, G., Davis, J., & Diaz-Arrastia, R. (2012). Distinctive disruption patterns of white matter tracts in Alzheimer’s disease with full diffusion tensor characterization. Neurobiology of Aging, 33(9), 2029–2045. CrossRef Google Scholar PubMed
- Huang, J., & Auchus, A.P. (2007). Diffusion tensor imaging of normal appearing white matter and its correlation with cognitive functioning in mild cognitive impairment and Alzheimer’s disease. Annals of the New York Academy of Sciences, 1097, 259–264. CrossRef Google Scholar PubMed
- Huang, J., Friedland, R.P., & Auchus, A.P. (2007). Diffusion tensor imaging of normal-appearing white matter in mild cognitive impairment and early Alzheimer disease: Preliminary evidence of axonal degeneration in the temporal lobe. AJNR American Journal of Neuroradiology, 28(10), 1943–1948. CrossRef Google Scholar PubMed
- Iturria-Medina, Y., Sotero, R.C., Toussaint, P.J., & Evans, A.C. (2014). Epidemic spreading model to characterize misfolded proteins propagation in aging and associated neurodegenerative disorders. PLoS Computational Biology, 10(11), e1003956. CrossRef Google Scholar PubMed
- Jack, C.R. Jr., Knopman, D.S., Jagust, W.J., Shaw, L.M., Aisen, P.S., Weiner, M.W., & Trojanowski, J.Q. (2010). Hypothetical model of dynamic biomarkers of the Alzheimer’s pathological cascade. The Lancet. Neurology, 9(1), 119–128. CrossRef Google Scholar PubMed
- Jacobs, H.I., Gronenschild, E.H., Evers, E.A., Ramakers, I.H., Hofman, P.A., Backes, W.H., & Van Boxtel, M.P. (2015). Visuospatial processing in early Alzheimer’s disease: A multimodal neuroimaging study. Cortex, 64, 394–406. CrossRef Google Scholar PubMed
- Jeurissen, B., Leemans, A., Tournier, J.D., Jones, D.K., & Sijbers, J. (2013). Investigating the prevalence of complex fiber configurations in white matter tissue with diffusion magnetic resonance imaging. Human Brain Mapping, 34(11), 2747–2766. CrossRef Google Scholar
- Jones, D.T., Vemuri, P., Murphy, M.C., Gunter, J.L., Senjem, M.L., Machulda, M.M., & Jack, C.R. Jr. (2012). Non-stationarity in the “resting brain’s” modular architecture. PLoS One, 7(6), e39731. CrossRef Google Scholar
- Kalus, P., Slotboom, J., Gallinat, J., Mahlberg, R., Cattapan-Ludewig, K., Wiest, R., & Kiefer, C. (2006). Examining the gateway to the limbic system with diffusion tensor imaging: The perforant pathway in dementia. Neuroimage, 30(3), 713–720. CrossRef Google Scholar PubMed
- Kantarci, K., Schwarz, C.G., Reid, R.I., Przybelski, S.A., Lesnick, T.G., Zuk, S.M., & Jack, C.R. Jr. (2014). White matter integrity determined with diffusion tensor imaging in older adults without dementia: Influence of amyloid load and neurodegeneration. JAMA Neurology, 71(12), 1547–1554. CrossRef Google Scholar
- Kehoe, E.G., Farrell, D., Metzler-Baddeley, C., Lawlor, B.A., Kenny, R.A., Lyons, D., & Bokde, A.L. (2015). Fornix white matter is correlated with resting-state functional connectivity of the thalamus and hippocampus in healthy aging but not in mild cognitive impairment - A preliminary study. Frontiers in Aging Neuroscience, 7, 10. CrossRef Google Scholar
- Kim, H.J., Im, K., Kwon, H., Lee, J.M., Kim, C., Kim, Y.J., & Seo, S.W. (2015). Clinical effect of white matter network disruption related to amyloid and small vessel disease. Neurology, 85(1), 63–70. CrossRef Google Scholar PubMed
- Kljajevic, V., Meyer, P., Holzmann, C., Dyrba, M., Kasper, E., Bokde, A.L., & Teipel, S. (2014). The epsilon4 genotype of apolipoprotein E and white matter integrity in Alzheimer’s disease. Alzheimers & Dementia, 10(3), 401–404. CrossRef Google Scholar PubMed
- Klupp, E., Grimmer, T., Tahmasian, M., Sorg, C., Yakushev, I., Yousefi, B.H., & Forster, S. (2015). Prefrontal hypometabolism in Alzheimer disease is related to longitudinal amyloid accumulation in remote brain regions. Journal of Nuclear Medicine, 56(3), 399–404. CrossRef Google Scholar
- Koch, K., Myers, N.E., Gottler, J., Pasquini, L., Grimmer, T., Forster, S., & Sorg, C. (2014). Disrupted intrinsic networks link amyloid-beta pathology and impaired cognition in prodromal Alzheimer’s disease. Cerebral Cortex. [Epub ahead of print]. Google Scholar
- Krajcovicova, L., Marecek, R., Mikl, M., & Rektorova, I. (2014). Disruption of resting functional connectivity in Alzheimer’s patients and at-risk subjects. Current Neurology and Neuroscience Reports, 14(10), 491. CrossRef Google Scholar PubMed
- Kuczynski, B., Targan, E., Madison, C., Weiner, M., Zhang, Y., Reed, B., & Jagust, W. (2010). White matter integrity and cortical metabolic associations in aging and dementia. Alzheimers & Dementia, 6(1), 54–62. CrossRef Google Scholar PubMed
- La Joie, R., Landeau, B., Perrotin, A., Bejanin, A., Egret, S., Pelerin, A., & Chetelat, G. (2014). Intrinsic connectivity identifies the hippocampus as a main crossroad between Alzheimer’s and semantic dementia-targeted networks. Neuron, 81(6), 1417–1428. CrossRef Google Scholar PubMed
- Laufs, H., Krakow, K., Sterzer, P., Eger, E., Beyerle, A., Salek-Haddadi, A., & Kleinschmidt, A. (2003). Electroencephalographic signatures of attentional and cognitive default modes in spontaneous brain activity fluctuations at rest. Proceedings of the National Academy of Sciences of the United States of America, 100(19), 11053–11058. CrossRef Google Scholar PubMed
- Le Bihan, D., Mangin, J.F., Poupon, C., Clark, C.A., Pappata, S., Molko, N., & Chabriat, H. (2001). Diffusion tensor imaging: Concepts and applications. Journal of Magnetic Resonance Imaging, 13(4), 534–546. CrossRef Google Scholar
- Le Bihan, D., Turner, R., Douek, P., & Patronas, N. (1992). Diffusion MR imaging: Clinical applications. AJR American Journal of Roentgenology, 159(3), 591–599. CrossRef Google Scholar
- Lehmann, M., Madison, C.M., Ghosh, P.M., Seeley, W.W., Mormino, E., Greicius, M.D., & Rabinovici, G.D. (2013). Intrinsic connectivity networks in healthy subjects explain clinical variability in Alzheimer’s disease. Proceedings of the National Academy of Sciences of the United States of America, 110(28), 11606–11611. CrossRef Google Scholar PubMed
- Liang, P., Wang, Z., Yang, Y., Jia, X., & Li, K. (2011). Functional disconnection and compensation in mild cognitive impairment: Evidence from DLPFC connectivity using resting-state fMRI. PLoS One, 6(7), e22153. CrossRef Google Scholar
- Liang, Y., Chen, Y., Li, H., Zhao, T., Sun, X., Shu, N., & Zhang, Z. (2015). Disrupted functional connectivity related to differential degeneration of the cingulum bundle in mild cognitive impairment patients. Current Alzheimer Research, 12(3), 255–265. CrossRef Google Scholar PubMed
- Liang, Y., Li, H., Lv, C., Shu, N., Chen, K., Li, X., & Zhang, Z. (2015). Sex moderates the effects of the Sorl1 gene rs2070045 polymorphism on cognitive impairment and disruption of the cingulum integrity in healthy elderly. Neuropsychopharmacology, 40(6), 1519–1527. CrossRef Google Scholar PubMed
- Liu, Z., Zhang, Y., Yan, H., Bai, L., Dai, R., Wei, W., & Tian, J. (2012). Altered topological patterns of brain networks in mild cognitive impairment and Alzheimer’s disease: A resting-state fMRI study. Psychiatry Research: Neuroimaging, 202(2), 118–125. CrossRef Google Scholar PubMed
- Lo, C.Y., Wang, P.N., Chou, K.H., Wang, J., He, Y., & Lin, C.P. (2010). Diffusion tensor tractography reveals abnormal topological organization in structural cortical networks in Alzheimer’s disease. The Journal of Neuroscience, 30(50), 16876–16885. CrossRef Google Scholar PubMed
- Lu, J., Liu, H., Zhang, M., Wang, D., Cao, Y., Ma, Q., & Li, K. (2011). Focal pontine lesions provide evidence that intrinsic functional connectivity reflects polysynaptic anatomical pathways. The Journal of Neuroscience, 31(42), 15065–15071. CrossRef Google Scholar
- Lyall, D.M., Harris, S.E., Bastin, M.E., Munoz Maniega, S., Murray, C., Lutz, M.W., & Deary, I.J. (2014). Alzheimer’s disease susceptibility genes APOE and TOMM40, and brain white matter integrity in the Lothian Birth Cohort 1936. Neurobiology of Aging, 35(6), 1513 e1525–e1533. CrossRef Google Scholar
- Machulda, M.M., Jones, D.T., Vemuri, P., McDade, E., Avula, R., Przybelski, S., & Jack, C.R. Jr. (2011). Effect of APOE epsilon4 status on intrinsic network connectivity in cognitively normal elderly subjects. Archives of Neurology, 68(9), 1131–1136. CrossRef Google Scholar PubMed
- Mander, B.A., Marks, S.M., Vogel, J.W., Rao, V., Lu, B., Saletin, J.M., & Walker, M.P. (2015). beta-amyloid disrupts human NREM slow waves and related hippocampus-dependent memory consolidation. Nature Neuroscience, 18(7), 1051–1057. CrossRef Google Scholar
- Mantini, D., Perrucci, M.G., Del Gratta, C., Romani, G.L., & Corbetta, M. (2007). Electrophysiological signatures of resting state networks in the human brain. Proceedings of the National Academy of Sciences of the United States of America, 104(32), 13170–13175. CrossRef Google Scholar PubMed
- Mason, M.F., Norton, M.I., Van Horn, J.D., Wegner, D.M., Grafton, S.T., & Macrae, C.N. (2007). Wandering minds: The default network and stimulus-independent thought. Science, 315(5810), 393–395. CrossRef Google Scholar PubMed
- Matura, S., Prvulovic, D., Jurcoane, A., Hartmann, D., Miller, J., Scheibe, M., & Pantel, J. (2014). Differential effects of the ApoE4 genotype on brain structure and function. Neuroimage, 89, 81–91. CrossRef Google Scholar PubMed
- Medina, D., DeToledo-Morrell, L., Urresta, F., Gabrieli, J.D., Moseley, M., Fleischman, D., & Stebbins, G.T. (2006). White matter changes in mild cognitive impairment and AD: A diffusion tensor imaging study. Neurobiology of Aging, 27(5), 663–672. CrossRef Google Scholar
- Mendez, M.F., & Shapira, J.S. (2009). Altered emotional morality in frontotemporal dementia. Cognitive Neuropsychiatry, 14(3), 165–179. CrossRef Google Scholar PubMed
- Menon, V., & Uddin, L.Q. (2010). Saliency, switching, attention and control: A network model of insula function. Brain Structure & Function, 214(5-6), 655–667. CrossRef Google Scholar PubMed
- Metzler-Baddeley, C., Hunt, S., Jones, D.K., Leemans, A., Aggleton, J.P., & O’Sullivan, M.J. (2012). Temporal association tracts and the breakdown of episodic memory in mild cognitive impairment. Neurology, 79(23), 2233–2240. CrossRef Google Scholar PubMed
- Miao, X., Wu, X., Li, R., Chen, K., & Yao, L. (2011). Altered connectivity pattern of hubs in default-mode network with Alzheimer’s disease: An Granger causality modeling approach. PLoS One, 6(10), e25546. CrossRef Google Scholar PubMed
- Mielke, M.M., Okonkwo, O.C., Oishi, K., Mori, S., Tighe, S., Miller, M.I., & Lyketsos, C.G. (2012). Fornix integrity and hippocampal volume predict memory decline and progression to Alzheimer’s disease. Alzheimers & Dementia, 8(2), 105–113. CrossRef Google Scholar PubMed
- Molinuevo, J.L., Ripolles, P., Simo, M., Llado, A., Olives, J., Balasa, M., & Rami, L. (2014). White matter changes in preclinical Alzheimer’s disease: A magnetic resonance imaging-diffusion tensor imaging study on cognitively normal older people with positive amyloid beta protein 42 levels. Neurobiology of Aging, 35(12), 2671–2680. CrossRef Google Scholar
- Moretti, D.V., Paternico, D., Binetti, G., Zanetti, O., & Frisoni, G.B. (2012). EEG markers are associated to gray matter changes in thalamus and basal ganglia in subjects with mild cognitive impairment. Neuroimage, 60(1), 489–496. CrossRef Google Scholar
- Mori, S., Crain, B.J., Chacko, V.P., & Van Zijl, P.C.M. (1999). Three-dimensional tracking of axonal projections in the brain by magnetic resonance imaging. Annals of Neurology, 45(2), 265–269.3.0.CO;2-3>CrossRef Google Scholar
- Mori, S., & Zhang, J. (2006). Principles of diffusion tensor imaging and its applications to basic neuroscience research. Neuron, 51(5), 527–539. CrossRef Google Scholar PubMed
- Mormino, E.C., Smiljic, A., Hayenga, A.O., Onami, S.H., Greicius, M.D., Rabinovici, G.D., & Jagust, W.J. (2011). Relationships between beta-amyloid and functional connectivity in different components of the default mode network in aging. Cerebral Cortex, 21(10), 2399–2407. CrossRef Google Scholar
- Morris, J.C., Roe, C.M., Xiong, C., Fagan, A.M., Goate, A.M., Holtzman, D.M., & Mintun, M.A. (2010). APOE predicts amyloid-beta but not tau Alzheimer pathology in cognitively normal aging. Annals of Neurology, 67(1), 122–131. CrossRef Google Scholar
- Myers, N., Pasquini, L., Gottler, J., Grimmer, T., Koch, K., Ortner, M., & Sorg, C. (2014). Within-patient correspondence of amyloid-beta and intrinsic network connectivity in Alzheimer’s disease. Brain, 137(Pt 7), 2052–2064. CrossRef Google Scholar PubMed
- Naggara, O., Oppenheim, C., Rieu, D., Raoux, N., Rodrigo, S., Dalla Barba, G., & Meder, J.F. (2006). Diffusion tensor imaging in early Alzheimer’s disease. Psychiatry Research, 146(3), 243–249. CrossRef Google Scholar PubMed
- Nath, S., Agholme, L., Kurudenkandy, F.R., Granseth, B., Marcusson, J., & Hallbeck, M. (2012). Spreading of neurodegenerative pathology via neuron-to-neuron transmission of beta-amyloid. The Journal of Neuroscience, 32(26), 8767–8777. CrossRef Google Scholar
- O’Dwyer, L., Lamberton, F., Bokde, A.L., Ewers, M., Faluyi, Y.O., Tanner, C., & Hampel, H. (2012). Using support vector machines with multiple indices of diffusion for automated classification of mild cognitive impairment. PLoS One, 7(2), e32441. CrossRef Google Scholar
- Oh, H., & Jagust, W.J. (2013). Frontotemporal network connectivity during memory encoding is increased with aging and disrupted by beta-amyloid. The Journal of Neuroscience, 33(47), 18425–18437. CrossRef Google Scholar PubMed
- Parihar, M.S., & Brewer, G.J. (2010). Amyloid-beta as a modulator of synaptic plasticity. Journal of Alzheimer’s Disease, 22(3), 741–763. CrossRef Google Scholar PubMed
- Patel, K.T., Stevens, M.C., Pearlson, G.D., Winkler, A.M., Hawkins, K.A., Skudlarski, P., & Bauer, L.O. (2013). Default mode network activity and white matter integrity in healthy middle-aged ApoE4 carriers. Brain Imaging and Behavior, 7(1), 60–67. CrossRef Google Scholar PubMed
- Patriat, R., Molloy, E.K., Meier, T.B., Kirk, G.R., Nair, V.A., Meyerand, M.E., & Birn, R.M. (2013). The effect of resting condition on resting-state fMRI reliability and consistency: A comparison between resting with eyes open, closed, and fixated. Neuroimage, 78, 463–473. CrossRef Google Scholar PubMed
- Perrotin, A., Desgranges, B., Landeau, B., Mezenge, F., La Joie, R., Egret, S., & Chetelat, G. (2015). Anosognosia in Alzheimer disease: Disconnection between memory and self-related brain networks. Annals of Neurology, 78, 477–486. CrossRef Google Scholar
- Persson, J., Lind, J., Larsson, A., Ingvar, M., Sleegers, K., Van Broeckhoven, C., & Nyberg, L. (2008). Altered deactivation in individuals with genetic risk for Alzheimer’s disease. Neuropsychologia, 46(6), 1679–1687. CrossRef Google Scholar PubMed
- Pievani, M., de Haan, W., Wu, T., Seeley, W.W., & Frisoni, G.B. (2011). Functional network disruption in the degenerative dementias. The Lancet. Neurology, 10(9), 829–843. CrossRef Google Scholar
- Puzzo, D., Privitera, L., Leznik, E., Fa, M., Staniszewski, A., Palmeri, A., & Arancio, O. (2008). Picomolar amyloid-beta positively modulates synaptic plasticity and memory in hippocampus. The Journal of Neuroscience, 28(53), 14537–14545. CrossRef Google Scholar
- Racine, A.M., Adluru, N., Alexander, A.L., Christian, B.T., Okonkwo, O.C., Oh, J., & Johnson, S.C. (2014). Associations between white matter microstructure and amyloid burden in preclinical Alzheimer’s disease: A multimodal imaging investigation. Neuroimage. Clinical, 4, 604–614. CrossRef Google Scholar PubMed
- Raichle, M.E., MacLeod, A.M., Snyder, A.Z., Powers, W.J., Gusnard, D.A., & Shulman, G.L. (2001). A default mode of brain function. Proceedings of the National Academy of Sciences of the United States of America, 98(2), 676–682. CrossRef Google Scholar
- Raj, A., Kuceyeski, A., & Weiner, M. (2012). A network diffusion model of disease progression in dementia. Neuron, 73(6), 1204–1215. CrossRef Google Scholar PubMed
- Raj, A., LoCastro, E., Kuceyeski, A., Tosun, D., Relkin, N., & Weiner, M. (2015). Network diffusion model of progression predicts longitudinal patterns of atrophy and metabolism in Alzheimer’s Disease. Cell Reports. [Epub ahead of print]. CrossRef Google Scholar
- Rankin, K.P., Gorno-Tempini, M.L., Allison, S.C., Stanley, C.M., Glenn, S., Weiner, M.W., & Miller, B.L. (2006). Structural anatomy of empathy in neurodegenerative disease. Brain, 129, 2945–2956. CrossRef Google Scholar PubMed
- Ray, N.J., Metzler-Baddeley, C., Khondoker, M.R., Grothe, M.J., Teipel, S., Wright, P., & O’Sullivan, M.J. (2015). Cholinergic Basal forebrain structure influences the reconfiguration of white matter connections to support residual memory in mild cognitive impairment. The Journal of Neuroscience, 35(2), 739–747. CrossRef Google Scholar PubMed
- Reijmer, Y.D., Leemans, A., Caeyenberghs, K., Heringa, S.M., Koek, H.L., & Biessels, G.J. (2013). Disruption of cerebral networks and cognitive impairment in Alzheimer disease. Neurology, 80(15), 1370–1377. CrossRef Google Scholar PubMed
- Reijmer, Y.D., Leemans, A., Heringa, S.M., Wielaard, I., Jeurissen, B., Koek, H.L., & Biessels, G.J. (2012). Improved sensitivity to cerebral white matter abnormalities in Alzheimer’s disease with spherical deconvolution based tractography. PLoS One, 7(8), e44074. CrossRef Google Scholar PubMed
- Ringman, J.M., O’Neill, J., Geschwind, D., Medina, L., Apostolova, L.G., Rodriguez, Y., & Bartzokis, G. (2007). Diffusion tensor imaging in preclinical and presymptomatic carriers of familial Alzheimer’s disease mutations. Brain, 130(Pt 7), 1767–1776. CrossRef Google Scholar
- Rubinov, M., & Sporns, O. (2010). Complex network measures of brain connectivity: Uses and interpretations. Neuroimage, 52(3), 1059–1069. CrossRef Google Scholar
- Ryan, N.S., Keihaninejad, S., Shakespeare, T.J., Lehmann, M., Crutch, S.J., Malone, I.B., & Fox, N.C. (2013). Magnetic resonance imaging evidence for presymptomatic change in thalamus and caudate in familial Alzheimer’s disease. Brain, 136(Pt 5), 1399–1414. CrossRef Google Scholar PubMed
- Sadaghiani, S., Scheeringa, R., Lehongre, K., Morillon, B., Giraud, A.L., & Kleinschmidt, A. (2010). Intrinsic connectivity networks, alpha oscillations, and tonic alertness: A simultaneous electroencephalography/functional magnetic resonance imaging study. The Journal of Neuroscience, 30(30), 10243–10250. CrossRef Google Scholar PubMed
- Salami, A., Pudas, S., & Nyberg, L. (2014). Elevated hippocampal resting-state connectivity underlies deficient neurocognitive function in aging. Proceedings of the National Academy of Sciences of the United States of America, 111(49), 17654–17659. CrossRef Google Scholar
- Sanz-Arigita, E.J., Schoonheim, M.M., Damoiseaux, J.S., Rombouts, S.A., Maris, E., Barkhof, F., & Stam, C.J. (2010). Loss of ‘small-world’ networks in Alzheimer’s disease: Graph analysis of FMRI resting-state functional connectivity. PLoS One, 5(11), e13788. CrossRef Google Scholar PubMed
- Scola, E., Bozzali, M., Agosta, F., Magnani, G., Franceschi, M., Sormani, M.P., & Falini, A. (2010). A diffusion tensor MRI study of patients with MCI and AD with a 2-year clinical follow-up. Journal of Neurology, Neurosurgery and Psychiatry, 81(7), 798–805. CrossRef Google Scholar
- Seeley, W.W., Allman, J.M., Carlin, D.A., Crawford, R.K., Macedo, M.N., Greicius, M.D., & Miller, B.L. (2007). Divergent social functioning in behavioral variant frontotemporal dementia and Alzheimer disease: Reciprocal networks and neuronal evolution. Alzheimer Disease and Associated Disorders, 21(4), S50–S57. CrossRef Google Scholar
- Seeley, W.W., Crawford, R.K., Zhou, J., Miller, B.L., & Greicius, M.D. (2009). Neurodegenerative diseases target large-scale human brain networks. Neuron, 62(1), 42–52. CrossRef Google Scholar
- Selnes, P., Aarsland, D., Bjornerud, A., Gjerstad, L., Wallin, A., Hessen, E., & Fladby, T. (2013). Diffusion tensor imaging surpasses cerebrospinal fluid as predictor of cognitive decline and medial temporal lobe atrophy in subjective cognitive impairment and mild cognitive impairment. Journal of Alzheimer’s Disease, 33(3), 723–736. Google Scholar PubMed
- Sepulcre, J., Sabuncu, M.R., Becker, A., Sperling, R., & Johnson, K.A. (2013). In vivo characterization of the early states of the amyloid-beta network. Brain, 136(Pt 7), 2239–2252. CrossRef Google Scholar
- Sepulcre, J., Sabuncu, M.R., Yeo, T.B., Liu, H., & Johnson, K.A. (2012). Stepwise connectivity of the modal cortex reveals the multimodal organization of the human brain. The Journal of Neuroscience, 32(31), 10649–10661. CrossRef Google Scholar
- Sexton, C.E., Mackay, C.E., Lonie, J.A., Bastin, M.E., Terriere, E., O’Carroll, R.E., & Ebmeier, K.P. (2010). MRI correlates of episodic memory in Alzheimer’s disease, mild cognitive impairment, and healthy aging. Psychiatry Research, 184(1), 57–62. CrossRef Google Scholar PubMed
- Shankar, G.M., Bloodgood, B.L., Townsend, M., Walsh, D.M., Selkoe, D.J., & Sabatini, B.L. (2007). Natural oligomers of the Alzheimer amyloid-beta protein induce reversible synapse loss by modulating an NMDA-type glutamate receptor-dependent signaling pathway. The Journal of Neuroscience, 27(11), 2866–2875. CrossRef Google Scholar PubMed
- Shao, J., Myers, N., Yang, Q., Feng, J., Plant, C., Bohm, C., & Sorg, C. (2012). Prediction of Alzheimer’s disease using individual structural connectivity networks. Neurobiology of Aging, 33(12), 2756–2765. CrossRef Google Scholar PubMed
- Sheline, Y.I., Morris, J.C., Snyder, A.Z., Price, J.L., Yan, Z., D’Angelo, G., & Mintun, M.A. (2010). APOE4 allele disrupts resting state fMRI connectivity in the absence of amyloid plaques or decreased CSF Abeta42. The Journal of Neuroscience, 30(50), 17035–17040. CrossRef Google Scholar PubMed
- Sheline, Y.I., Raichle, M.E., Snyder, A.Z., Morris, J.C., Head, D., Wang, S., & Mintun, M.A. (2010). Amyloid plaques disrupt resting state default mode network connectivity in cognitively normal elderly. Biological Psychiatry, 67(6), 584–587. CrossRef Google Scholar
- Shulman, G.L., Corbetta, M., Fiez, J.A., Buckner, R.L., Miezin, F.M., Raichle, M.E., & Petersen, S.E. (1997). Searching for activations that generalize over tasks. Human Brain Mapping, 5(4), 317–322.3.0.CO;2-A>CrossRef Google Scholar
- Smith, S.M., Beckmann, C.F., Andersson, J., Auerbach, E.J., Bijsterbosch, J., Douaud, G., & Glasser, M.F. (2013). Resting-state fMRI in the Human Connectome Project. Neuroimage, 80, 144–168. CrossRef Google Scholar PubMed
- Smith, S.M., Fox, P.T., Miller, K.L., Glahn, D.C., Fox, P.M., Mackay, C.E., & Beckmann, C.F. (2009). Correspondence of the brain’s functional architecture during activation and rest. Proceedings of the National Academy of Sciences of the United States of America, 106(31), 13040–13045. CrossRef Google Scholar
- Soldner, J., Meindl, T., Koch, W., Bokde, A.L., Reiser, M.F., Moller, H.J., & Teipel, S.J. (2012). [Structural and functional neuronal connectivity in Alzheimer’s disease: A combined DTI and fMRI study]. Der Nervenarzt, 83(7), 878–887. CrossRef Google Scholar
- Sollberger, M., Stanley, C.M., Wilson, S.M., Gyurak, A., Beckman, V., Growdon, M., & Rankin, K.P. (2009). Neural basis of interpersonal traits in neurodegenerative diseases. Neuropsychologia, 47(13), 2812–2827. CrossRef Google Scholar
- Song, S.K., Sun, S.W., Ju, W.K., Lin, S.J., Cross, A.H., & Neufeld, A.H. (2003). Diffusion tensor imaging detects and differentiates axon and myelin degeneration in mouse optic nerve after retinal ischemia. Neuroimage, 20(3), 1714–1722. CrossRef Google Scholar PubMed
- Sorg, C., Riedl, V., Muhlau, M., Calhoun, V.D., Eichele, T., Laer, L., & Wohlschlager, A.M. (2007). Selective changes of resting-state networks in individuals at risk for Alzheimer’s disease. Proceedings of the National Academy of Sciences of the United States of America, 104(47), 18760–18765. CrossRef Google Scholar
- Sperling, R.A., Laviolette, P.S., O’Keefe, K., O’Brien, J., Rentz, D.M., Pihlajamaki, M., & Johnson, K.A. (2009). Amyloid deposition is associated with impaired default network function in older persons without dementia. Neuron, 63(2), 178–188. CrossRef Google Scholar PubMed
- Spires-Jones, T.L., & Hyman, B.T. (2014). The intersection of amyloid beta and tau at synapses in Alzheimer’s disease. Neuron, 82(4), 756–771. CrossRef Google Scholar PubMed
- Sporns, O., Honey, C.J., & Kotter, R. (2007). Identification and classification of hubs in brain networks. PLoS One, 2(10), e1049. CrossRef Google Scholar PubMed
- Stahl, R., Dietrich, O., Teipel, S.J., Hampel, H., Reiser, M.F., & Schoenberg, S.O. (2007). White matter damage in Alzheimer disease and mild cognitive impairment: Assessment with diffusion-tensor MR imaging and parallel imaging techniques. Radiology, 243(2), 483–492. CrossRef Google Scholar
- Stenset, V., Bjornerud, A., Fjell, A.M., Walhovd, K.B., Hofoss, D., Due-Tonnessen, P., & Fladby, T. (2011). Cingulum fiber diffusivity and CSF T-tau in patients with subjective and mild cognitive impairment. Neurobiology of Aging, 32(4), 581–589. CrossRef Google Scholar PubMed
- Sturm, V.E., McCarthy, M.E., Yun, I., Madan, A., Yuan, J.W., Holley, S.R., & Levenson, R.W. (2011). Mutual gaze in Alzheimer’s disease, frontotemporal and semantic dementia couples. Social Cognitive and Affective Neuroscience, 6(3), 359–367. CrossRef Google Scholar PubMed
- Sturm, V.E., Yokoyama, J.S., Seeley, W.W., Kramer, J.H., Miller, B.L., & Rankin, K.P. (2013). Heightened emotional contagion in mild cognitive impairment and Alzheimer’s disease is associated with temporal lobe degeneration. Proceedings of the National Academy of Sciences of the United States of America, 110(24), 9944–9949. CrossRef Google Scholar PubMed
- Supekar, K., Menon, V., Rubin, D., Musen, M., & Greicius, M.D. (2008). Network analysis of intrinsic functional brain connectivity in Alzheimer’s disease. PLoS Computational Biology, 4(6), e1000100. CrossRef Google Scholar
- Tahmasian, M., Pasquini, L., Scherr, M., Meng, C., Forster, S., Mulej Bratec, S., & Drzezga, A. (2015). The lower hippocampus global connectivity, the higher its local metabolism in Alzheimer disease. Neurology, 84(19), 1956–1963. CrossRef Google Scholar
- Teipel, S., Ehlers, I., Erbe, A., Holzmann, C., Lau, E., Hauenstein, K., & Berger, C. (2015). Structural connectivity changes underlying altered working memory networks in mild cognitive impairment: A three-way image fusion analysis. J Neuroimaging, 25(4), 634–642. CrossRef Google Scholar PubMed
- Teipel, S.J., Bokde, A.L., Meindl, T., Amaro, E. Jr., Soldner, J., Reiser, M.F., & Hampel, H. (2010). White matter microstructure underlying default mode network connectivity in the human brain. Neuroimage, 49(3), 2021–2032. CrossRef Google Scholar PubMed
- Teipel, S.J., Grothe, M.J., Filippi, M., Fellgiebel, A., Dyrba, M., Frisoni, G.B., & group, E.S. (2014). Fractional anisotropy changes in Alzheimer’s disease depend on the underlying fiber tract architecture: A multiparametric DTI study using joint independent component analysis. Journal of Alzheimer’s Disease, 41(1), 69–83. Google Scholar PubMed
- Teipel, S.J., Lerche, M., Kilimann, I., O’Brien, K., Grothe, M., Meyer, P., & Hauenstein, K. (2014). Decline of fiber tract integrity over the adult age range: A diffusion spectrum imaging study. Journal of Magnetic Resonance Imaging, 40(2), 348–359. CrossRef Google Scholar PubMed
- Teipel, S.J., Meindl, T., Grinberg, L., Grothe, M., Cantero, J.L., Reiser, M.F., & Hampel, H. (2011). The cholinergic system in mild cognitive impairment and Alzheimer’s disease: An in vivo MRI and DTI study. Human Brain Mapping, 32(9), 1349–1362. CrossRef Google Scholar
- Teipel, S.J., Meindl, T., Wagner, M., Stieltjes, B., Reuter, S., Hauenstein, K.H., & Hampel, H. (2010). Longitudinal changes in fiber tract integrity in healthy aging and mild cognitive impairment: A DTI follow-up study. Journal of Alzheimer’s Disease, 22(2), 507–522. CrossRef Google Scholar
- Teipel, S.J., Pogarell, O., Meindl, T., Dietrich, O., Sydykova, D., Hunklinger, U., & Hampel, H. (2009). Regional networks underlying interhemispheric connectivity: An EEG and DTI study in healthy ageing and amnestic mild cognitive impairment. Human Brain Mapping, 30(7), 2098–2119. CrossRef Google Scholar PubMed
- Teipel, S.J., Reuter, S., Stieltjes, B., Acosta-Cabronero, J., Ernemann, U., Fellgiebel, A., & Hampel, H. (2011). Multicenter stability of diffusion tensor imaging measures: A European clinical and physical phantom study. Psychiatry Research, 194(3), 363–371. CrossRef Google Scholar PubMed
- Teipel, S.J., Sabri, O., Grothe, M., Barthel, H., Prvulovic, D., Buerger, K., & Hampel, H. (2013). Perspectives for multimodal neurochemical and imaging biomarkers in Alzheimer’s disease. Journal of Alzheimer’s Disease, 33(Suppl. 1), S329–S347. Google Scholar
- Teipel, S.J., Wegrzyn, M., Meindl, T., Frisoni, G., Bokde, A.L., Fellgiebel, A., & Ewers, M. (2012). Anatomical MRI and DTI in the diagnosis of Alzheimer’s disease: A European multicenter study. Journal of Alzheimer’s Disease, 31(Suppl. 3), S33–S47. Google Scholar
- Thal, D.R., Attems, J., & Ewers, M. (2014). Spreading of amyloid, tau, and microvascular pathology in Alzheimer’s disease: Findings from neuropathological and neuroimaging studies. Journal of Alzheimer’s Disease, 42(Suppl. 4), S421–S429. Google Scholar
- van den Heuvel, M., Mandl, R., Luigjes, J., & Hulshoff Pol, H. (2008). Microstructural organization of the cingulum tract and the level of default mode functional connectivity. The Journal of Neuroscience, 28(43), 10844–10851. CrossRef Google Scholar PubMed
- van den Heuvel, M.P., Mandl, R.C., Kahn, R.S., & Hulshoff Pol, H.E. (2009). Functionally linked resting-state networks reflect the underlying structural connectivity architecture of the human brain. Human Brain Mapping, 30(10), 3127–3141. CrossRef Google Scholar
- van den Heuvel, M.P., & Sporns, O. (2011). Rich-club organization of the human connectome. Journal of Neuroscience, 31(44), 15775–15786. CrossRef Google Scholar
- Vecchio, F., Miraglia, F., Curcio, G., Altavilla, R., Scrascia, F., Giambattistelli, F., & Rossini, P.M. (2015). Cortical brain connectivity evaluated by graph theory in dementia: A correlation study between functional and structural data. Journal of Alzheimer’s Disease, 45, 745–756. Google Scholar
- Vidal-Pineiro, D., Valls-Pedret, C., Fernandez-Cabello, S., Arenaza-Urquijo, E.M., Sala-Llonch, R., Solana, E., & Bartres-Faz, D. (2014). Decreased default mode network connectivity correlates with age-associated structural and cognitive changes. Frontiers in Aging Neuroscience, 6, 256. Google Scholar PubMed
- Villain, N., Fouquet, M., Baron, J.C., Mezenge, F., Landeau, B., de La Sayette, V., & Chetelat, G. (2010). Sequential relationships between grey matter and white matter atrophy and brain metabolic abnormalities in early Alzheimer’s disease. Brain, 133(11), 3301–3314. CrossRef Google Scholar PubMed
- Villemagne, V.L., Pike, K.E., Chetelat, G., Ellis, K.A., Mulligan, R.S., Bourgeat, P., & Rowe, C.C. (2011). Longitudinal assessment of Abeta and cognition in aging and Alzheimer disease. Annals of Neurology, 69(1), 181–192. CrossRef Google Scholar PubMed
- Vincent, J.L., Patel, G.H., Fox, M.D., Snyder, A.Z., Baker, J.T., Van Essen, D.C., & Raichle, M.E. (2007). Intrinsic functional architecture in the anaesthetized monkey brain. Nature, 447(7140), 83–86. CrossRef Google Scholar PubMed
- Voineskos, A.N., Lerch, J.P., Felsky, D., Shaikh, S., Rajji, T.K., Miranda, D., & Kennedy, J.L. (2011). The brain-derived neurotrophic factor Val66Met polymorphism and prediction of neural risk for Alzheimer disease. Archives of General Psychiatry, 68(2), 198–206. CrossRef Google Scholar PubMed
- Wang, D., Liu, B., Qin, W., Wang, J., Zhang, Y., Jiang, T., & Yu, C. (2013). KIBRA gene variants are associated with synchronization within the default-mode and executive control networks. Neuroimage, 69, 213–222. CrossRef Google Scholar PubMed
- Wang, J., Wang, X., He, Y., Yu, X., Wang, H., & He, Y. (2015). Apolipoprotein E epsilon4 modulates functional brain connectome in Alzheimer’s disease. Human Brain Mapping, 36, 1828–1846. CrossRef Google Scholar PubMed
- Wang, K., Liang, M., Wang, L., Tian, L., Zhang, X., Li, K., & Jiang, T. (2007). Altered functional connectivity in early Alzheimer’s disease: A resting-state fMRI study. Human Brain Mapping, 28(10), 967–978. CrossRef Google Scholar PubMed
- Wedeen, V.J., Wang, R.P., Schmahmann, J.D., Benner, T., Tseng, W.Y., Dai, G., & de Crespigny, A.J. (2008). Diffusion spectrum magnetic resonance imaging (DSI) tractography of crossing fibers. Neuroimage, 41(4), 1267–1277. CrossRef Google Scholar PubMed
- Wee, C.Y., Yap, P.T., Zhang, D., Denny, K., Browndyke, J.N., Potter, G.G., & Shen, D. (2012). Identification of MCI individuals using structural and functional connectivity networks. Neuroimage, 59(3), 2045–2056. CrossRef Google Scholar PubMed
- Weiler, M., de Campos, B.M., Nogueira, M.H., Pereira Damasceno, B., Cendes, F., & Balthazar, M.L. (2014). Structural connectivity of the default mode network and cognition in Alzheimers disease. Psychiatry Research, 223(1), 15–22. CrossRef Google Scholar PubMed
- Westlye, L.T., Reinvang, I., Rootwelt, H., & Espeseth, T. (2012). Effects of APOE on brain white matter microstructure in healthy adults. Neurology, 79(19), 1961–1969. CrossRef Google Scholar PubMed
- Whitwell, J.L., Przybelski, S.A., Weigand, S.D., Knopman, D.S., Boeve, B.F., Petersen, R.C., & Jack, C.R. Jr. (2007). 3D maps from multiple MRI illustrate changing atrophy patterns as subjects progress from mild cognitive impairment to Alzheimer’s disease. Brain, 130(Pt 7), 1777–1786. CrossRef Google Scholar PubMed
- Xie, S., Xiao, J.X., Gong, G.L., Zang, Y.F., Wang, Y.H., Wu, H.K., & Jiang, X.X. (2006). Voxel-based detection of white matter abnormalities in mild Alzheimer disease. Neurology, 66(12), 1845–1849. CrossRef Google Scholar
- Xiong, C., Roe, C.M., Buckles, V., Fagan, A., Holtzman, D., Balota, D., & Morris, J.C. (2011). Role of family history for Alzheimer biomarker abnormalities in the adult children study. Archives of Neurology, 68(10), 1313–1319. CrossRef Google Scholar PubMed
- Yakushev, I., Schreckenberger, M., Muller, M.J., Schermuly, I., Cumming, P., Stoeter, P., & Fellgiebel, A. (2011). Functional implications of hippocampal degeneration in early Alzheimer’s disease: A combined DTI and PET study. European Journal of Nuclear Medicine and Molecular Imaging, 38(12), 2219–2227. CrossRef Google Scholar PubMed
- Yeo, B.T., Krienen, F.M., Sepulcre, J., Sabuncu, M.R., Lashkari, D., Hollinshead, M., & Buckner, R.L. (2011). The organization of the human cerebral cortex estimated by intrinsic functional connectivity. Journal of Neurophysiology, 106(3), 1125–1165. Google Scholar PubMed
- Zhang, H.Y., Wang, S.J., Liu, B., Ma, Z.L., Yang, M., Zhang, Z.J., & Teng, G.J. (2010). Resting brain connectivity: Changes during the progress of Alzheimer disease. Radiology, 256(2), 598–606. CrossRef Google Scholar PubMed
- Zhang, Y., Schuff, N., Jahng, G.H., Bayne, W., Mori, S., Schad, L., & Weiner, M.W. (2007). Diffusion tensor imaging of cingulum fibers in mild cognitive impairment and Alzheimer disease. Neurology, 68(1), 13–19. CrossRef Google Scholar PubMed
- Zhou, J., Gennatas, E.D., Kramer, J.H., Miller, B.L., & Seeley, W.W. (2012). Predicting regional neurodegeneration from the healthy brain functional connectome. Neuron, 73(6), 1216–1227. CrossRef Google Scholar
- Zhou, J., Greicius, M.D., Gennatas, E.D., Growdon, M.E., Jang, J.Y., Rabinovici, G.D., & Seeley, W.W. (2010). Divergent network connectivity changes in behavioural variant frontotemporal dementia and Alzheimer’s disease. Brain, 133(Pt 5), 1352–1367. CrossRef Google Scholar
- Zhuang, L., Sachdev, P.S., Trollor, J.N., Kochan, N.A., Reppermund, S., Brodaty, H., & Wen, W. (2012). Microstructural white matter changes in cognitively normal individuals at risk of amnestic MCI. Neurology, 79(8), 748–754. CrossRef Google Scholar PubMed
- Zuo, X.N., Ehmke, R., Mennes, M., Imperati, D., Castellanos, F.X., Sporns, O., & Milham, M.P. (2012). Network centrality in the human functional connectome. Cerebral Cortex, 22(8), 1862–1875. CrossRef Google Scholar PubMed
Clinical neuroscience is increasingly turning to imaging the human brain for answers to a range of questions and challenges. To date, the majority of studies have focused on the neural basis of current psychiatric symptoms, which can facilitate the identification of neurobiological markers for diagnosis. However, the increasing availability and feasibility of using imaging modalities, such as diffusion imaging and resting-state fMRI, enable longitudinal mapping of brain development. This shift in the field is opening the possibility of identifying predictive markers of risk or prognosis, and also represents a critical missing element for efforts to promote personalized or individualized medicine in psychiatry (i.e., stratified psychiatry).
The present work provides a selective review of potentially high-yield populations for longitudinal examination with MRI, based upon our understanding of risk from epidemiologic studies and initial MRI findings.
Our discussion is organized into three topic areas: (1) practical considerations for establishing temporal precedence in psychiatric research; (2) readiness of the field for conducting longitudinal MRI, particularly for neurodevelopmental questions; and (3) illustrations of high-yield populations and time windows for examination that can be used to rapidly generate meaningful and useful data. Particular emphasis is placed on the implementation of time-appropriate, developmentally informed longitudinal designs, capable of facilitating the identification of biomarkers predictive of risk and prognosis.
Strategic longitudinal examination of the brain at-risk has the potential to bring the concepts of early intervention and prevention to psychiatry. (JINS, 2016,22, 164–179)
- Aarnoudse-Moens, C.S., Weisglas-Kuperus, N., van Goudoever, J.B., & Oosterlaan, J. (2009). Meta-analysis of neurobehavioral outcomes in very preterm and/or very low birth weight children. Pediatrics, 124(2), 717–728. doi:10.1542/peds.2008-2816 CrossRef Google Scholar PubMed
- Alexander, A.L., Hurley, S.A., Samsonov, A.A., Adluru, N., Hosseinbor, A.P., Mossahebi, P.,& Field, A.S. (2011). Characterization of cerebral white matter properties using quantitative magnetic resonance imaging stains. Brain Connectivity, 1(6), 423–446. doi:10.1089/brain.2011.0071 CrossRef Google Scholar PubMed
- Allin, M., Walshe, M., Fern, A., Nosarti, C., Cuddy, M., Rifkin, L., & Wyatt, J. (2008). Cognitive maturation in preterm and term born adolescents. Journal of Neurology, Neurosurgery, & Psychiatry, 79(4), 381–386. doi:10.1136/jnnp.2006.110858 CrossRef Google Scholar
- Almli, C.R., Rivkin, M.J., & McKinstry, R.C., & Brain Development Cooperative, Group. (2007). The NIH MRI study of normal brain development (Objective-2): Newborns, infants, toddlers, and preschoolers. Neuroimage, 35(1), 308–325. doi:10.1016/j.neuroimage.2006.08.058 CrossRef Google Scholar
- Ameis, S.H., & Catani, M. (2015). Altered white matter connectivity as a neural substrate for social impairment in Autism Spectrum Disorder. Cortex, 62, 158–181. doi:10.1016/j.cortex.2014.10.014 CrossRef Google Scholar PubMed
- Anderson, A.L., & Thomason, M.E. (2013). Functional plasticity before the cradle: A review of neural functional imaging in the human fetus. Neuroscience & Biobehavioral Reviews, 37(9 Pt B), 2220–2232. doi:10.1016/j.neubiorev.2013.03.013 CrossRef Google Scholar
- Anderson, D.K., Lord, C., Risi, S., DiLavore, P.S., Shulman, C., Thurm, A., & Pickles, A. (2007). Patterns of growth in verbal abilities among children with autism spectrum disorder. Journal of Consulting and Clinical Psychology, 75(4), 594–604. doi:10.1037/0022-006X.75.4.594 CrossRef Google Scholar PubMed
- Aylward, E.H., Richards, T.L., Berninger, V.W., Nagy, W.E., Field, K.M., Grimme, A.C., & Cramer, S.C. (2003). Instructional treatment associated with changes in brain activation in children with dyslexia. Neurology, 61(2), 212–219. CrossRef Google Scholar PubMed
- Baghdadli, A., Picot, M.C., Michelon, C., Bodet, J., Pernon, E., Burstezjn, C., & Aussilloux, C. (2007). What happens to children with PDD when they grow up? Prospective follow-up of 219 children from preschool age to mid-childhood. Acta Psychiatrica Scandinavica, 115(5), 403–412. doi:10.1111/j.1600-0447.2006.00898.x CrossRef Google Scholar
- Bal, V.H., Kim, S.H., Cheong, D., & Lord, C. (2015). Daily living skills in individuals with autism spectrum disorder from 2 to 21 years of age. Autism, 19(7), 774–784. doi:10.1177/1362361315575840 CrossRef Google Scholar PubMed
- Barquero, L.A., Davis, N., & Cutting, L.E. (2014). Neuroimaging of reading intervention: A systematic review and activation likelihood estimate meta-analysis. PLoS One, 9(1), e83668. doi:10.1371/journal.pone.0083668 CrossRef Google Scholar PubMed
- Barre, N., Morgan, A., Doyle, L.W., & Anderson, P.J. (2011). Language abilities in children who were very preterm and/or very low birth weight: A meta-analysis. Journal of Pediatrics, 158(5), 766–774 e761. doi:10.1016/j.jpeds.2010.10.032 CrossRef Google Scholar PubMed
- Barttfeld, P., Uhrig, L., Sitt, J.D., Sigman, M., Jarraya, B., & Dehaene, S. (2015). Signature of consciousness in the dynamics of resting-state brain activity. Proceedings of the National Academy of Sciences of the United States of America, 112(3), 887–892. doi:10.1073/pnas.1418031112 CrossRef Google Scholar PubMed
- Bartzokis, G. (2004). Quadratic trajectories of brain myelin content: Unifying construct for neuropsychiatric disorders. Neurobiology of Aging, 25(1), 49–62. doi:http://dx.doi.org/10.1016/j.neurobiolaging.2003.08.001 CrossRef Google Scholar
- Basser, P.J., & Pierpaoli, C. (1996). Microstructural and physiological features of tissues elucidated by quantitative-diffusion-tensor MRI. Journal of Magnetic Resonance, Series B, 111(3), 209–219. CrossRef Google Scholar
- Beesdo, K., Pine, D.S., Lieb, R., & Wittchen, H.U. (2010). Incidence and risk patterns of anxiety and depressive disorders and categorization of generalized anxiety disorder. Archives of General Psychiatry, 67(1), 47–57. doi:10.1001/archgenpsychiatry.2009.177 CrossRef Google Scholar PubMed
- Behrman, R.E., & Butler, A.S. (2007). Preterm birth: Causes, consequences, and prevention. Washington, DC: National Academies Press. Google Scholar
- Billstedt, E., Gillberg, I.C., & Gillberg, C. (2005). Autism after adolescence: Population-based 13- to 22-year follow-up study of 120 individuals with autism diagnosed in childhood. Journal of Autism and Developmental Disorders, 35(3), 351–360. CrossRef Google Scholar PubMed
- Blasi, A., Lloyd-Fox, S., Sethna, V., Brammer, M.J., Mercure, E., Murray, L., & Johnson, M.H. (2015). Atypical processing of voice sounds in infants at risk for autism spectrum disorder. Cortex, 71, 122–133. doi:10.1016/j.cortex.2015.06.015 CrossRef Google Scholar PubMed
- Bora, E., Harrison, B.J., Davey, C.G., Yucel, M., & Pantelis, C. (2012). Meta-analysis of volumetric abnormalities in cortico-striatal-pallidal-thalamic circuits in major depressive disorder. Psychological Medicine, 42(4), 671–681. doi:10.1017/S0033291711001668 CrossRef Google Scholar PubMed
- Botteron, K.N., Raichle, M.E., Drevets, W.C., Heath, A.C., & Todd, R.D. (2002). Volumetric reduction in left subgenual prefrontal cortex in early onset depression. Biological Psychiatry, 51(4), 342–344. CrossRef Google Scholar PubMed
- Bremner, J.D. (2004). Brain imaging in anxiety disorders. Expert Review of Neurotherapeutics, 4(2), 275–284. doi:10.1586/14737175.4.2.275 CrossRef Google Scholar PubMed
- Brown, C.J., Miller, S.P., Booth, B.G., Andrews, S., Chau, V., Poskitt, K.J., & Hamarneh, G. (2014). Structural network analysis of brain development in young preterm neonates. Neuroimage, 101, 667–680. doi:10.1016/j.neuroimage.2014.07.030 CrossRef Google Scholar PubMed
- Brown, I.S., & Felton, R.H. (1999). Effects of instruction on beginning reading skills in children at risk for reading disability. Reading and Writing: An Interdisciplinary Journal, 2, 223–241. CrossRef Google Scholar
- Bruhl, A.B., Delsignore, A., Komossa, K., & Weidt, S. (2014). Neuroimaging in social anxiety disorder-a meta-analytic review resulting in a new neurofunctional model. Neuroscience and Biobehavioral Reviews, 47, 260–280. doi:10.1016/j.neubiorev.2014.08.003 CrossRef Google Scholar
- Buckholtz, J.W., & Meyer-Lindenberg, A. (2012). Psychopathology and the human connectome: Toward a transdiagnostic model of risk for mental illness. Neuron, 74(6), 990–1004. doi:10.1016/j.neuron.2012.06.002 CrossRef Google Scholar
- Burmeister, M., McInnis, M.G., & Zollner, S. (2008). Psychiatric genetics: Progress amid controversy. Nature Reviews. Genetics, 9(7), 527–540. doi:10.1038/nrg2381 CrossRef Google Scholar PubMed
- Buss, C., Davis, E.P., Shahbaba, B., Pruessner, J.C., Head, K., & Sandman, C.A. (2012). Maternal cortisol over the course of pregnancy and subsequent child amygdala and hippocampus volumes and affective problems. Proceedings of the National Academy of Sciences of the United States of America, 109(20), E1312–E1319. doi:10.1073/pnas.1201295109 CrossRef Google Scholar PubMed
- Buss, C., Entringer, S., Swanson, J.M., & Wadhwa, P.D. (2012). The role of stress in brain development: The gestational environment’s long-term effects on the brain. Cerebrum, 2012, 4. Google Scholar
- Carballedo, A., Scheuerecker, J., Meisenzahl, E., Schoepf, V., Bokde, A., Moller, H.J., & Frodl, T. (2011). Functional connectivity of emotional processing in depression. Journal of Affective Disorders, 134(1–3), 272–279. doi:10.1016/j.jad.2011.06.021 CrossRef Google Scholar PubMed
- Carmody, D.P., Bendersky, M., Dunn, S.M., DeMarco, J.K., Hegyi, T., Hiatt, M., & Lewis, M. (2006). Early risk, attention, and brain activation in adolescents born preterm. Child Development, 77(2), 384–394. doi:10.1111/j.1467-8624.2006.00877.x CrossRef Google Scholar PubMed
- Caspi, A., & Moffitt, T.E. (2006). Gene-environment interactions in psychiatry: Joining forces with neuroscience. Nature Reviews Neuroscience, 7(7), 583–590. doi:10.1038/nrn1925 CrossRef Google Scholar PubMed
- Castellanos, F.X., Di Martino, A., Craddock, R.C., Mehta, A.D., & Milham, M.P. (2013). Clinical applications of the functional connectome. Neuroimage, 80, 527–540. doi:10.1016/j.neuroimage.2013.04.083 CrossRef Google Scholar PubMed
- Colvert, E., Tick, B., McEwen, F., Stewart, C., Curran, S.R., Woodhouse, E., & Bolton, P. (2015). Heritability of Autism Spectrum Disorder in a UK Population-Based Twin Sample. The Journal of the American Medical Association, Psychiatry, 72(5), 415–423. doi:10.1001/jamapsychiatry.2014.3028 Google Scholar
- Constable, R.T., Ment, L.R., Vohr, B.R., Kesler, S.R., Fulbright, R.K., Lacadie, C., & Reiss, A.R. (2008). Prematurely born children demonstrate white matter microstructural differences at 12 years of age, relative to term control subjects: An investigation of group and gender effects. Pediatrics, 121(2), 306–316. doi:10.1542/peds.2007-0414 CrossRef Google Scholar PubMed
- Costa e Silva, J.A. (2013). Personalized medicine in psychiatry: New technologies and approaches. Metabolism, 62(Suppl. 1), S40–S44. doi:10.1016/j.metabol.2012.08.017 CrossRef Google Scholar
- Counsell, S.J., Edwards, A.D., Chew, A.T., Anjari, M., Dyet, L.E., Srinivasan, L., & Cowan, F.M. (2008). Specific relations between neurodevelopmental abilities and white matter microstructure in children born preterm. Brain, 131(Pt 12), 3201–3208. doi:10.1093/brain/awn268 CrossRef Google Scholar PubMed
- Cross-Disorder Group of the Psychiatric Genomics, C., Lee, S.H., Ripke, S., Neale, B.M., Faraone, S.V., Purcell, S.M., … Wray, N.R. (2013). Genetic relationship between five psychiatric disorders estimated from genome-wide SNPs. Nature Genetics, 45(9), 984–994. doi:10.1038/ng.2711 CrossRef Google Scholar PubMed
- Damaraju, E., Phillips, J.R., Lowe, J.R., Ohls, R., Calhoun, V.D., & Caprihan, A. (2010). Resting-state functional connectivity differences in premature children. Frontiers in Systems Neuroscience, 4, pii:23. doi:10.3389/fnsys.2010.00023 Google Scholar PubMed
- Davis, E.P., & Sandman, C.A. (2010). The timing of prenatal exposure to maternal cortisol and psychosocial stress is associated with human infant cognitive development. Child Development, 81(1), 131–148. doi:10.1111/j.1467-8624.2009.01385.x CrossRef Google Scholar PubMed
- Dawson, G., Ashman, S.B., & Carver, L.J. (2000). The role of early experience in shaping behavioral and brain development and its implications for social policy. Development and Psychopathology, 12(4), 695–712. CrossRef Google Scholar
- Degnan, A.J., Wisnowski, J.L., Choi, S., Ceschin, R., Bhushan, C., Leahy, R.M., & Panigrahy, A. (2015). Altered structural and functional connectivity in late preterm preadolescence: An anatomic seed-based study of resting state networks related to the posteromedial and lateral parietal cortex. PLoS One, 10(6), e0130686. doi:10.1371/journal.pone.0130686 CrossRef Google Scholar
- Deoni, S.C., Dean, D.C. III, Remer, J., Dirks, H., & O’Muircheartaigh, J. (2015). Cortical maturation and myelination in healthy toddlers and young children. Neuroimage, 115, 147–161. doi:10.1016/j.neuroimage.2015.04.058 CrossRef Google Scholar PubMed
- Di Martino, A., Fair, D.A., Kelly, C., Satterthwaite, T.D., Castellanos, F.X., Thomason, M.E., & Milham, M.P. (2014). Unraveling the miswired connectome: A developmental perspective. Neuron, 83(6), 1335–1353. doi:10.1016/j.neuron.2014.08.050 CrossRef Google Scholar PubMed
- Di Martino, A., Yan, C.G., Li, Q., Denio, E., Castellanos, F.X., Alaerts, K., & Milham, M.P. (2014). The autism brain imaging data exchange: Towards a large-scale evaluation of the intrinsic brain architecture in autism. Molecular Psychiatry, 19(6), 659–667. doi:10.1038/mp.2013.78 CrossRef Google Scholar PubMed
- Drevets, W.C., Savitz, J., & Trimble, M. (2008). The subgenual anterior cingulate cortex in mood disorders. CNS Spectrums, 13(8), 663–681. CrossRef Google Scholar PubMed
- Dubois, J., Dehaene-Lambertz, G., Kulikova, S., Poupon, C., Huppi, P.S., & Hertz-Pannier, L. (2014). The early development of brain white matter: A review of imaging studies in fetuses, newborns and infants. Neuroscience, 276, 48–71. doi:10.1016/j.neuroscience.2013.12.044 CrossRef Google Scholar
- Duerden, E.G., Card, D., Lax, I.D., Donner, E.J., & Taylor, M.J. (2013). Alterations in frontostriatal pathways in children born very preterm. Developmental Medicine and Child Neurology, 55(10), 952–958. doi:10.1111/dmcn.12198 CrossRef Google Scholar PubMed
- Eckert, M.A., Leonard, C.M., Richards, T.L., Aylward, E.H., Thomson, J., & Berninger, V.W. (2003). Anatomical correlates of dyslexia: Frontal and cerebellar findings. Brain, 126(Pt 2), 482–494. CrossRef Google Scholar PubMed
- Eden, G.F., Jones, K.M., Cappell, K., Gareau, L., Wood, F.B., Zeffiro, T.A., & Flowers, D.L. (2004). Neural changes following remediation in adult developmental dyslexia. Neuron, 44(3), 411–422. doi:10.1016/j.neuron.2004.10.019 CrossRef Google Scholar PubMed
- Elison, J.T., Paterson, S.J., Wolff, J.J., Reznick, J.S., Sasson, N.J., Gu, H., & Network, I. (2013). White matter microstructure and atypical visual orienting in 7-month-olds at risk for autism. The American Journal of Psychiatry, 170(8), 899–908. doi:10.1176/appi.ajp.2012.12091150 CrossRef Google Scholar PubMed
- Eriksen, H.L., Kesmodel, U.S., Pedersen, L.H., & Mortensen, E.L. (2015). No association between prenatal exposure to psychotropics and intelligence at age five. Acta Obstetricia et Gynecologica Scandinavica, 94(5), 501–507. doi:10.1111/aogs.12611 CrossRef Google Scholar PubMed
- Etkin, A., & Wager, T.D. (2007). Functional neuroimaging of anxiety: A meta-analysis of emotional processing in PTSD, social anxiety disorder, and specific phobia. The American Journal of Psychiatry, 164(10), 1476–1488. doi:10.1176/appi.ajp.2007.07030504 CrossRef Google Scholar PubMed
- Fair, D.A., Posner, J., Nagel, B.J., Bathula, D., Dias, T.G., Mills, K.L., & Nigg, J.T. (2010). Atypical default network connectivity in youth with attention-deficit/hyperactivity disorder. Biological Psychiatry, 68(12), 1084–1091. doi:10.1016/j.biopsych.2010.07.003 CrossRef Google Scholar PubMed
- Feldman, H.M., Lee, E.S., Yeatman, J.D., & Yeom, K.W. (2012). Language and reading skills in school-aged children and adolescents born preterm are associated with white matter properties on diffusion tensor imaging. Neuropsychologia, 50(14), 3348–3362. doi:10.1016/j.neuropsychologia.2012.10.014 CrossRef Google Scholar PubMed
- Ferrazzi, G., Kuklisova Murgasova, M., Arichi, T., Malamateniou, C., Fox, M.J., Makropoulos, A., & Hajnal, J.V. (2014). Resting State fMRI in the moving fetus: A robust framework for motion, bias field and spin history correction. Neuroimage, 101, 555–568. doi:10.1016/j.neuroimage.2014.06.074 CrossRef Google Scholar PubMed
- Finke, K., Neitzel, J., Bauml, J.G., Redel, P., Muller, H.J., Meng, C., & Sorg, C. (2015). Visual attention in preterm born adults: Specifically impaired attentional sub-mechanisms that link with altered intrinsic brain networks in a compensation-like mode. Neuroimage, 107, 95–106. doi:10.1016/j.neuroimage.2014.11.062 CrossRef Google Scholar
- Finn, E.S., Shen, X., Scheinost, D., Rosenberg, M.D., Huang, J., Chun, M.M., & Constable, R.T. (2015). Functional connectome fingerprinting: Identifying individuals using patterns of brain connectivity. Nature Neuroscience, 18, 1664–1671. doi:10.1038/nn.4135 CrossRef Google Scholar PubMed
- Foland-Ross, L.C., Gilbert, B.L., Joormann, J., & Gotlib, I.H. (2015). Neural markers of familial risk for depression: An investigation of cortical thickness abnormalities in healthy adolescent daughters of mothers with recurrent depression. Journal of Abnormal Psychology, 124(3), 476–485. doi:10.1037/abn0000050 CrossRef Google Scholar PubMed
- Foland-Ross, L.C., Hardin, M.G., & Gotlib, I.H. (2013). Neurobiological markers of familial risk for depression. Current Topics in Behavioral Neurosciences, 14, 181–206. 10.1007/7854_2012_213 CrossRef Google Scholar PubMed
- Foland-Ross, L.C., Sacchet, M.D., Prasad, G., Gilbert, B., Thompson, P.M., & Gotlib, I.H. (2015). Cortical thickness predicts the first onset of major depression in adolescence. International Journal of Developmental Neuroscience, 46, 125–131. doi:10.1016/j.ijdevneu.2015.07.007 CrossRef Google Scholar
- Frye, R.E., Malmberg, B., Desouza, L., Swank, P., Smith, K., & Landry, S. (2009). Increased prefrontal activation in adolescents born prematurely at high risk during a reading task. Brain Research, 1303, 111–119. doi:10.1016/j.brainres.2009.09.091 CrossRef Google Scholar PubMed
- Fukunaga, M., Horovitz, S.G., van Gelderen, P., de Zwart, J.A., Jansma, J.M., Ikonomidou, V.N., & Duyn, J.H. (2006). Large-amplitude, spatially correlated fluctuations in BOLD fMRI signals during extended rest and early sleep stages. Magnetic Resonance Imaging, 24(8), 979–992. doi:10.1016/j.mri.2006.04.018 CrossRef Google Scholar PubMed
- Gao, W., Alcauter, S., Elton, A., Hernandez-Castillo, C.R., Smith, J.K., Ramirez, J., & Lin, W. (2015). Functional network development during the first year: Relative sequence and socioeconomic correlations. Cerebral Cortex, 25(9), 2919–2928. doi:10.1093/cercor/bhu088 CrossRef Google Scholar PubMed
- Gaugler, T., Klei, L., Sanders, S.J., Bodea, C.A., Goldberg, A.P., Lee, A.B., & Buxbaum, J.D. (2014). Most genetic risk for autism resides with common variation. Nature Genetics, 46(8), 881–885. doi:10.1038/ng.3039 CrossRef Google Scholar PubMed
- Georgiades, S., Szatmari, P., Boyle, M., Hanna, S., Duku, E., & Zwaigenbaum, L., … Pathways in ASD Study Team. (2013). Investigating phenotypic heterogeneity in children with autism spectrum disorder: A factor mixture modeling approach. Journal of Child Psychology and Psychiatry, 54(2), 206–215. doi:10.1111/j.1469-7610.2012.02588.x CrossRef Google Scholar PubMed
- Germano, E., Gagliano, A., & Curatolo, P. (2010). Comorbidity of ADHD and dyslexia. Developmental Neuropsychology, 35(5), 475–493. doi:10.1080/87565641.2010.494748 CrossRef Google Scholar
- Geschwind, D.H. (2009). Advances in autism. Annual Review of Medicine, 60, 367–380. doi:10.1146/annurev.med.60.053107.121225 CrossRef Google Scholar PubMed
- Geschwind, N. (1965a). Disconnexion syndromes in animals and man. I. Brain, 88(2), 237–294. CrossRef Google Scholar PubMed
- Geschwind, N. (1965b). Disconnexion syndromes in animals and man. II. Brain, 88(3), 585–644. CrossRef Google Scholar PubMed
- Glass, H.C., Costarino, A.T., Stayer, S.A., Brett, C.M., Cladis, F., & Davis, P.J. (2015). Outcomes for extremely premature infants. Anesthesia and Analgesia, 120(6), 1337–1351. doi:10.1213/ANE.0000000000000705 CrossRef Google Scholar PubMed
- Glover, V. (2014). Maternal depression, anxiety and stress during pregnancy and child outcome; what needs to be done. Best Practice and Research: Clinical Obstetrics and Gynaecology, 28(1), 25–35. doi:10.1016/j.bpobgyn.2013.08.017 CrossRef Google Scholar
- Gooch, D., Hulme, C., Nash, H.M., & Snowling, M.J. (2014). Comorbidities in preschool children at family risk of dyslexia. Journal of Child Psychology and Psychiatry, 55(3), 237–246. doi:10.1111/jcpp.12139 CrossRef Google Scholar PubMed
- Hastings, R.S., Parsey, R.V., Oquendo, M.A., Arango, V., & Mann, J.J. (2004). Volumetric analysis of the prefrontal cortex, amygdala, and hippocampus in major depression. Neuropsychopharmacology, 29(5), 952–959. doi:10.1038/sj.npp.1300371 CrossRef Google Scholar PubMed
- Hay, D.F., Pawlby, S., Waters, C.S., Perra, O., & Sharp, D. (2010). Mothers’ antenatal depression and their children’s antisocial outcomes. Child Development, 81(1), 149–165. doi:10.1111/j.1467-8624.2009.01386.x CrossRef Google Scholar PubMed
- Hill, R.M., Pettit, J.W., Lewinsohn, P.M., Seeley, J.R., & Klein, D.N. (2014). Escalation to major depressive disorder among adolescents with subthreshold depressive symptoms: Evidence of distinct subgroups at risk. Journal of Affective Disorders, 158, 133–138. doi:10.1016/j.jad.2014.02.011 CrossRef Google Scholar PubMed
- Ho, T.C., Yang, G., Wu, J., Cassey, P., Brown, S.D., Hoang, N., & Yang, T.T. (2014). Functional connectivity of negative emotional processing in adolescent depression. Journal of Affective Disorders, 155, 65–74. doi:10.1016/j.jad.2013.10.025 CrossRef Google Scholar PubMed
- Hoeft, F., McCandliss, B.D., Black, J.M., Gantman, A., Zakerani, N., Hulme, C., & Gabrieli, J.D. (2011). Neural systems predicting long-term outcome in dyslexia. Proceedings of the National Academy of Sciences of the United States of America, 108(1), 361–366. doi:10.1073/pnas.1008950108 CrossRef Google Scholar PubMed
- Holmes, A.J., Hollinshead, M.O., O’Keefe, T.M., Petrov, V.I., Fariello, G.R., Wald, L.L., & Buckner, R.L. (2015). Brain Genomics Superstruct Project initial data release with structural, functional, and behavioral measures. Scientific Data, 2, 150031. doi:10.1038/sdata.2015.31 CrossRef Google Scholar
- Howlin, P., Goode, S., Hutton, J., & Rutter, M. (2004). Adult outcome for children with autism. Journal of Child Psychology and Psychiatry, 45(2), 212–229. CrossRef Google Scholar PubMed
- Huizink, A.C., de Medina, P.G., Mulder, E.J., Visser, G.H., & Buitelaar, J.K. (2002). Psychological measures of prenatal stress as predictors of infant temperament. Journal of the American Academy of Child and Adolescent Psychiatry, 41(9), 1078–1085. CrossRef Google Scholar PubMed
- Jakab, A., Kasprian, G., Schwartz, E., Gruber, G.M., Mitter, C., Prayer, D., & Langs, G. (2015). Disrupted developmental organization of the structural connectome in fetuses with corpus callosum agenesis. Neuroimage, 111, 277–288. doi:10.1016/j.neuroimage.2015.02.038 CrossRef Google Scholar PubMed
- Jakab, A., Schwartz, E., Kasprian, G., Gruber, G.M., Prayer, D., Schopf, V., & Langs, G. (2014). Fetal functional imaging portrays heterogeneous development of emerging human brain networks. Frontiers in Human Neuroscience, 8, 852. doi:10.3389/fnhum.2014.00852 CrossRef Google Scholar
- Jernigan, T.L., Brown, T.T., Hagler, D.J. Jr., Akshoomoff, N., Bartsch, H., & Newman, E., … Pediatric Imaging Neurocognition and Genetics Study. (2015). The Pediatric Imaging, Neurocognition, and Genetics (PING) data repository. Neuroimage, 124, 1149–1154. doi:10.1016/j.neuroimage.2015.04.057 CrossRef Google Scholar PubMed
- Jeste, S.S., & Geschwind, D.H. (2014). Disentangling the heterogeneity of autism spectrum disorder through genetic findings. Nature Reviews. Neurology, 10(2), 74–81. doi:10.1038/nrneurol.2013.278 CrossRef Google Scholar PubMed
- Johnson, S., & Marlow, N. (2011). Preterm birth and childhood psychiatric disorders. Pediatric Research, 69(5, Part 2 of 2), 11R–18R. CrossRef Google Scholar PubMed
- Jovicich, J., Marizzoni, M., Bosch, B., Bartres-Faz, D., Arnold, J., & Benninghoff, J., … PharmaCog, Consortium. (2014). Multisite longitudinal reliability of tract-based spatial statistics in diffusion tensor imaging of healthy elderly subjects. Neuroimage, 101, 390–403. doi:10.1016/j.neuroimage.2014.06.075 CrossRef Google Scholar PubMed
- Kaiser, M. (2013). The potential of the human connectome as a biomarker of brain disease. Frontier in Human Neuroscience, 7, 484. doi: 10.3389/fnhum.2013.00484 Google Scholar PubMed
- Kaiser, R.H., Andrews-Hanna, J.R., Wager, T.D., & Pizzagalli, D.A. (2015). Large-scale network dysfunction in major depressive disorder: A Meta-analysis of resting-state functional connectivity. Journal of the American Medical Association Psychiatry, 72(6), 603–611. doi:10.1001/jamapsychiatry.2015.0071 Google Scholar PubMed
- Kapur, S., Phillips, A.G., & Insel, T.R. (2012). Why has it taken so long for biological psychiatry to develop clinical tests and what to do about it? Molecular Psychiatry, 17(12), 1174–1179. doi:10.1038/mp.2012.105 CrossRef Google Scholar
- Kasprian, G., Langs, G., Brugger, P.C., Bittner, M., Weber, M., Arantes, M., & Prayer, D. (2011). The prenatal origin of hemispheric asymmetry: An in utero neuroimaging study. Cerebral Cortex, 21(5), 1076–1083. doi:10.1093/cercor/bhq179 CrossRef Google Scholar
- Kelly, C., Biswal, B.B., Craddock, R.C., Castellanos, F.X., & Milham, M.P. (2012). Characterizing variation in the functional connectome: Promise and pitfalls. Trends in Cognitive Science, 16(3), 181–188. doi:10.1016/j.tics.2012.02.001 CrossRef Google Scholar PubMed
- Kersbergen, K.J., Leemans, A., Groenendaal, F., van der Aa, N.E., Viergever, M.A., de Vries, L.S., & Benders, M.J. (2014). Microstructural brain development between 30 and 40 weeks corrected age in a longitudinal cohort of extremely preterm infants. Neuroimage, 103, 214–224. doi:10.1016/j.neuroimage.2014.09.039 CrossRef Google Scholar
- Kessler, R.C., Avenevoli, S., & Merikangas, K.R. (2001). Mood disorders in children and adolescents: An epidemiologic perspective. Biological Psychiatry, 49(12), 1002–1014. CrossRef Google Scholar
- Kingston, D., Tough, S., & Whitfield, H. (2012). Prenatal and postpartum maternal psychological distress and infant development: A systematic review. Child Psychiatry and Human Development, 43(5), 683–714. doi:10.1007/s10578-012-0291-4 CrossRef Google Scholar PubMed
- Koyama, M.S., Di Martino, A., Kelly, C., Jutagir, D.R., Sunshine, J., Schwartz, S.J., & Milham, M.P. (2013). Cortical signatures of dyslexia and remediation: An intrinsic functional connectivity approach. PLoS One, 8(2), e55454. 10.1371/journal.pone.0055454 CrossRef Google Scholar PubMed
- Krogsrud, S.K., Fjell, A.M., Tamnes, C.K., Grydeland, H., Mork, L., Due-Tonnessen, P., & Walhovd, K.B. (2015). Changes in white matter microstructure in the developing brain-A longitudinal diffusion tensor imaging study of children from 4 to 11years of age. Neuroimage, 124(Pt A), 473–486. doi:10.1016/j.neuroimage.2015.09.017 CrossRef Google Scholar PubMed
- Kuhn, T., Gullett, J.M., Nguyen, P., Boutzoukas, A.E., Ford, A., Colon-Perez, L.M., & Bauer, R.M. (2015). Test-retest reliability of high angular resolution diffusion imaging acquisition within medial temporal lobe connections assessed via tract based spatial statistics, probabilistic tractography and a novel graph theory metric. Brain Imaging and Behavior. doi:10.1007/s11682-015-9425-1 Google Scholar
- Kwon, S.H., Vasung, L., Ment, L.R., & Huppi, P.S. (2014). The role of neuroimaging in predicting neurodevelopmental outcomes of preterm neonates. Clinics in Perinatology, 41(1), 257–283. doi:10.1016/j.clp.2013.10.003 CrossRef Google Scholar PubMed
- Limperopoulos, C., & Clouchoux, C. (2009). Advancing fetal brain MRI: Targets for the future. Seminars in Perinatology, 33(4), 289–298. doi:10.1053/j.semperi.2009.04.002 CrossRef Google Scholar PubMed
- Liston, C., Chen, A.C., Zebley, B.D., Drysdale, A.T., Gordon, R., & Leuchter, B., Dubin, M.J. (2014). Default mode network mechanisms of transcranial magnetic stimulation in depression. Biological Psychiatry, 76(7), 517–526. doi:10.1016/j.biopsych.2014.01.023 CrossRef Google Scholar PubMed
- Liu, J., Glenn, O.A., & Xu, D. (2014). Fast, free-breathing, in vivo fetal imaging using time-resolved 3D MRI technique: Preliminary results. Quantitative Imaging Medicine and Surgery, 4(2), 123–128. doi:10.3978/j.issn.2223-4292.2014.04.08 Google Scholar
- Loeffler, M., Engel, C., Ahnert, P., Alfermann, D., Arelin, K., Baber, R., & Thiery, J. (2015). The LIFE-Adult-Study: Objectives and design of a population-based cohort study with 10,000 deeply phenotyped adults in Germany. BioMed Central Public Health, 15, 691. doi:10.1186/s12889-015-1983-z CrossRef Google Scholar PubMed
- Lombardo, M.V., Pierce, K., Eyler, L.T., Barnes, C.C., Ahrens-Barbeau, C., Solso, S., & Courchesne, E. (2015). Different functional neural substrates for good and poor language outcome in autism. Neuron, 86(2), 567–577. CrossRef Google Scholar PubMed
- Lubsen, J., Vohr, B., Myers, E., Hampson, M., Lacadie, C., Schneider, K.C., & Ment, L.R. (2011). Microstructural and functional connectivity in the developing preterm brain. Seminars in Perinatology, 35(1), 34–43. doi:10.1053/j.semperi.2010.10.006 CrossRef Google Scholar
- Martin, J., O’Donovan, M.C., Thapar, A., Langley, K., & Williams, N. (2015). The relative contribution of common and rare genetic variants to ADHD. Translational Psychiatry, 5, e506. doi:10.1038/tp.2015.5 CrossRef Google Scholar
- Matson, J.L., & Williams, L.W. (2013). Differential diagnosis and comorbidity: Distinguishing autism from other mental health issues. Neuropsychiatry, 3(2), 233–243. doi:10.2217/npy.13.1 CrossRef Google Scholar
- McArthur, G., Kohnen, S., Larsen, L., Jones, K., Anandakumar, T., Banales, E., & Castles, A. (2013). Getting to grips with the heterogeneity of developmental dyslexia. Cognitive Neuropsychology, 30(1), 1–24. doi:10.1080/02643294.2013.784192 CrossRef Google Scholar PubMed
- McGrath, C.L., Kelley, M.E., Dunlop, B.W., Holtzheimer, P.E. III, Craighead, W.E., & Mayberg, H.S. (2014). Pretreatment brain states identify likely nonresponse to standard treatments for depression. Biological Psychiatry, 76(7), 527–535. doi:10.1016/j.biopsych.2013.12.005 CrossRef Google Scholar
- Mennes, M., Biswal, B.B., Castellanos, F.X., & Milham, M.P. (2013). Making data sharing work: The FCP/INDI experience. Neuroimage, 82, 683–691. doi:10.1016/j.neuroimage.2012.10.064 CrossRef Google Scholar PubMed
- Ment, L.R., Hirtz, D., & Huppi, P.S. (2009). Imaging biomarkers of outcome in the developing preterm brain. Lancet Neurology, 8(11), 1042–1055. doi:10.1016/S1474-4422(09)70257-1 CrossRef Google Scholar
- Ment, L.R., Kesler, S., Vohr, B., Katz, K.H., Baumgartner, H., Schneider, K.C., & Reiss, A.L. (2009). Longitudinal brain volume changes in preterm and term control subjects during late childhood and adolescence. Journal of Pediatrics, 123(2), 503–511. doi:10.1542/peds.2008-0025 CrossRef Google Scholar PubMed
- Milham, M.P., Nugent, A.C., Drevets, W.C., Dickstein, D.P., Leibenluft, E., Ernst, M., & Pine, D.S. (2005). Selective reduction in amygdala volume in pediatric anxiety disorders: A voxel-based morphometry investigation. Biological Psychiatry, 57(9), 961–966. doi:10.1016/j.biopsych.2005.01.038 CrossRef Google Scholar
- Miller, C.H., Hamilton, J.P., Sacchet, M.D., & Gotlib, I.H. (2015). Meta-analysis of functional neuroimaging of major depressive disorder in youth. Journal of the American Medical Association Psychiatry, 72(10), 1045–1053. doi:10.1001/jamapsychiatry.2015.1376 Google Scholar PubMed
- Monk, C., Spicer, J., & Champagne, F.A. (2012). Linking prenatal maternal adversity to developmental outcomes in infants: The role of epigenetic pathways. Development and Psychopathology, 24(4), 1361–1376. doi:10.1017/S0954579412000764 CrossRef Google Scholar PubMed
- Mueller, S., Wang, D., Fox, M.D., Pan, R., Lu, J., Li, K., & Liu, H. (2015). Reliability correction for functional connectivity: Theory and implementation. Human Brain Mapping, 36(11), 4664–4680. doi:10.1002/hbm.22947 CrossRef Google Scholar PubMed
- Muhle, R., Trentacoste, S.V., & Rapin, I. (2004). The genetics of autism. Journal of Pediatrics, 113(5), e472–e486. CrossRef Google Scholar PubMed
- Mullen, K.M., Vohr, B.R., Katz, K.H., Schneider, K.C., Lacadie, C., Hampson, M., & Ment, L.R. (2011). Preterm birth results in alterations in neural connectivity at age 16 years. Neuroimage, 54(4), 2563–2570. doi:10.1016/j.neuroimage.2010.11.019 CrossRef Google Scholar PubMed
- Myers, E.H., Hampson, M., Vohr, B., Lacadie, C., Frost, S.J., Pugh, K.R., & Ment, L.R. (2010). Functional connectivity to a right hemisphere language center in prematurely born adolescents. Neuroimage, 51(4), 1445–1452. doi:10.1016/j.neuroimage.2010.03.049 CrossRef Google Scholar PubMed
- NessAiver, A., NessAiver, M., Harms, M., & Xu, J. (2015). FBIRN-X: An updated fBIRN quality assurance protocol for slice accelerated fMRI. Retrieved from https://ww4.aievolution.com/hbm1501/files/content/abstracts/44138/1686_NessAiver.pdf. Google Scholar
- Nooner, K.B., Colcombe, S.J., Tobe, R.H., Mennes, M., Benedict, M.M., Moreno, A.L., & Milham, M.P. (2012). The NKI-Rockland Sample: A model for accelerating the pace of discovery science in psychiatry. Frontiers in Neuroscience, 6, 152. doi:10.3389/fnins.2012.00152 CrossRef Google Scholar
- O’Connor, T.G., Monk, C., & Fitelson, E.M. (2014). Practitioner review: Maternal mood in pregnancy and child development--implications for child psychology and psychiatry. Journal of Child Psychology and Psychiatry, 55(2), 99–111. doi:10.1111/jcpp.12153 CrossRef Google Scholar PubMed
- Odegard, T.N., Ring, J., Smith, S., Biggan, J., & Black, J. (2008). Differentiating the neural response to intervention in children with developmental dyslexia. Annals of Dyslexia, 58(1), 1–14. doi:10.1007/s11881-008-0014-5 CrossRef Google Scholar PubMed
- Odsbu, I., Skurtveit, S., Selmer, R., Roth, C., Hernandez-Diaz, S., & Handal, M. (2015). Prenatal exposure to anxiolytics and hypnotics and language competence at 3 years of age. European Journal Clinical Pharmacology, 71(3), 283–291. doi:10.1007/s00228-014-1797-4 CrossRef Google Scholar PubMed
- Ornoy, A., & Koren, G. (2014). Selective serotonin reuptake inhibitors in human pregnancy: On the way to resolving the controversy. Seminars in Fetal and Neonatal Medicine, 19(3), 188–194. doi:10.1016/j.siny.2013.11.007 CrossRef Google Scholar
- Ozonoff, S., Young, G.S., Carter, A., Messinger, D., Yirmiya, N., Zwaigenbaum, L., & Stone, W.L. (2011). Recurrence risk for autism spectrum disorders: A Baby Siblings Research Consortium study. Journal of Pediatrics, 128(3), e488–e495. doi:10.1542/peds.2010-2825 Google Scholar PubMed
- Perez-Edgar, K., & Fox, N.A. (2005). Temperament and anxiety disorders. Child and Adolescent Psychiatric Clinics of North America, 14(4), 681–706, viii. doi:10.1016/j.chc.2005.05.008 CrossRef Google Scholar PubMed
- Petzoldt, J., Wittchen, H.U., Wittich, J., Einsle, F., Hofler, M., & Martini, J. (2014). Maternal anxiety disorders predict excessive infant crying: A prospective longitudinal study. Archives of Disease in Childhood, 99(9), 800–806. doi:10.1136/archdischild-2013-305562 CrossRef Google Scholar PubMed
- Pezawas, L., Meyer-Lindenberg, A., Drabant, E.M., Verchinski, B.A., Munoz, K.E., Kolachana, B.S., & Weinberger, D.R. (2005). 5-HTTLPR polymorphism impacts human cingulate-amygdala interactions: A genetic susceptibility mechanism for depression. Nature Neuroscience, 8(6), 828–834. doi:10.1038/nn1463 CrossRef Google Scholar
- Pickles, A., Anderson, D.K., & Lord, C. (2014). Heterogeneity and plasticity in the development of language: A 17-year follow-up of children referred early for possible autism. Journal of Child Psychology and Psychiatry, 55(12), 1354–1362. doi:10.1111/jcpp.12269 CrossRef Google Scholar PubMed
- Pine, D.S., Cohen, E., Cohen, P., & Brook, J. (1999). Adolescent depressive symptoms as predictors of adult depression: Moodiness or mood disorder? American Journal of Psychiatry, 156(1), 133–135. CrossRef Google Scholar PubMed
- Pine, D.S., Cohen, P., Gurley, D., Brook, J., & Ma, Y. (1998). The risk for early-adulthood anxiety and depressive disorders in adolescents with anxiety and depressive disorders. Archives of General Psychiatry, 55(1), 56–64. CrossRef Google Scholar PubMed
- Power, J.D., Barnes, K.A., Snyder, A.Z., Schlaggar, B.L., & Petersen, S.E. (2012). Spurious but systematic correlations in functional connectivity MRI networks arise from subject motion. Neuroimage, 59(3), 2142–2154. doi:10.1016/j.neuroimage.2011.10.018 CrossRef Google Scholar PubMed
- Price, J.L., & Drevets, W.C. (2012). Neural circuits underlying the pathophysiology of mood disorders. Trends in Cognitive Sciences, 16(1), 61–71. doi:10.1016/j.tics.2011.12.011 CrossRef Google Scholar PubMed
- Qin, S., Young, C.B., Duan, X., Chen, T., Supekar, K., & Menon, V. (2014). Amygdala subregional structure and intrinsic functional connectivity predicts individual differences in anxiety during early childhood. Biological Psychiatry, 75(11), 892–900. doi:10.1016/j.biopsych.2013.10.006 CrossRef Google Scholar PubMed
- Qiu, A., Rifkin-Graboi, A., Chen, H., Chong, Y.S., Kwek, K., Gluckman, P.D., & Meaney, M.J. (2013). Maternal anxiety and infants’ hippocampal development: Timing matters. Translational Psychiatry, 3, e306. doi:10.1038/tp.2013.79 CrossRef Google Scholar PubMed
- Radua, J., Via, E., Catani, M., & Mataix-Cols, D. (2011). Voxel-based meta-analysis of regional white-matter volume differences in autism spectrum disorder versus healthy controls. Psychological Medicine, 41(7), 1539–1550. doi:10.1017/S0033291710002187 CrossRef Google Scholar PubMed
- Rafii, M.S., Wishnek, H., Brewer, J.B., Donohue, M.C., Ness, S., Mobley, W.C., & Rissman, R.A. (2015). The down syndrome biomarker initiative (DSBI) pilot: Proof of concept for deep phenotyping of Alzheimer’s disease biomarkers in down syndrome. Frontiers in Behavioral Neuroscience, 9, 239. doi:10.3389/fnbeh.2015.00239 CrossRef Google Scholar PubMed
- Ramenghi, L.A., Rutherford, M., Fumagalli, M., Bassi, L., Messner, H., Counsell, S., & Mosca, F. (2009). Neonatal neuroimaging: Going beyond the pictures. Early Human Development, 85(10 Suppl.), S75–S77. doi:10.1016/j.earlhumdev.2009.08.022 CrossRef Google Scholar
- Ramus, F., Rosen, S., Dakin, S.C., Day, B.L., Castellote, J.M., White, S., & Frith, U. (2003). Theories of developmental dyslexia: Insights from a multiple case study of dyslexic adults. Brain, 126(Pt 4), 841–865. CrossRef Google Scholar PubMed
- Raschle, N.M., Chang, M., & Gaab, N. (2011). Structural brain alterations associated with dyslexia predate reading onset. Neuroimage, 57(3), 742–749. doi:10.1016/j.neuroimage.2010.09.055 CrossRef Google Scholar PubMed
- Raschle, N.M., Stering, P.L., Meissner, S.N., & Gaab, N. (2014). Altered neuronal response during rapid auditory processing and its relation to phonological processing in prereading children at familial risk for dyslexia. Cerebral Cortex, 24(9), 2489–2501. doi:10.1093/cercor/bht104 CrossRef Google Scholar PubMed
- Ray, R.D., & Zald, D.H. (2012). Anatomical insights into the interaction of emotion and cognition in the prefrontal cortex. Neuroscience and Biobehavioral Reviews, 36(1), 479–501. doi:10.1016/j.neubiorev.2011.08.005 CrossRef Google Scholar
- Reddy, U.M., Abuhamad, A.Z., Levine, D., Saade, G.R., & Fetal Imaging Workshop Invited Paraticipants. (2014). Fetal imaging: Executive summary of a Joint Eunice Kennedy Shriver National Institute of Child Health and Human Development, Society for Maternal-Fetal Medicine, American Institute of Ultrasound in Medicine, American College of Obstetricians and Gynecologists, American College of Radiology, Society for Pediatric Radiology, and Society of Radiologists in Ultrasound Fetal Imaging Workshop. American Journal of Obstetrics and Gynecology, 210(5), 387–397. doi:10.1016/j.ajog.2014.02.028 CrossRef Google Scholar
- Richlan, F., Kronbichler, M., & Wimmer, H. (2011). Meta-analyzing brain dysfunctions in dyslexic children and adults. Neuroimage, 56(3), 1735–1742. doi:10.1016/j.neuroimage.2011.02.040 CrossRef Google Scholar PubMed
- Richlan, F., Kronbichler, M., & Wimmer, H. (2013). Structural abnormalities in the dyslexic brain: A meta-analysis of voxel-based morphometry studies. Human Brain Mapping, 34(11), 3055–3065. doi:10.1002/hbm.22127 CrossRef Google Scholar PubMed
- Rifkin-Graboi, A., Bai, J., Chen, H., Hameed, W.B., Sim, L.W., Tint, M.T., & Qiu, A. (2013). Prenatal maternal depression associates with microstructure of right amygdala in neonates at birth. Biological Psychiatry, 74(11), 837–844. doi:10.1016/j.biopsych.2013.06.019 CrossRef Google Scholar PubMed
- Robinson, O.J., Krimsky, M., Lieberman, L., Allen, P., Vytal, K., & Grillon, C. (2014). Towards a mechanistic understanding of pathological anxiety: The dorsal medial prefrontal-amygdala ‘aversive amplification’ circuit in unmedicated generalized and social anxiety disorders. Lancet Psychiatry, 1(4), 294–302. doi:10.1016/S2215-0366(14)70305-0 CrossRef Google Scholar
- Rose, J., Butler, E.E., Lamont, L.E., Barnes, P.D., Atlas, S.W., & Stevenson, D.K. (2009). Neonatal brain structure on MRI and diffusion tensor imaging, sex, and neurodevelopment in very-low-birthweight preterm children. Developmental Medicine and Child Neurology, 51(7), 526–535. doi:10.1111/j.1469-8749.2008.03231.x CrossRef Google Scholar
- Roy, A.K., Fudge, J.L., Kelly, C., Perry, J.S., Daniele, T., Carlisi, C., & Ernst, M. (2013). Intrinsic functional connectivity of amygdala-based networks in adolescent generalized anxiety disorder. Journal of the American Academy of Child and Adolescent Psychiatry, 52(3), 290–299 e292. doi:10.1016/j.jaac.2012.12.010 CrossRef Google Scholar PubMed
- Roy, A.K., Shehzad, Z., Margulies, D.S., Kelly, A.M., Uddin, L.Q., Gotimer, K., & Milham, M.P. (2009). Functional connectivity of the human amygdala using resting state fMRI. Neuroimage, 45(2), 614–626. doi:10.1016/j.neuroimage.2008.11.030 CrossRef Google Scholar PubMed
- Sadeghi, N., Prastawa, M., Fletcher, P.T., Wolff, J., Gilmore, J.H., & Gerig, G. (2013). Regional characterization of longitudinal DT-MRI to study white matter maturation of the early developing brain. Neuroimage, 68, 236–247. doi:10.1016/j.neuroimage.2012.11.040 CrossRef Google Scholar
- Saleem, S.N. (2014). Fetal MRI: An approach to practice: A review. Journal of Advanced Research, 5(5), 507–523. doi:10.1016/j.jare.2013.06.001 CrossRef Google Scholar
- Sandin, S., Lichtenstein, P., Kuja-Halkola, R., Larsson, H., Hultman, C.M., & Reichenberg, A. (2014). The familial risk of autism. Journal of the American Medical Association, 311(17), 1770–1777. doi:10.1001/jama.2014.4144 CrossRef Google Scholar PubMed
- Sandman, C.A., Buss, C., Head, K., & Davis, E.P. (2015). Fetal exposure to maternal depressive symptoms is associated with cortical thickness in late childhood. Biological Psychiatry, 77(4), 324–334. doi:10.1016/j.biopsych.2014.06.025 CrossRef Google Scholar PubMed
- Satterthwaite, T.D., Elliott, M.A., Gerraty, R.T., Ruparel, K., Loughead, J., Calkins, M.E., & Wolf, D.H. (2013). An improved framework for confound regression and filtering for control of motion artifact in the preprocessing of resting-state functional connectivity data. Neuroimage, 64, 240–256. doi:10.1016/j.neuroimage.2012.08.052 CrossRef Google Scholar PubMed
- Saygin, Z.M., Norton, E.S., Osher, D.E., Beach, S.D., Cyr, A.B., Ozernov-Palchik, O., & Gabrieli, J.D. (2013). Tracking the roots of reading ability: White matter volume and integrity correlate with phonological awareness in prereading and early-reading kindergarten children. Journal of Neuroscience, 33(33), 13251–13258. doi:10.1523/JNEUROSCI.4383-12.2013 CrossRef Google Scholar PubMed
- Schmaal, L., Veltman, D.J., van Erp, T.G., Samann, P.G., Frodl, T., Jahanshad, N., & Hibar, D.P. (2015). Subcortical brain alterations in major depressive disorder: Findings from the ENIGMA Major Depressive Disorder working group. Molecular Psychiatry. doi:10.1038/mp.2015.69 Google Scholar PubMed
- Schopf, V., Kasprian, G., Brugger, P.C., & Prayer, D. (2012). Watching the fetal brain at ‘rest’. International Journal of Developmental Neuroscience, 30(1), 11–17. doi:10.1016/j.ijdevneu.2011.10.006 CrossRef Google Scholar
- Schumann, G., Binder, E.B., Holte, A., de Kloet, E.R., Oedegaard, K.J., Robbins, T.W., & Wittchen, H.U. (2014). Stratified medicine for mental disorders. European Neuropsychopharmacology, 24(1), 5–50. doi:10.1016/j.euroneuro.2013.09.010 CrossRef Google Scholar PubMed
- Seshamani, S., Cheng, X., Fogtmann, M., Thomason, M.E., & Studholme, C. (2014). A method for handling intensity inhomogenieties in fMRI sequences of moving anatomy of the early developing brain. Medical Image Analysis, 18(2), 285–300. doi:10.1016/j.media.2013.10.011 CrossRef Google Scholar PubMed
- Shaywitz, B.A., Shaywitz, S.E., Blachman, B.A., Pugh, K.R., Fulbright, R.K., Skudlarski, P., & Gore, J.C. (2004). Development of left occipitotemporal systems for skilled reading in children after a phonologically-based intervention. Biological Psychiatry, 55(9), 926–933. doi:10.1016/j.biopsych.2003.12.019 CrossRef Google Scholar PubMed
- Shen, M.D., Nordahl, C.W., Young, G.S., Wootton-Gorges, S.L., Lee, A., Liston, S.E., & Amaral, D.G. (2013). Early brain enlargement and elevated extra-axial fluid in infants who develop autism spectrum disorder. Brain, 136(Pt 9), 2825–2835. doi:10.1093/brain/awt166 CrossRef Google Scholar PubMed
- Silberg, J., Rutter, M., Neale, M., & Eaves, L. (2001). Genetic moderation of environmental risk for depression and anxiety in adolescent girls. The British Journal of Psychiatry, 179(2), 116–121. CrossRef Google Scholar PubMed
- Silk, J.S., Davis, S., McMakin, D.L., Dahl, R.E., & Forbes, E.E. (2012). Why do anxious children become depressed teenagers? The role of social evaluative threat and reward processing. Psychological Medicine, 42(10), 2095–2107. CrossRef Google Scholar PubMed
- Simos, P.G., Fletcher, J.M., Sarkari, S., Billingsley-Marshall, R., Denton, C.A., & Papanicolaou, A.C. (2007). Intensive instruction affects brain magnetic activity associated with oral word reading in children with persistent reading disabilities. Journal of Learning Disabilities, 40(1), 37–48. CrossRef Google Scholar PubMed
- Smith, L.K., Draper, E.S., & Field, D. (2014). Long-term outcome for the tiniest or most immature babies: Survival rates. Seminars in Fetal and Neonatal Medicine, 19(2), 72–77. doi:10.1016/j.siny.2013.11.002 CrossRef Google Scholar PubMed
- Smyser, C.D., Inder, T.E., Shimony, J.S., Hill, J.E., Degnan, A.J., Snyder, A.Z., & Neil, J.J. (2010). Longitudinal analysis of neural network development in preterm infants. Cerebral Cortex, 20(12), 2852–2862. doi:10.1093/cercor/bhq035 CrossRef Google Scholar PubMed
- Smyser, C.D., Snyder, A.Z., & Neil, J.J. (2011). Functional connectivity MRI in infants: Exploration of the functional organization of the developing brain. Neuroimage, 56(3), 1437–1452. doi:10.1016/j.neuroimage.2011.02.073 CrossRef Google Scholar PubMed
- Stepniak, B., Papiol, S., Hammer, C., Ramin, A., Everts, S., Hennig, L., & Ehrenreich, H. (2014). Accumulated environmental risk determining age at schizophrenia onset: A deep phenotyping-based study. Lancet Psychiatry, 1(6), 444–453. doi:10.1016/S2215-0366(14)70379-7 CrossRef Google Scholar PubMed
- Szatmari, P., Merette, C., Bryson, S.E., Thivierge, J., Roy, M.A., Cayer, M., & Maziade, M. (2002). Quantifying dimensions in autism: A factor-analytic study. Journal of the American Academy of Child and Adolescent Psychiatry, 41(4), 467–474. doi:10.1097/00004583-200204000-00020 CrossRef Google Scholar PubMed
- Tau, G.Z., & Peterson, B.S. (2010). Normal development of brain circuits. Neuropsychopharmacology, 35(1), 147–168. doi:10.1038/npp.2009.115 CrossRef Google Scholar
- Temple, E., Deutsch, G.K., Poldrack, R.A., Miller, S.L., Tallal, P., Merzenich, M.M., &Gabrieli, J.D. (2003). Neural deficits in children with dyslexia ameliorated by behavioral remediation: Evidence from functional MRI. Proceedings of the National Academy of Sciences of the United States of America, 100(5), 2860–2865. doi:10.1073/pnas.0030098100 CrossRef Google Scholar
- Thomason, M.E., Brown, J.A., Dassanayake, M.T., Shastri, R., Marusak, H.A., Hernandez-Andrade, E., & Romero, R. (2014). Intrinsic functional brain architecture derived from graph theoretical analysis in the human fetus. PLoS One, 9(5), e94423. doi:10.1371/journal.pone.0094423 CrossRef Google Scholar
- Thomason, M.E., Dassanayake, M.T., Shen, S., Katkuri, Y., Alexis, M., Anderson, A.L., & Romero, R. (2013). Cross-hemispheric functional connectivity in the human fetal brain. Science Translational Medicine, 5(173), 173ra124. doi:10.1126/scitranslmed.3004978 CrossRef Google Scholar
- Thomason, M.E., Grove, L.E., Lozon, T.A. Jr., Vila, A.M., Ye, Y., Nye, M.J., & Romero, R. (2015). Age-related increases in long-range connectivity in fetal functional neural connectivity networks in utero. Developmental Cognitive Neuroscience, 11, 96–104. doi:10.1016/j.dcn.2014.09.001 CrossRef Google Scholar
- Thompson, P.A., Hulme, C., Nash, H.M., Gooch, D., Hayiou-Thomas, E., & Snowling, M.J. (2015). Developmental dyslexia: Predicting individual risk. Journal of Child Psychology and Psychiatry, 56(9), 976–987. doi:10.1111/jcpp.12412 CrossRef Google Scholar
- Torgesen, J.K. (2000). Individual differences in response to early interventions in reading: The lingering problem of treatment resisters. Learning Disabilities Research & Practice, 15(1), 55–64. CrossRef Google Scholar
- Torgesen, J.K., Wagner, R.K., Rashotte, C.A., Rose, E., Lindamood, P., Conway, T., & Garvan, C. (1999). Preventing reading failure in young children with phonological processing disabilities: Group and individual responses to instruction. Journal of Educational Psychology, 91, 1–15. CrossRef Google Scholar
- Treyvaud, K., Ure, A., Doyle, L.W., Lee, K.J., Rogers, C.E., Kidokoro, H., & Anderson, P.J. (2013). Psychiatric outcomes at age seven for very preterm children: Rates and predictors. Journal of Child Psychology and Psychiatry, 54(7), 772–779. doi:10.1111/jcpp.12040 CrossRef Google Scholar PubMed
- Uehara, T., Yamasaki, T., Okamoto, T., Koike, T., Kan, S., Miyauchi, S., & Tobimatsu, S. (2014). Efficiency of a “small-world” brain network depends on consciousness level: A resting-state FMRI study. Cerebral Cortex, 24(6), 1529–1539. doi:10.1093/cercor/bht004 CrossRef Google Scholar PubMed
- Van den Bergh, B.R., & Marcoen, A. (2004). High antenatal maternal anxiety is related to ADHD symptoms, externalizing problems, and anxiety in 8- and 9-year-olds. Child Development, 75(4), 1085–1097. doi:10.1111/j.1467-8624.2004.00727.x CrossRef Google Scholar
- Van Essen, D.C., Smith, S.M., Barch, D.M., Behrens, T.E., Yacoub, E., Ugurbil, K., & WU-Minn HPC Consortium (2013). The WU-Minn HPC Consortium. Neuroimage, 80, 62–79. doi:10.1016/j.neuroimage.2013.05.041 CrossRef Google Scholar
- Vellutino, F.R., Scanlon, D.M., Sipay, E.R., Small, S.G., Pratt, A., Chen, R., & Dencla, M.B. (1996). Cognitive profiles of difficult-to-remediate and readily remediated poor readers: Early interventions as a vehicle for distinguishing between cognitive and experiential deficits as basic causes of specific reading disability. Journal of Educational Psychology, 88, 601–638. CrossRef Google Scholar
- Walker, L., Chang, L.C., Nayak, A., Irfanoglu, M.O., Botteron, K.N., McCracken, J., … Brain Development Cooperative Group. (2016). The diffusion tensor imaging (DTI) component of the NIH MRI study of normal brain development (PedsDTI). Neuroimage, 124, 1125–1130. doi:10.1016/j.neuroimage.2015.05.083 CrossRef Google Scholar
- Wang, X.H., Li, L., Xu, T., & Ding, Z. (2015). Investigating the temporal patterns within and between intrinsic connectivity networks under eyes-open and eyes-closed resting states: A dynamical functional connectivity study based on phase synchronization. PLoS One, 10(10), e0140300. doi:10.1371/journal.pone.0140300 CrossRef Google Scholar PubMed
- Wang, Z., Fernandez-Seara, M., Alsop, D.C., Liu, W.C., Flax, J.F., Benasich, A.A., & Detre, J.A. (2008). Assessment of functional development in normal infant brain using arterial spin labeled perfusion MRI. Neuroimage, 39(3), 973–978. doi:10.1016/j.neuroimage.2007.09.045 CrossRef Google Scholar PubMed
- Watanabe, T., Kessler, D., Scott, C., Angstadt, M., & Sripada, C. (2014). Disease prediction based on functional connectomes using a scalable and spatially-informed support vector machine. Neuroimage, 96, 183–202. doi:10.1016/j.neuroimage.2014.03.067 CrossRef Google Scholar PubMed
- Weissman, M.M., Fendrich, M., Warner, V., & Wickramaratne, P. (1992). Incidence of psychiatric disorder in offspring at high and low risk for depression. Journal of the American Academy of Child & Adolescent Psychiatry, 31(4), 640–648. CrossRef Google Scholar PubMed
- Weissman, M.M., Wickramaratne, P., Nomura, Y., Warner, V., Verdeli, H., Pilowsky, D.J., & Bruder, G. (2005). Families at high and low risk for depression: A 3-generation study. Archives of General Psychiatry, 62(1), 29–36. CrossRef Google Scholar PubMed
- Weissman, M.M., Wolk, S., Wickramaratne, P., Goldstein, R.B., Adams, P., Greenwald, S., & Steinberg, D. (1999). Children with prepubertal-onset major depressive disorder and anxiety grown up. Archives of General Psychiatry, 56(9), 794–801. CrossRef Google Scholar PubMed
- Welsh, R.C., Nemec, U., & Thomason, M.E. (2011). Fetal magnetic resonance imaging at 3.0 T. Topics in Magnetic Resonance Imaging, 22(3), 119–131. doi:10.1097/RMR.0b013e318267f932 CrossRef Google Scholar PubMed
- Werner, E.A., Myers, M.M., Fifer, W.P., Cheng, B., Fang, Y., Allen, R., & Monk, C. (2007). Prenatal predictors of infant temperament. Developmental Psychobiology, 49(5), 474–484. doi:10.1002/dev.20232 CrossRef Google Scholar PubMed
- White, T., Nelson, M., & Lim, K.O. (2008). Diffusion tensor imaging in psychiatric disorders. Topics in Magnetic Resonance Imaging, 19(2), 97–109. doi:10.1097/RMR.0b013e3181809f1e CrossRef Google Scholar
- Whitfield-Gabrieli, S., Thermenos, H.W., Milanovic, S., Tsuang, M.T., Faraone, S.V., McCarley, R.W., & Seidman, L.J. (2009). Hyperactivity and hyperconnectivity of the default network in schizophrenia and in first-degree relatives of persons with schizophrenia. Proceedings of the National Academy of Sciences of the United States of America, 106(4), 1279–1284. doi:10.1073/pnas.0809141106 CrossRef Google Scholar PubMed
- Wiggins, L.D., Robins, D.L., Adamson, L.B., Bakeman, R., & Henrich, C.C. (2012). Support for a dimensional view of autism spectrum disorders in toddlers. Journal of Autism and Developmental Disorders, 42(2), 191–200. doi:10.1007/s10803-011-1230-0 CrossRef Google Scholar
- Willcutt, E.G., Betjemann, R.S., McGrath, L.M., Chhabildas, N.A., Olson, R.K., DeFries, J.C., & Pennington, B.F. (2010). Etiology and neuropsychology of comorbidity between RD and ADHD: The case for multiple-deficit models. Cortex, 46(10), 1345–1361. doi:10.1016/j.cortex.2010.06.009 CrossRef Google Scholar PubMed
- Willcutt, E.G., Petrill, S.A., Wu, S., Boada, R., Defries, J.C., Olson, R.K., & Pennington, B.F. (2013). Comorbidity between reading disability and math disability: Concurrent psychopathology, functional impairment, and neuropsychological functioning. Journal of Learning Disabilities, 46(6), 500–516. doi:10.1177/0022219413477476 CrossRef Google Scholar
- Wolff, J.J., Botteron, K.N., Dager, S.R., Elison, J.T., Estes, A.M., Gu, H., & Network, I. (2014). Longitudinal patterns of repetitive behavior in toddlers with autism. Journal of Child Psychology and Psychiatry, 55(8), 945–953. doi:10.1111/jcpp.12207 CrossRef Google Scholar PubMed
- Wolff, J.J., Gerig, G., Lewis, J.D., Soda, T., Styner, M.A., Vachet, C., IBIS Network. (2015). Altered corpus callosum morphology associated with autism over the first 2 years of life. Brain, 138(Pt 7), 2046–2058. doi:10.1093/brain/awv118 CrossRef Google Scholar PubMed
- Wolff, J.J., Gu, H., Gerig, G., Elison, J.T., Styner, M., Gouttard, S., … IBIS Network. (2012). Differences in white matter fiber tract development present from 6 to 24 months in infants with autism. American Journal of Psychiatry, 169(6), 589–600. doi:10.1176/appi.ajp.2011.11091447 CrossRef Google Scholar PubMed
- Woodward, L.J., & Fergusson, D.M. (2001). Life course outcomes of young people with anxiety disorders in adolescence. Journal of the American Academy of Child and Adolescent Psychiatry, 40(9), 1086–1093. doi:10.1097/00004583-200109000-00018 CrossRef Google Scholar
- Yan, C.G., Cheung, B., Kelly, C., Colcombe, S., Craddock, R.C., Di Martino, A., & Milham, M.P. (2013). A comprehensive assessment of regional variation in the impact of head micromovements on functional connectomics. Neuroimage, 76(0), 183–201. doi:http://dx.doi.org/10.1016/j.neuroimage.2013.03.004 CrossRef Google Scholar
- Yan, C.G., Craddock, R.C., He, Y., & Milham, M.P. (2013). Addressing head motion dependencies for small-world topologies in functional connectomics. Frontiers in Human Neuroscience, 7, 910. doi:10.3389/fnhum.2013.00910 CrossRef Google Scholar PubMed
- Yan, C.G., Craddock, R.C., Zuo, X.-N., Zang, Y.-F., & Milham, M.P. (2013). Standardizing the intrinsic brain: Towards robust measurement of inter-individual variation in 1000 functional connectomes. Neuroimage, 80(0), 246–262. doi:http://dx.doi.org/10.1016/j.neuroimage.2013.04.081 CrossRef Google Scholar PubMed
- Young, J.M., Powell, T.L., Morgan, B.R., Card, D., Lee, W., Smith, M.L., & Taylor, M.J. (2015). Deep grey matter growth predicts neurodevelopmental outcomes in very preterm children. Neuroimage, 111, 360–368. doi:10.1016/j.neuroimage.2015.02.030 CrossRef Google Scholar PubMed
- Zuo, X.N., Anderson, J.S., Bellec, P., Birn, R.M., Biswal, B.B., Blautzik, J., & Milham, M.P. (2014). An open science resource for establishing reliability and reproducibility in functional connectomics. Scientific Data, 1, 140049. doi:10.1038/sdata.2014.49 CrossRef Google Scholar PubMed
The extended face network contains clusters of neurons that perform distinct functions on facial stimuli. Regions in the posterior ventral visual stream appear to perform basic perceptual functions on faces, while more anterior regions, such as the ventral anterior temporal lobe and amygdala, function to link mnemonic and affective information to faces. Anterior and posterior regions are interconnected by a long-range white matter tracts; however, it is not known if variation in connectivity of these pathways explains cognitive performance.
Here, we used diffusion imaging and deterministic tractography in a cohort of 28 neurologically normal adults ages 18–28 to examine microstructural properties of visual fiber pathways and their relationship to certain mnemonic and affective functions involved in face processing. We investigated how inter-individual variability in two tracts, the inferior longitudinal fasciculus (ILF) and the inferior fronto-occipital fasciculus (IFOF), related to performance on tests of facial emotion recognition and face memory.
Results revealed that microstructure of both tracts predicted variability in behavioral performance indexed by both tasks, suggesting that the ILF and IFOF play a role in facilitating our ability to discriminate emotional expressions in faces, as well as to remember unique faces. Variation in a control tract, theuncinate fasciculus, did not predict performance on these tasks.
These results corroborate and extend the findings of previous neuropsychology studies investigating the effects of damage to the ILF and IFOF, and demonstrate that differences in face processing abilities are related to white matter microstructure, even in healthy individuals. (JINS, 2016,22, 180–190)
- Alexander, A.L., Hurley, S.A., Samsonov, A.A., Adluru, N., Hosseinbor, A.P., Mossahebi, P., & Field, A.S. (2011). Characterization of cerebral white matter properties using quantitative magnetic resonance imaging stains. Brain Connectivity, 1(6), 423–446. http://doi.org/10.1089/brain.2011.0071 CrossRef Google Scholar PubMed
- Alexander, A.L., Lee, J.E., Lazar, M., & Field, A.S. (2007b). Diffusion tensor imaging of the brain. Neurotherapeutics, 4(3), 316–329. http://doi.org/10.1016/j.nurt.2007.05.011 CrossRef Google Scholar
- Alm, K.H., Rolheiser, T., Mohamed, F.B., & Olson, I.R. (2015). Fronto-temporal white matter connectivity predicts reversal learning errors. Frontiers in Neuroscience, 9(343), 1–11. doi:10.3389/fnhum.2015.00343 Google Scholar
- Almairac, F., Herbet, G., Moritz-Gasser, S., de Champfleur, N.M., & Duffau, H. (2014). The left inferior fronto-occipital fasciculus subserves language semantics: A multilevel lesion study. Brain Structure & Function. http://doi.org/10.1007/s00429-014-0773-1 Google Scholar
- Baggio, H.C., Segura, B., Ibarretxe-Bilbao, N., Valldeoriola, F., Marti, M.J., Compta, Y., & Junqué, C. (2012). Structural correlates of facial emotion recognition deficits in Parkinson’s disease patients. Neuropsychologia, 50(8), 2121–2128. http://doi.org/10.1016/j.neuropsychologia.2012.05.020 CrossRef Google Scholar PubMed
- Bauer, R.M. (1982). Visual hypoemotionality as a symptom of visual-limbic disconnection in man. Archives of Neurology, 39(11), 702–708. Retrieved from http://www.ncbi.nlm.nih.gov/pubmed/7126000 CrossRef Google Scholar PubMed
- Braem, B., Honoré, J., Rousseaux, M., Saj, A., & Coello, Y. (2014). Integration of visual and haptic informations in the perception of the vertical in young and old healthy adults and right brain-damaged patients. Neurophysiologie Clinique, 44(1), 41–48. http://doi.org/10.1016/j.neucli.2013.10.137 CrossRef Google Scholar
- Catani, M. (2007). From hodology to function. Brain, 130(Pt 3), 602–605. http://doi.org/10.1093/brain/awm008 CrossRef Google Scholar
- Catani, M., Jones, D.K., Donato, R., & Ffytche, D.H. (2003). Occipito-temporal connections in the human brain. Brain, 126(Pt 9), 2093–2107. http://doi.org/10.1093/brain/awg203 CrossRef Google Scholar
- Catani, M., & Thiebaut de Schotten, M. (2008). A diffusion tensor imaging tractography atlas for virtual in vivo dissections. Cortex, 44(8), 1105–1132. http://doi.org/10.1016/j.cortex.2008.05.004 CrossRef Google Scholar PubMed
- Coello, A.F., Duvaux, S., De Benedictis, A., Matsuda, R., & Duffau, H. (2013). Involvement of the right inferior longitudinal fascicle in visual hemiagnosia: A brain stimulation mapping study. Journal of Neurosurgery, 118(1), 202–205. http://doi.org/10.3171/2012.10.JNS12527 CrossRef Google Scholar
- Coello, Y., Bartolo, A., Amiri, B., Devanne, H., Houdayer, E., & Derambure, P. (2008). Perceiving what is reachable depends on motor representations: Evidence from a transcranial magnetic stimulation study. PLoS One, 3(8), e2862. http://doi.org/10.1371/journal.pone.0002862 CrossRef Google Scholar PubMed
- De Zubicaray, G.I., Rose, S.E., & McMahon, K.L. (2011). The structure and connectivity of semantic memory in the healthy older adult brain. Neuroimage, 54(2), 1488–1494. http://doi.org/10.1016/j.neuroimage.2010.08.058 CrossRef Google Scholar
- Diehl, B., Busch, R.M., Duncan, J.S., Piao, Z., Tkach, J., & Lüders, H.O. (2008). Abnormalities in diffusion tensor imaging of the uncinate fasciculus relate to reduced memory in temporal lobe epilepsy. Epilepsia, 49(8), 1409–1418. http://doi.org/10.1111/j.1528-1167.2008.01596.x CrossRef Google Scholar
- Duchaine, B., & Nakayama, K. (2006). The Cambridge Face Memory Test: Results for neurologically intact individuals and an investigation of its validity using inverted face stimuli and prosopagnosic participants. Neuropsychologia, 44(4), 576–585. http://doi.org/10.1016/j.neuropsychologia.2005.07.001 CrossRef Google Scholar PubMed
- Duffau, H., Gatignol, P., Mandonnet, E., Peruzzi, P., Tzourio-Mazoyer, N., & Capelle, L. (2005). New insights into the anatomo-functional connectivity of the semantic system: A study using cortico-subcortical electrostimulations. Brain, 128(Pt 4), 797–810. http://doi.org/10.1093/brain/awh423 CrossRef Google Scholar PubMed
- Duffau, H., Peggy Gatignol, S.T., Mandonnet, E., Capelle, L., & Taillandier, L. (2008). Intraoperative subcortical stimulation mapping of language pathways in a consecutive series of 115 patients with Grade II glioma in the left dominant hemisphere. Journal of Neurosurgery, 109(3), 461–471. http://doi.org/10.3171/JNS/2008/109/9/0461 CrossRef Google Scholar
- Ekman, P., & Friesen, W.V. (1976). Measuring facial movement. Environmental Psychology and Nonverbal Behavior, 1(1), 56–75. http://doi.org/10.1007/BF01115465 CrossRef Google Scholar
- Epelbaum, S., Pinel, P., Gaillard, R., Delmaire, C., Perrin, M., Dupont, S., & Cohen, L. (2008). Pure alexia as a disconnection syndrome: New diffusion imaging evidence for an old concept. Cortex, 44(8), 962–974. http://doi.org/10.1016/j.cortex.2008.05.003 CrossRef Google Scholar
- Genova, H.M., Rajagopalan, V., Chiaravalloti, N., Binder, A., Deluca, J., & Lengenfelder, J. (2015). Facial affect recognition linked to damage in specific white matter tracts in traumatic brain injury. Social Neuroscience, 10(1), 27–34. doi:10.1080/17470919.2014.959618. CrossRef Google Scholar PubMed
- Gil-Robles, S., Carvallo, A., Jimenez, M.D.M., Gomez Caicoya, A., Martinez, R., Ruiz-Ocaña, C., & Duffau, H. (2013). Double dissociation between visual recognition and picture naming: A study of the visual language connectivity using tractography and brain stimulation. Neurosurgery, 72(4), 678–686. http://doi.org/10.1227/NEU.0b013e318282a361 CrossRef Google Scholar
- Gong, G., He, Y., & Evans, A.C. (2011). Brain connectivity: Gender makes a difference. The Neuroscientist, 17(5), 575–591. http://doi.org/10.1177/1073858410386492 CrossRef Google Scholar
- Grossi, D., Soricelli, A., Ponari, M., Salvatore, E., Quarantelli, M., Prinster, A., & Trojano, L. (2014). Structural connectivity in a single case of progressive prosopagnosia: The role of the right inferior longitudinal fasciculus. Cortex, 56, 111–120. http://doi.org/10.1016/j.cortex.2012.09.010 CrossRef Google Scholar
- Habib, M. (1986). Visual hypoemotionality and prosopagnosia associated with right temporal lobe isolation. Neuropsychologia, 24(4), 577–582. Retrieved from http://www.ncbi.nlm.nih.gov/pubmed/3774141 CrossRef Google Scholar
- Hoeft, F., Meyler, A., Hernandez, A., Juel, C., Taylor-Hill, H., Martindale, J.L., McMillon, G., Kolchugina, G., … Gabrieli, J. D. (2007). Functional and morphometric brain dissociation between dyslexia and reading ability. Behavioral Neuroscience, 121(3), 602–613. CrossRef Google Scholar
- Horel, J.A., & Misantone, L.J. (1974). The Klüver-Bucy syndrome produced by partial isolation of the temporal lobe. Experimental Neurology, 42(1), 101–112. http://doi.org/10.1016/0014-4886(74)90010-7 CrossRef Google Scholar PubMed
- Ingalhalikar, M., Smith, A., Parker, D., Satterthwaite, T.D., Elliott, M.A., Ruparel, K., & Verma, R. (2014). Sex differences in the structural connectome of the human brain. Proceedings of the National Academy of Sciences of the United States of America, 111(2), 823–828. http://doi.org/10.1073/pnas.1316909110 CrossRef Google Scholar PubMed
- Johansen-Berg, H., & Behrens, T.E.J. (2009). Diffusion MRI. Amsterdam: Elsevier. http://doi.org/10.1016/B978-0-12-374709-9.00022-5 Google Scholar
- Jones, D.K. (2008). Studying connections in the living human brain with diffusion MRI. Cortex, 44(8), 936–952. http://doi.org/10.1016/j.cortex.2008.05.002 CrossRef Google Scholar PubMed
- Lebel, C., Gee, M., Camicioli, R., Wieler, M., Martin, W., & Beaulieu, C. (2012). Diffusion tensor imaging of white matter tract evolution over the lifespan. Neuroimage, 60(1), 340–352. http://doi.org/10.1016/j.neuroimage.2011.11.094 CrossRef Google Scholar
- Mandonnet, E., Nouet, A., Gatignol, P., Capelle, L., & Duffau, H. (2007). Does the left inferior longitudinal fasciculus play a role in language? A brain stimulation study. Brain, 130(Pt 3), 623–629. http://doi.org/10.1093/brain/awl361 CrossRef Google Scholar
- McDonald, C.R., Hagler, D.J., Girard, H.M., Pung, C., Ahmadi, M.E., Holland, D., & Dale, A.M. (2010). Changes in fiber tract integrity and visual fields after anterior temporal lobectomy. Neurology, 75(18), 1631–1638. http://doi.org/10.1212/WNL.0b013e3181fb44db CrossRef Google Scholar
- Metzler-Baddeley, C., Jones, D.K., Belaroussi, B., Aggleton, J.P., & O’Sullivan, M.J. (2011). Frontotemporal connections in episodic memory and aging: A diffusion MRI tractography study. The Journal of Neuroscience, 31(37), 13236–13245. http://doi.org/10.1523/JNEUROSCI.2317-11.2011 CrossRef Google Scholar PubMed
- Mori, S., Crain, B.J., Chacko, V.P., & Van Zijl, P.C.M. (1999). Three-dimensional tracking of axonal projections in the brain by magnetic resonance imaging. Annals of Neurology, 45(2), 265–269. http://doi.org/10.1002/1531-8249(199902)45:2<265::AID-ANA21>3.0.CO;2-33.0.CO;2-3>CrossRef Google Scholar PubMed
- Mori, S., Kaufmann, W.E., Davatzikos, C., Stieltjes, B., Amodei, L., Fredericksen, K., & van Zijl, P.C.M. (2002). Imaging cortical association tracts in the human brain using diffusion-tensor-based axonal tracking. Magnetic Resonance in Medicine, 47(2), 215–223. http://doi.org/10.1002/mrm.10074 CrossRef Google Scholar
- Mundfrom, D.J., Piccone, A., Perrett, J.J., Schaffer, J., & Roozeboom, M. (2006). Bonferroni adjustments in tests for regression coefficients. Multiple Linear Regression Viewpoints, 32, 1–6. Google Scholar
- Niogi, S.N., Mukherjee, P., Ghajar, J., Johnson, C.E., Kolster, R., Lee, H., & McCandliss, B.D. (2008). Structural dissociation of attentional control and memory in adults with and without mild traumatic brain injury. Brain, 131(Pt 12), 3209–3221. http://doi.org/10.1093/brain/awn247 CrossRef Google Scholar PubMed
- Olson, I.R., Heide, R.J., Alm, K.H., & Vyas, G. (2015). Development of the uncinate fasciculus: implications for theory and developmental disorders. Developmental Cognitive Neuroscience, 14, 50–61. CrossRef Google Scholar
- Philippi, C.L., Mehta, S., Grabowski, T., Adolphs, R., & Rudrauf, D. (2009). Damage to association fiber tracts impairs recognition of the facial expression of emotion. The Journal of Neuroscience, 29(48), 15089–15099. http://doi.org/10.1523/JNEUROSCI.0796-09.2009 CrossRef Google Scholar PubMed
- Sarubbo, S., De Benedictis, A., Maldonado, I.L., Basso, G., & Duffau, H. (2013). Frontal terminations for the inferior fronto-occipital fascicle: Anatomical dissection, DTI study and functional considerations on a multi-component bundle. Brain Structure & Function, 218(1), 21–37. http://doi.org/10.1007/s00429-011-0372-3 CrossRef Google Scholar PubMed
- Schmahmann, J.D., & Pandya, D.N. (2007). The complex history of the fronto-occipital fasciculus. Journal of the History of the Neurosciences, 16, 362–377. CrossRef Google Scholar
- Smith, S.M., Jenkinson, M., Woolrich, M.W., Beckmann, C.F., Behrens, T.E.J., Johansen-Berg, H., & Matthews, P.M. (2004). Advances in functional and structural MR image analysis and implementation as FSL. Neuroimage, 23(Suppl. 1), S208–S219. http://doi.org/10.1016/j.neuroimage.2004.07.051 CrossRef Google Scholar
- Sprague, J.M. (1966). Interaction of cortex and superior colliculus in mediation of visually guided behavior in the cat. Science (New York, N.Y.), 153(3743), 1544–1547. Retrieved from http://www.ncbi.nlm.nih.gov/pubmed/5917786 CrossRef Google Scholar
- Tavor, I., Yablonski, M., Mezer, A., Rom, S., Assaf, Y., & Yovel, G. (2014). Separate parts of occipito-temporal white matter fibers are associated with recognition of faces and places. Neuroimage, 86(2014), 123–130. http://doi.org/10.1016/j.neuroimage.2013.07.085 CrossRef Google Scholar
- Thomas, A.L., Lawler, K., Olson, I.R., & Aguirre, G.K. (2008). The Philadelphia Face Perception Battery. Archives of Clinical Neuropsychology, 23(2), 175–187. http://doi.org/10.1016/j.acn.2007.10.003 CrossRef Google Scholar PubMed
- Thomas, C., Avidan, G., Humphreys, K., Jung, K., Gao, F., & Behrmann, M. (2009). Reduced structural connectivity in ventral visual cortex in congenital prosopagnosia. Nature Neuroscience, 12(1), 29–31. http://doi.org/10.1038/nn.2224 CrossRef Google Scholar PubMed
- Thomas, C., Humphreys, K., Jung, K.-J., Minshew, N., & Behrmann, M. (2010). The anatomy of the callosal and visual-association pathways in high-functioning autism: A DTI tractography study. Cortex, 47(7), 863–873. http://doi.org/10.1016/j.cortex.2010.07.006 CrossRef Google Scholar PubMed
- Thomas, C., Moya, L., Avidan, G., Humphreys, K., Jung, K.J., Peterson, M.A., & Behrmann, M. (2008). Reduction in white matter connectivity, revealed by diffusion tensor imaging, may account for age-related changes in face perception. Journal of Cognitive Neuroscience, 20(2), 268–284. CrossRef Google Scholar PubMed
- Tournier, J.-D., Mori, S., & Leemans, A. (2011). Diffusion tensor imaging and beyond. Magnetic Resonance in Medicine, 65(6), 1532–1556. http://doi.org/10.1002/mrm.22924 CrossRef Google Scholar
- Valdés-Sosa, M., Bobes, M.A., Quiñones, I., Garcia, L., Valdes-Hernandez, P.A., Iturria, Y., & Asencio, J. (2011). Covert face recognition without the fusiform-temporal pathways. Neuroimage, 57(3), 1162–1176. http://doi.org/10.1016/j.neuroimage.2011.04.057 CrossRef Google Scholar PubMed
- Von Der Heide, R.J., Skipper, L.M., Klobusicky, E., & Olson, I.R. (2013). Dissecting the uncinate fasciculus: Disorders, controversies and a hypothesis. Brain, 136(Pt 6), 1692–1707. http://doi.org/10.1093/brain/awt094 CrossRef Google Scholar
- Wakana, S., Caprihan, A., Panzenboeck, M.M., Fallon, J.H., Perry, M., Gollub, R.L., & Mori, S. (2007). Reproducibility of quantitative tractography methods applied to cerebral white matter. Neuroimage, 36(3), 630–644. http://doi.org/10.1016/j.neuroimage.2007.02.049 CrossRef Google Scholar
- Wang, R., Benner, T., Sorensen, A.G., & Wedeen, V.J. (2007). Diffusion toolkit: A software package for diffusion imaging data processing and tractography. Proceedings of the 15th Scientific Meeting of the International Society for Magnetic Resonance in Medicine, Seattle, Washington. Abstract 3720. Google Scholar
- Yamasaki, T., Taniwaki, T., Tobimatsu, S., Arakawa, K., Kuba, H., Maeda, Y., & Kira, J. (2004). Electrophysiological correlates of associative visual agnosia lesioned in the ventral pathway. Journal of the Neurological Sciences, 221(1-2), 53–60. http://doi.org/10.1016/j.jns.2004.03.024 CrossRef Google Scholar PubMed
- Yeatman, J.D., Rauschecker, A.M., & Wandell, B.A. (2013). Anatomy of the visual word form area: Adjacent cortical circuits and long-range white matter connections. Brain and Language, 125(2), 146–155. http://doi.org/10.1016/j.bandl.2012.04.010 CrossRef Google Scholar PubMed
- Zemmoura, I., Herbet, G., Moritz-Gasser, S., & Duffau, H. (2015). New insights into the neural network mediating reading processes provided by cortico-subcortical electrical mapping. Human Brain Mapping, 36, 2215–2230. http://doi.org/10.1002/hbm.22766 CrossRef Google Scholar PubMed
The purpose of this study was to assess whether age-related differences in white matter microstructure are associated with altered task-related connectivity during episodic recognition.
Using functional magnetic resonance imaging and diffusion tensor imaging from 282 cognitively healthy middle-to-late aged adults enrolled in the Wisconsin Registry for Alzheimer’s Prevention, we investigated whether fractional anisotropy (FA) within white matter regions known to decline with age was associated with task-related connectivity within the recognition network.
There was a positive relationship between fornix FA and memory performance, both of which negatively correlated with age. Psychophysiological interaction analyses revealed that higher fornix FA was associated with increased task-related connectivity amongst the hippocampus, caudate, precuneus, middle occipital gyrus, and middle frontal gyrus. In addition, better task performance was associated with increased task-related connectivity between the posterior cingulate gyrus, middle frontal gyrus, cuneus, and hippocampus.
The findings indicate that age has a negative effect on white matter microstructure, which in turn has a negative impact on memory performance. However, fornix microstructure did not significantly mediate the effect of age on performance. Of interest, dynamic functional connectivity was associated with better memory performance. The results of the psychophysiological interaction analysis further revealed that alterations in fornix microstructure explain–at least in part–connectivity among cortical regions in the recognition memory network. Our results may further elucidate the relationship between structural connectivity, neural function, and cognition. (JINS, 2016,22, 191–204)
- Adluru, N., Destiche, D.J., Lu, S. Y.-F., Doran, S.T., Birdsill, A.C., Melah, K.E., & Bendlin, B.B. (2014). White matter microstructure in late middle-age: Effects of apolipoprotein E4 and parental family history of Alzheimer’s disease. Neuroimage. Clinical, 4, 730–742. http://doi.org/10.1016/j.nicl.2014.04.008 CrossRef Google Scholar PubMed
- Aggleton, J.P., & Brown, M.W. (1999). Episodic memory, amnesia, and the hippocampal-anterior thalamic axis. The Behavioral and Brain Sciences, 22(3), 425–444; discussion 444–489. http://doi.org/10.1017/S0140525X99002034 CrossRef Google Scholar
- Aggleton, J.P., & Brown, M.W. (2006). Interleaving brain systems for episodic and recognition memory. Trends in Cognitive Sciences. http://doi.org/10.1016/j.tics.2006.08.003 CrossRef Google Scholar
- Anderson, N.D., Iidaka, T., Cabeza, R., Kapur, S., McIntosh, A.R., & Craik, F.I. (2000). The effects of divided attention on encoding- and retrieval-related brain activity: A PET study of younger and older adults. Journal of Cognitive Neuroscience, 12(5), 775–792. http://doi.org/10.1162/089892900562598 CrossRef Google Scholar PubMed
- Antonenko, D., Meinzer, M., Lindenberg, R., Witte, A.V., & Flöel, A. (2012). Grammar learning in older adults is linked to white matter microstructure and functional connectivity. Neuroimage, 62(3), 1667–1674. http://doi.org/10.1016/j.neuroimage.2012.05.074 CrossRef Google Scholar PubMed
- Ashburner, J., & Friston, K.J. (1999). Nonlinear spatial normalization using basis functions. Human Brain Mapping, 7(4), 254–266. http://doi.org/10.1002/(SICI)1097-0193(1999)7:4<254::AID-HBM4>3.0.CO;2-G3.0.CO;2-G>CrossRef Google Scholar PubMed
- Baddeley, A. (1996). Exploring the central executive. The Quarterly Journal of Experimental Psychology, 49A(1), 5–28. CrossRef Google Scholar
- Beaulieu, C. (2002). The basis of anisotropic water diffusion in the nervous system - A technical review. NMR in Biomedicine, 15(7-8), 435–455. http://doi.org/10.1002/nbm.782 CrossRef Google Scholar
- Benedict, R. (1997). Brief Visuospatial Memory Test-Revised. Odessa, FL: Psychological Assessment Resources, Inc. Google Scholar
- Braak, H., & Braak, E. (1995). Staging of Alzheimer’s disease-related neurofibrillary changes. In Neurobiology of aging (Vol. 16, pp. 271–278). http://doi.org/10.1016/0197-4580(95)00021-6 Google Scholar
- Cabeza, R. (2004). Task-independent and task-specific age effects on brain activity during working memory, visual attention and episodic retrieval. Cerebral Cortex, 14(4), 364–375. http://doi.org/10.1093/cercor/bhg133 CrossRef Google Scholar PubMed
- Cabeza, R., Anderson, N.D., Houle, S., Mangels, J.A., & Nyberg, L. (2000). Age-related differences in neural activity during item and temporal-order memory retrieval: A positron emission tomography study. Journal of Cognitive Neuroscience, 12(1), 197–206. CrossRef Google Scholar PubMed
- Cabeza, R., & Dennis, N. (2012). Frontal lobes and aging: Deterioration and compensation. In Principles of frontal lobe function (pp. 628–652). http://doi.org/10.1093/acprof:oso/9780195134971.001.0001 Google Scholar
- Cabeza, R., Grady, C.L., Nyberg, L., McIntosh, A.R., Tulving, E., Kapur, S., & Craik, F.I. (1997). Age-related differences in neural activity during memory encoding and retrieval: A positron emission tomography study. The Journal of Neuroscience, 17(1), 391–400. http://doi.org/0270-6474/96/170391-10$05.00/0 Google Scholar PubMed
- Cassel, J.C., Duconseille, E., Jeltsch, H., & Will, B. (1997). The fimbria-fornix/cingular bundle pathways: A review of neurochemical and behavioural approaches using lesions and transplantation techniques. Progress in Neurobiology, 51(6), 663–716. http://doi.org/10.1016/S0301-0082(97)00009-9 CrossRef Google Scholar PubMed
- Catani, M., Howard, R.J., Pajevic, S., & Jones, D.K. (2002). Virtual in vivo interactive dissection of white matter fasciculi in the human brain. Neuroimage, 17(1), 77–94. CrossRef Google Scholar
- Charlton, R.A., Schiavone, F., Barrick, T.R., Morris, R.G., & Markus, H.S. (2010). Diffusion tensor imaging detects age related white matter change over a 2 year follow-up which is associated with working memory decline. Journal of Neurology, Neurosurgery, and Psychiatry, 81(1), 13–19. http://doi.org/10.1136/jnnp.2008.167288 CrossRef Google Scholar
- Crosby, E.C. (1962). Correlative anatomy of the nervous system. Macmillan. Retrieved from http://books.google.com/books/about/Correlative_anatomy_of_the_nervous_syste.html?id=zrRqAAAAMAAJ&pgis=1 Google Scholar
- Daselaar, S.M., Fleck, M.S., Dobbins, I.G., Madden, D.J., & Cabeza, R. (2006). Effects of healthy aging on hippocampal and rhinal memory functions: An event-related fMRI study. Cerebral Cortex, 16(12), 1771–1782. http://doi.org/10.1093/cercor/bhj112 CrossRef Google Scholar
- Davis, S.W., Dennis, N.A., Daselaar, S.M., Fleck, M.S., & Cabeza, R. (2008). Qué PASA? the posterior-anterior shift in aging. Cerebral Cortex, 18(5), 1201–1209. http://doi.org/10.1093/cercor/bhm155 CrossRef Google Scholar
- Davis, S.W., Kragel, J.E., Madden, D.J., & Cabeza, R. (2012). The architecture of cross-hemispheric communication in the aging brain: Linking behavior to functional and structural connectivity. Cerebral Cortex, 22(1), 232–242. http://doi.org/10.1093/cercor/bhr123 CrossRef Google Scholar
- Du, A.T., Schuff, N., Zhu, X.P., Jagust, W.J., Miller, B.L., Reed, B.R., & Weiner, M.W. (2003). Atrophy rates of entorhinal cortex in AD and normal aging. Neurology, 60(3), 481–486. CrossRef Google Scholar
- Duverne, S., Motamedinia, S., & Rugg, M.D. (2009). The relationship between aging, performance, and the neural correlates of successful memory encoding. Cerebral Cortex, 19(3), 733–744. http://doi.org/10.1093/cercor/bhn122 CrossRef Google Scholar
- Faure, A., Haberland, U., Condé, F., & El Massioui, N. (2005). Lesion to the nigrostriatal dopamine system disrupts stimulus-response habit formation. The Journal of Neuroscience, 25(11), 2771–2780. http://doi.org/10.1523/JNEUROSCI.3894-04.2005 CrossRef Google Scholar PubMed
- Finsterer, J., & Fuglsang-Frederiksen, A. (2000). Concentric needle EMG versus macro EMG I. Relation in healthy subjects. Clinical Neurophysiology, 111(7), 1211–1215. http://doi.org/10.1016/S1388-2457(00)00310-2 CrossRef Google Scholar PubMed
- Folstein, M.F., Folstein, S.E., & McHugh, P.R. (1975). “Mini-mental state.” A practical method for grading the cognitive state of patients for the clinician. Journal of Psychiatric Research, 12(3), 189–198. http://doi.org/10.1016/0022-3956(75)90026-6 CrossRef Google Scholar
- Gläscher, J., Rudrauf, D., Colom, R., Paul, L.K., Tranel, D., Damasio, H., & Adolphs, R. (2010). Distributed neural system for general intelligence revealed by lesion mapping. Proceedings of the National Academy of Sciences of the United States of America, 107(10), 4705–4709. http://doi.org/10.1073/pnas.0910397107 CrossRef Google Scholar
- Grady, C.L. (2008). Cognitive neuroscience of aging. Annals of the New York Academy of Sciences, 1124, 127–144. http://doi.org/10.1196/annals.1440.009 CrossRef Google Scholar
- Grady, C.L., Grady, C.L., McIntosh, A.R., McIntosh, A.R., Craik, F.I.M., & Craik, F.I.M. (2005). Task-related activity in prefrontal cortex and its relation to recognition memory performance in young and old adults. Neuropsychologia, 43(10), 1466–1481. http://doi.org/10.1016/j.neuropsychologia.2004.12.016 CrossRef Google Scholar
- Grady, C.L., McIntosh, A.R., & Craik, F.I.M. (2003). Age-related differences in the functional connectivity of the hippocampus during memory encoding. Hippocampus, 13(5), 572–586. http://doi.org/10.1002/hipo.10114 CrossRef Google Scholar PubMed
- Greenwood, P.M. (2007). Functional plasticity in cognitive aging: Review and hypothesis. Neuropsychology, 21(6), 657–673. http://doi.org/10.1037/0894-4105.21.6.657 CrossRef Google Scholar
- Gunning-Dixon, F.M., & Raz, N. (2000). The cognitive correlates of white matter abnormalities in normal aging: A quantitative review. Neuropsychology, 14(2), 224–232. http://doi.org/10.1037//0894-4105.14.2.224 CrossRef Google Scholar PubMed
- Harvey, L.O. (1992). The critical operating characteristic and the evaluation of expert judgment. Organizational Behavior and Human Decision Processes. Available from http://doi.org/10.1016/0749-5978(92)90063-D CrossRef Google Scholar
- Head, D., Kennedy, K.M., Rodrigue, K.M., & Raz, N. (2009). Age differences in perseveration: Cognitive and neuroanatomical mediators of performance on the Wisconsin Card Sorting Test. Neuropsychologia, 47(4), 1200–1203. http://doi.org/10.1016/j.neuropsychologia.2009.01.003 CrossRef Google Scholar PubMed
- Hellyer, P.J., Shanahan, M., Scott, G., Wise, R.J.S., Sharp, D.J., & Leech, R. (2014). The control of global brain dynamics: Opposing actions of frontoparietal control and default mode networks on attention. The Journal of Neuroscience, 34(2), 451–461. http://doi.org/10.1523/JNEUROSCI.1853-13.2014 CrossRef Google Scholar
- Jastak, S., & Wilkinson, G.S. (1984). The Wide Range Achievement test - Revised (Vol. III). Wilmington, DE: Jastak Associates. Google Scholar
- Kaplan, E., Goodglass, H., & Weintraub, S. (1983). Boston Naming Test. Philadelphia: Lea & Febiger. Google Scholar
- Kawas, C., Segal, J., Stewart, W.F., Corrada, M., & Thal, L.J. (1994). A validation study of the Dementia Questionnaire. Archives of Neurology, 51(9), 901–906. http://doi.org/10.1001/archneur.1994.00540210073015 CrossRef Google Scholar PubMed
- Kehoe, E.G., Farrell, D., Metzler-Baddeley, C., Lawlor, B.A., Kenny, R.A., Lyons, D., & Bokde, A.L. (2015). Fornix white matter is correlated with resting-state functional connectivity of the thalamus and hippocampus in healthy aging but not in mild cognitive impairment - A preliminary study. Frontiers in Aging Neuroscience, 7, 10. http://doi.org/10.3389/fnagi.2015.00010 CrossRef Google Scholar
- King, D.R., de Chastelaine, M., Elward, R.L., Wang, T.H., & Rugg, M.D. (2015). Recollection-related increases in functional connectivity predict individual differences in memory accuracy. Journal of Neuroscience, 35(4), 1763–1772. http://doi.org/10.1523/JNEUROSCI.3219-14.2015 CrossRef Google Scholar PubMed
- Lamont, A.C., Stewart-Williams, S., & Podd, J. (2005). Face recognition and aging: Effects of target age and memory load. Memory & Cognition, 33(6), 1017–1024. http://doi.org/10.3758/BF03193209 CrossRef Google Scholar PubMed
- Lockhart, S.N., Mayda, A.B.V., Roach, A.E., Fletcher, E., Carmichael, O., Maillard, P., & DeCarli, C. (2012). Episodic memory function is associated with multiple measures of white matter integrity in cognitive aging. Frontiers in Human Neuroscience, 6, 56. http://doi.org/10.3389/fnhum.2012.00056 CrossRef Google Scholar PubMed
- Luk, G., Bialystok, E., Craik, F.I.M., & Grady, C.L. (2011). Lifelong bilingualism maintains white matter integrity in older adults. Journal of Neuroscience, 31(46), 16808–16813. http://doi.org/10.1523/JNEUROSCI.4563-11.2011 CrossRef Google Scholar
- McKhann, G.M., Drachman, D., Folstein, M.F., Katzman, R., Price, D., & Stadlan, E.M. (1984). Clinical diagnosis of Alzheimer’s disease: Report of the NINCDS-ADRDA Work Group under the auspices of Department of Health and Human Services Task Force on Alzheimer’s Disease. Neurology, 34(7), 939–944. http://www.ncbi.nlm.nih.gov/pubmed/6610841 CrossRef Google Scholar PubMed
- McKhann, G.M., Knopman, D.S., Chertkow, H., Hyman, B.T., Jack, C.R., Kawas, C.H., & Phelps, C.H. (2011). The diagnosis of dementia due to Alzheimer’s disease: Recommendations from the National Institute on Aging-Alzheimer’s Association workgroups on diagnostic guidelines for Alzheimer’s disease. Alzheimer’s & Dementia, 7(3), 263–269. http://doi.org/10.1016/j.jalz.2011.03.005 CrossRef Google Scholar
- McLaren, D.G., Ries, M.L., Xu, G., & Johnson, S.C. (2012). A generalized form of context-dependent psychophysiological interactions (gPPI): A comparison to standard approaches. Neuroimage, 61(4), 1277–1286. http://doi.org/10.1016/j.neuroimage.2012.03.068 CrossRef Google Scholar PubMed
- McLaren, D.G., Sperling, R.A., & Atri, A. (2014). Flexible modulation of network connectivity related to cognition in alzheimer’s disease. Neuroimage, 100, 544–557. http://doi.org/10.1016/j.neuroimage.2014.05.032 CrossRef Google Scholar
- McNab, F., & Klingberg, T. (2008). Prefrontal cortex and basal ganglia control access to working memory. Nature Neuroscience, 11(1), 103–107. http://doi.org/10.1038/nn2024 CrossRef Google Scholar PubMed
- Metzler-Baddeley, C., Jones, D.K., Belaroussi, B., Aggleton, J.P., & O’Sullivan, M.J. (2011). Frontotemporal connections in episodic memory and aging: A diffusion MRI tractography study. The Journal of Neuroscience, 31(37), 13236–13245. http://doi.org/10.1523/JNEUROSCI.2317-11.2011 CrossRef Google Scholar
- Morcom, A.M., Good, C.D., Frackowiak, R.S.J., & Rugg, M.D. (2003). Age effects on the neural correlates of successful memory encoding. Brain, 126(1), 213–229. http://doi.org/10.1093/brain/awg020 CrossRef Google Scholar PubMed
- Nandedkar, S., & Stalberg, E. (1983). Simulation of macro EMG motor unit potentials. Electroencephalography and Clinical Neurophysiology, 56(1), 52–62. http://doi.org/10.1016/0013-4694(83)90006-8 CrossRef Google Scholar
- Nicholas, C.R., Okonkwo, O.C., Bendlin, B.B., Oh, J.M., Asthana, S., Rowley, H.A., & Johnson, S.C. (2014). Posteromedial hyperactivation during episodic recognition among people with memory decline: Findings from the WRAP study. Brain Imaging and Behavior. [Epub ahead of print]. http://doi.org/10.1007/s11682-014-9322-z Google Scholar
- O’Sullivan, M., Jones, D.K., Summers, P.E., Morris, R.G., Williams, S.C., & Markus, H.S. (2001). Evidence for cortical “disconnection” as a mechanism of age-related cognitive decline. Neurology, 57(4), 632–638. http://doi.org/10.1212/WNL.57.4.632 CrossRef Google Scholar PubMed
- Persson, J., Nyberg, L., Lind, J., Larsson, A., Nilsson, L.G., Ingvar, M., & Buckner, R.L. (2006). Structure-function correlates of cognitive decline in aging. Cerebral Cortex, 16(7), 907–915. http://doi.org/10.1093/cercor/bhj036 CrossRef Google Scholar
- Pfefferbaum, A., Adalsteinsson, E., & Sullivan, E.V. (2005). Frontal circuitry degradation marks healthy adult aging: Evidence from diffusion tensor imaging. Neuroimage, 26(3), 891–899. http://doi.org/10.1016/j.neuroimage.2005.02.034 CrossRef Google Scholar PubMed
- Poletti, C.E., & Creswell, G. (1977). Fornix system efferent projections in the squirrel monkey: An experimental degeneration study. The Journal of Comparative Neurology, 175(1), 101–128. http://doi.org/10.1002/cne.901750107 CrossRef Google Scholar
- Rajah, M.N., Languay, R., & Grady, C.L. (2011). Age-related changes in right middle frontal gyrus volume correlate with altered episodic retrieval activity. Journal of Neuroscience, 31(49), 17941–17954. http://doi.org/10.1523/JNEUROSCI.1690-11.2011 CrossRef Google Scholar PubMed
- Raz, N., Rodrigue, K.M., Kennedy, K.M., Dahle, C., Head, D., & Acker, J.D. (2003). Differential age-related changes in the regional metencephalic volumes in humans: A 5-year follow-up. Neuroscience Letters, 349(3), 163–166. http://doi.org/10.1016/S0304-3940(03)00820-6 CrossRef Google Scholar PubMed
- Reitan, R., & Wolfson, D. (1993). The Halstead-Reitan Neuropsychological Test Battery: Theory and clinical interpretation. Tucson, AZ: Neuropsychology Press. Google Scholar
- Rodrigue, K.M., & Raz, N. (2004). Shrinkage of the entorhinal cortex over five years predicts memory performance in healthy adults. The Journal of Neuroscience, 24(4), 956–963. http://doi.org/10.1523/JNEUROSCI.4166-03.2004 CrossRef Google Scholar PubMed
- Rudebeck, S.R., Scholz, J., Millington, R., Rohenkohl, G., Johansen-Berg, H., & Lee, A.C.H. (2009). Fornix microstructure correlates with recollection but not familiarity memory. The Journal of Neuroscience, 29(47), 14987–14992. http://doi.org/10.1523/JNEUROSCI.4707-09.2009 CrossRef Google Scholar
- Rugg, M.D., & Vilberg, K.L. (2013). Brain networks underlying episodic memory retrieval. Current Opinion in Neurobiology, 23(2), 255–260. http://doi.org/10.1016/j.conb.2012.11.005 CrossRef Google Scholar
- Sadeh, T., Shohamy, D., Levy, D.R., Reggev, N., & Maril, A. (2011). Cooperation between the hippocampus and the striatum during episodic encoding. Journal of Cognitive Neuroscience, 23(7), 1597–1608. http://doi.org/10.1162/jocn.2010.21549 CrossRef Google Scholar
- Sager, M.A., Hermann, B., & La Rue, A. (2005). Middle-aged children of persons with Alzheimer’s disease: APOE genotypes and cognitive function in the Wisconsin Registry for Alzheimer’s Prevention. Journal of Geriatric Psychiatry and Neurology, 18(4), 245–249. http://doi.org/10.1177/0891988705281882 CrossRef Google Scholar PubMed
- Salami, A., Pudas, S., & Nyberg, L. (2014). Elevated hippocampal resting-state connectivity underlies deficient neurocognitive function in aging. Proceedings of the National Academy of Sciences of the United States of America, 111(49), 17654–17659. http://doi.org/10.1073/pnas.1410233111 CrossRef Google Scholar
- Schacter, D.L., Savage, C.R., Alpert, N.M., Rauch, S.L., & Albert, M.S. (1996). The role of hippocampus and frontal cortex in age-related memory changes: A PET study. Neuroreport, 7(6), 1165–1169. CrossRef Google Scholar PubMed
- Schedlbauer, A.M., Copara, M.S., Watrous, A.J., & Ekstrom, A.D. (2014). Multiple interacting brain areas underlie successful spatiotemporal memory retrieval in humans. Scientific Reports, 4, 6431. http://doi.org/10.1038/srep06431 CrossRef Google Scholar PubMed
- Schiavetto, A., Köhler, S., Grady, C.L., Winocur, G., & Moscovitch, M. (2002). Neural correlates of memory for object identity and object location: Effects of aging. Neuropsychologia, 40(8), 1428–1442. http://doi.org/10.1016/S0028-3932(01)00206-8 CrossRef Google Scholar
- Schmahmann, J.D., & Pandya, D.N. (2007). The complex history of the fronto-occipital fasciculus. Journal of the History of the Neurosciences, 16(4), 362–377. http://doi.org/10.1080/09647040600620468 CrossRef Google Scholar PubMed
- Schmidt, M. (1996). Rey Auditory Verbal Learning Test: RAVLT : A Handbook. Western Psychological Services. Retrieved from http://books.google.com/books/about/Rey_Auditory_Verbal_Learning_Test.html?id=UOcPRAAACAAJ&pgis=1 Google Scholar
- Staresina, B.P., Cooper, E., & Henson, R.N. (2013). Reversible information flow across the medial temporal lobe: The hippocampus links cortical modules during memory retrieval. The Journal of Neuroscience, 33(35), 14184–14192. http://doi.org/10.1523/JNEUROSCI.1987-13.2013 CrossRef Google Scholar
- Steffener, J., Habeck, C.G., & Stern, Y. (2012). Age-related changes in task related functional network connectivity. PLoS One, 7(9), e44421. http://doi.org/10.1371/journal.pone.0044421 CrossRef Google Scholar PubMed
- Takahashi, S., Yonezawa, H., Takahashi, J., Kudo, M., Inoue, T., & Tohgi, H. (2002). Selective reduction of diffusion anisotropy in white matter of Alzheimer disease brains measured by 3.0 Tesla magnetic resonance imaging. Neuroscience Letters, 332(1), 45–48. http://doi.org/S030439400200914X [pii]. CrossRef Google Scholar PubMed
- Tang, Y., Nyengaard, J.R., Pakkenberg, B., & Gundersen, H.J. (1997). Age-induced white matter changes in the human brain: a stereological investigation. Neurobiology of Aging, 18(6), 600–615. CrossRef Google Scholar PubMed
- Trenerry, M., Crosson, B., Deboe, J., & Leber, L. (1989). Stroop Neuropsychological Screening Test. Odessa, FL: Psychological Assessment Resources, Inc. Google Scholar
- Tulving, E. (1984). Precis of elements of episodic. The Behavioral and Brain Sciences, 7(2), 223–268. http://doi.org/http://dx.doi.org/10.1017/S0140525X0004440X CrossRef Google Scholar
- Vakil, E., Blachstein, H., & Soroker, N. (2004). Differential effect of right and left basal ganglionic infarctions on procedural learning. Cognitive and Behavioral Neurology, 17(2), 62–73. http://doi.org/10.1097/01.wnn.0000094553.44085.25 CrossRef Google Scholar
- Wakana, S., Jiang, H., Nagae-Poetscher, L.M., van Zijl, P.C.M., & Mori, S. (2004). Fiber tract-based atlas of human white matter anatomy. Radiology, 230(1), 77–87. http://doi.org/10.1148/radiol.2301021640 CrossRef Google Scholar
- Wang, Y., Gupta, A., Liu, Z., Zhang, H., Escolar, M.L., Gilmore, J.H., & Styner, M. (2011). DTI registration in atlas based fiber analysis of infantile Krabbe disease. Neuroimage, 55(4), 1577–1586. http://doi.org/10.1016/j.neuroimage.2011.01.038 CrossRef Google Scholar PubMed
- Watrous, A.J., Tandon, N., Conner, C.R., Pieters, T., & Ekstrom, A.D. (2013). Frequency-specific network connectivity increases underlie accurate spatiotemporal memory retrieval. Nature Neuroscience, 16(3), 349–356. http://doi.org/10.1038/nn.3315 CrossRef Google Scholar PubMed
- Williams, Z.M., & Eskandar, E.N. (2006). Selective enhancement of associative learning by microstimulation of the anterior caudate. Nature Neuroscience, 9(4), 562–568. http://doi.org/10.1038/nn1662 CrossRef Google Scholar PubMed
- Xu, G., McLaren, D.G., Ries, M.L., Fitzgerald, M.E., Bendlin, B.B., Rowley, H.A., & Johnson, S.C. (2009). The influence of parental history of Alzheimer’s disease and apolipoprotein E epsilon4 on the BOLD signal during recognition memory. Brain, 132(Pt 2), 383–391. http://doi.org/10.1093/brain/awn254 CrossRef Google Scholar PubMed
- Yin, H.H., Ostlund, S.B., Knowlton, B.J., & Balleine, B.W. (2005). The role of the dorsomedial striatum in instrumental conditioning. European Journal of Neuroscience, 22(2), 513–523. http://doi.org/10.1111/j.1460-9568.2005.04218.x CrossRef Google Scholar
- Yonelinas, A.P., Widaman, K., Mungas, D., Reed, B., Weiner, M.W., & Chui, H.C. (2007). Memory in the aging brain: Doubly dissociating the contribution of the hippocampus and entorhinal cortex. Hippocampus, 17(11), 1134–1140. http://doi.org/10.1002/hipo.20341 CrossRef Google Scholar
- Zahr, N.M., Rohlfing, T., Pfefferbaum, A., & Sullivan, E.V. (2009). Problem solving, working memory, and motor correlates of association and commissural fiber bundles in normal aging: A quantitative fiber tracking study. Neuroimage, 44(3), 1050–1062. http://doi.org/10.1016/j.neuroimage.2008.09.046 CrossRef Google Scholar
- Zhang, H., Avants, B.B., Yushkevich, P.A., Woo, J.H., Wang, S., McCluskey, L.F., & Gee, J.C. (2007). High-dimensional spatial normalization of diffusion tensor images improves the detection of white matter differences: An example study using amyotrophic lateral sclerosis. IEEE Transactions on Medical Imaging, 26(11), 1585–1597. http://doi.org/10.1109/TMI.2007.906784 CrossRef Google Scholar
- Zhang, H., Yushkevich, P.A., Alexander, D.C., & Gee, J.C. (2006). Deformable registration of diffusion tensor MR images with explicit orientation optimization. Medical Image Analysis, 10(5), 764–785. http://doi.org/10.1016/j.media.2006.06.004 CrossRef Google Scholar
- Zhang, H., Yushkevich, P.A., Rueckert, D., & Gee, J.C. (2007). Unbiased white matter atlas construction using diffusion tensor images. Medical Image Computing and Computer-Assisted Intervention, 10(Pt 2), 211–218. Google Scholar PubMed
- Zhuang, L., Sachdev, P.S., Trollor, J.N., Kochan, N.A., Reppermund, S., Brodaty, H., & Wen, W. (2012). Microstructural white matter changes in cognitively normal individuals at risk of amnestic MCI. Neurology, 79(8), 748–754. http://doi.org/10.1212/WNL.0b013e3182661f4d CrossRef Google Scholar PubMed
Cognitive impairment is common in Parkinson’s disease (PD). Three neurocognitive networks support efficient cognition: the salience network, the default mode network, and the central executive network. The salience network is thought to switch between activating and deactivating the default mode and central executive networks. Anti-correlated interactions between the salience and default mode networks in particular are necessary for efficient cognition. Our previous work demonstrated altered functional coupling between the neurocognitive networks in non-demented individuals with PD compared to age-matched control participants. Here, we aim to identify associations between cognition and functional coupling between these neurocognitive networks in the same group of participants.
We investigated the extent to which intrinsic functional coupling among these neurocognitive networks is related to cognitive performance across three neuropsychological domains: executive functioning, psychomotor speed, and verbal memory. Twenty-four non-demented individuals with mild to moderate PD and 20 control participants were scanned at rest and evaluated on three neuropsychological domains.
PD participants were impaired on tests from all three domains compared to control participants. Our imaging results demonstrated that successful cognition across healthy aging and Parkinson’s disease participants was related to anti-correlated coupling between the salience and default mode networks. Individuals with poorer performance scores across groups demonstrated more positive salience network/default-mode network coupling.
Successful cognition relies on healthy coupling between the salience and default mode networks, which may become dysfunctional in PD. These results can help inform non-pharmacological interventions (repetitive transcranial magnetic stimulation) targeting these specific networks before they become vulnerable in early stages of Parkinson’s disease. (JINS, 2016,22, 205–215)
- Aarsland, D., Andersen, K., Larsen, J.P., Lolk, A., & Kragh-Sorensen, P. (2003). Prevalence and characteristics of dementia in Parkinson disease: An 8-year prospective study. Archives of Neurology, 60(3), 387–392. CrossRef Google Scholar
- Barone, P., Aarsland, D., Burn, D., Emre, M., Kulisevsky, J., & Weintraub, D. (2011). Cognitive impairment in nondemented Parkinson’s disease. Movement Disorders, 26(14), 2483–2495. doi:10.1002/mds.23919 CrossRef Google Scholar PubMed
- Beckmann, C.F., Mackay, C.E., Filippini, N., & Smith, S.M. (2009). Group comparison of resting-state FMRI data using multi-subject ICA and dual regression. Paper presented at the OHBM. Google Scholar
- Bonnelle, V., Ham, T.E., Leech, R., Kinnunen, K.M., Mehta, M.A., Greenwood, R.J., && Sharp, D.J. (2012). Salience network integrity predicts default mode network function after traumatic brain injury. Proceedings of the National Academy of Sciences of the United States of America, 109(12), 4690–4695. doi:10.1073/pnas.1113455109 CrossRef Google Scholar PubMed
- Bosboom, J.L., Stoffers, D., & Wolters, E. (2004). Cognitive dysfunction and dementia in Parkinson’s disease. Journal of Neural Transmission, 111(10-11), 1303–1315. doi:10.1007/s00702-004-0168-1 CrossRef Google Scholar PubMed
- Bressler, S.L., & Menon, V. (2010). Large-scale brain networks in cognition: Emerging methods and principles. Trends in Cognitive Sciences, 14(6), 277–290. doi:10.1016/j.tics.2010.04.004 CrossRef Google Scholar PubMed
- Bronnick, K., Alves, G., Aarsland, D., Tysnes, O.B., & Larsen, J.P. (2011). Verbal memory in drug-naive, newly diagnosed Parkinson’s disease. The retrieval deficit hypothesis revisited. Neuropsychology, 25(1), 114–124. doi:10.1037/a0020857 CrossRef Google Scholar
- Buckner, R.L., Andrews-Hanna, J.R., & Schacter, D.L. (2008). The brain’s default network: Anatomy, function, and relevance to disease. Annals of the New York Academy of Sciences, 1124, 1–38. CrossRef Google Scholar
- Buddenberg, L.A., & Davis, C. (2000). Test-retest reliability of the Purdue Pegboard Test. The American Journal of Occupational Therapy, 54(5), 555–558. CrossRef Google Scholar PubMed
- Chikama, M., McFarland, N.R., Amaral, D.G., & Haber, S.N. (1997). Insular cortical projections to functional regions of the striatum correlate with cortical cytoarchitectonic organization in the primate. The Journal of Neuroscience, 17(24), 9686–9705. Google Scholar PubMed
- Chiong, W., Wilson, S.M., D’Esposito, M., Kayser, A.S., Grossman, S.N., Poorzand, P., & Rankin, K.P. (2013). The salience network causally influences default mode network activity during moral reasoning. Brain, 136(Pt 6), 1929–1941. doi:10.1093/brain/awt066 CrossRef Google Scholar PubMed
- Christopher, L., Duff-Canning, S., Koshimori, Y., Segura, B., Boileau, I., Chen, R., & Strafella, A.P. (2015). Salience network and parahippocampal dopamine dysfunction in memory-impaired Parkinson disease. Annals of Neurology, 77(2), 269–280. doi:10.1002/ana.24323 CrossRef Google Scholar PubMed
- Christopher, L., Koshimori, Y., Lang, A.E., Criaud, M., & Strafella, A.P. (2014). Uncovering the role of the insula in non-motor symptoms of Parkinson’s disease. Brain, 137, 2143–2154. doi:awu084 [pii] CrossRef Google Scholar PubMed
- Christopher, L., Marras, C., Duff-Canning, S., Koshimori, Y., Chen, R., Boileau, I., & Strafella, A.P. (2014). Combined insular and striatal dopamine dysfunction are associated with executive deficits in Parkinson’s disease with mild cognitive impairment. Brain, 137(Pt 2), 565–575. doi:awt337 [pii] CrossRef Google Scholar PubMed
- Cole, D.M., Beckmann, C.F., Long, C.J., Matthews, P.M., Durcan, M.J., & Beaver, J.D. (2010). Nicotine replacement in abstinent smokers improves cognitive withdrawal symptoms with modulation of resting brain network dynamics. Neuroimage, 52(2), 590–599. doi:10.1016/j.neuroimage.2010.04.251 CrossRef Google Scholar PubMed
- Dikmen, S.S., Heaton, R.K., Grant, I., & Temkin, N.R. (1999). Test-retest reliability and practice effects of expanded Halstead-Reitan Neuropsychological Test Battery. Journal of the International Neuropsychological Society, 5(4), 346–356. CrossRef Google Scholar PubMed
- Dirnberger, G., & Jahanshahi, M. (2013). Executive dysfunction in Parkinson’s disease: A review. Journal of Neuropsychology, 7(2), 193–224. doi:10.1111/jnp.12028 CrossRef Google Scholar PubMed
- Disbrow, E.A., Carmichael, O., He, J., Lanni, K.E., Dressler, E.M., Zhang, L., & Sigvardt, K.A. (2014). Resting state functional connectivity is associated with cognitive dysfunction in non-demented people with Parkinson’s disease. Journal of Parkinsons Disease, 4, 453–465. doi:1J0371M16R3R8714 [pii] Google Scholar
- Dosenbach, N.U., Visscher, K.M., Palmer, E.D., Miezin, F.M., Wenger, K.K., Kang, H.C., & Petersen, S.E. (2006). A core system for the implementation of task sets. Neuron, 50(5), 799–812. doi:S0896-6273(06)00349-7 [pii] CrossRef Google Scholar
- Doyen, A.L., & Carlier, M. (2002). Measuring handedness: A validation study of Bishop’s reaching card test. Laterality, 7(2), 115–130. doi:10.1080/13576500143000140 CrossRef Google Scholar
- Duan, X., Liao, W., Liang, D., Qiu, L., Gao, Q., Liu, C., & Chen, H. (2012). Large-scale brain networks in board game experts: Insights from a domain-related task and task-free resting state. PLoS One, 7(3), e32532. doi:10.1371/journal.pone.0032532 CrossRef Google Scholar PubMed
- Filippini, N., MacIntosh, B.J., Hough, M.G., Goodwin, G.M., Frisoni, G.B., Smith, S.M., & Mackay, C.E. (2009). Distinct patterns of brain activity in young carriers of the APOE-epsilon4 allele. Proceedings of the National Academy of Sciences of the United States of America, 106(17), 7209–7214. doi:0811879106 [pii]. CrossRef Google Scholar PubMed
- Foltynie, T., Brayne, C.E., Robbins, T.W., & Barker, R.A. (2004). The cognitive ability of an incident cohort of Parkinson’s patients in the UK. The CamPaIGN study. Brain, 127(Pt 3), 550–560. doi:10.1093/brain/awh067 CrossRef Google Scholar PubMed
- Fox, M.D., Snyder, A.Z., Vincent, J.L., Corbetta, M., Van Essen, D.C., & Raichle, M.E. (2005). The human brain is intrinsically organized into dynamic, anticorrelated functional networks. Proceedings of the National Academy of Sciences of the United States of America, 102(27), 9673–9678. CrossRef Google Scholar PubMed
- Fox, M.D., Snyder, A.Z., Vincent, J.L., & Raichle, M.E. (2007). Intrinsic fluctuations within cortical systems account for intertrial variability in human behavior. Neuron, 56(1), 171–184. doi:S0896-6273(07)00666-6 [pii] CrossRef Google Scholar PubMed
- Fox, P.T., Laird, A.R., Fox, S.P., Fox, P.M., Uecker, A.M., Crank, M., & Lancaster, J.L. (2005). BrainMap taxonomy of experimental design: Description and evaluation. Human Brain Mapping, 25(1), 185–198. doi:10.1002/hbm.20141 CrossRef Google Scholar PubMed
- Fransson, P., & Marrelec, G. (2008). The precuneus/posterior cingulate cortex plays a pivotal role in the default mode network: Evidence from a partial correlation network analysis. Neuroimage, 42(3), 1178–1184. CrossRef Google Scholar
- Fudge, J.L., Breitbart, M.A., Danish, M., & Pannoni, V. (2005). Insular and gustatory inputs to the caudal ventral striatum in primates. Journal of Comparitive Neurology, 490(2), 101–118. doi:10.1002/cne.20660 CrossRef Google Scholar PubMed
- Greicius, M.D., Krasnow, B., Reiss, A.L., & Menon, V. (2003). Functional connectivity in the resting brain: A network analysis of the default mode hypothesis. Proceedings of the National Academy of Sciences of the United States of America, 100(1), 253–258. doi:10.1073/pnas.0135058100 CrossRef Google Scholar PubMed
- Huang, C., Mattis, P., Tang, C., Perrine, K., Carbon, M., & Eidelberg, D. (2007). Metabolic brain networks associated with cognitive function in Parkinson’s disease. Neuroimage, 34(2), 714–723. doi:10.1016/j.neuroimage.2006.09.003 CrossRef Google Scholar PubMed
- Janes, A.C., Farmer, S., Frederick, B., Nickerson, L.D., & Lukas, S.E. (2014). An increase in tobacco craving is associated with enhanced medial prefrontal cortex network coupling. PLoS One, 9(2), e88228. doi:10.1371/journal.pone.0088228 CrossRef Google Scholar PubMed
- Janvin, C., Aarsland, D., Larsen, J.P., & Hugdahl, K. (2003). Neuropsychological profile of patients with Parkinson’s disease without dementia. Dementia and Geriatric Cognitive Disorders, 15(3), 126–131. doi:68483 CrossRef Google Scholar PubMed
- Janvin, C., Larsen, J.P., Aarsland, D., & Hugdahl, K. (2006). Subtypes of mild cognitive impairment in Parkinson’s disease: Progression to dementia. Movement Disorders, 21(9), 1343–1349. doi:10.1002/mds.20974 CrossRef Google Scholar
- Jilka, S.R., Scott, G., Ham, T., Pickering, A., Bonnelle, V., Braga, R.M., & Sharp, D.J. (2014). Damage to the salience network and interactions with the default mode network. Journal of Neuroscience, 34(33), 10798–10807. doi:10.1523/JNEUROSCI.0518-14.2014 CrossRef Google Scholar PubMed
- Kelly, A.M., Uddin, L.Q., Biswal, B.B., Castellanos, F.X., & Milham, M.P. (2008). Competition between functional brain networks mediates behavioral variability. Neuroimage, 39(1), 527–537. doi:10.1016/j.neuroimage.2007.08.008 CrossRef Google Scholar PubMed
- Kish, S.J., Shannak, K., & Hornykiewicz, O. (1988). Uneven pattern of dopamine loss in the striatum of patients with idiopathic Parkinson’s disease. Pathophysiologic and clinical implications. New England Journal of Medicine, 318(14), 876–880. doi:10.1056/NEJM198804073181402 CrossRef Google Scholar
- Klepac, N., Trkulja, V., Relja, M., & Babic, T. (2008). Is quality of life in non-demented Parkinson’s disease patients related to cognitive performance? A clinic-based cross-sectional study. European Journal of Neurology, 15(2), 128–133. doi:10.1111/j.1468-1331.2007.02011.x CrossRef Google Scholar
- Kortte, K.B., Horner, M.D., & Windham, W.K. (2002). The trail making test, part B: Cognitive flexibility or ability to maintain set? Applied Neuropsychology, 9(2), 106–109. doi:10.1207/S15324826AN0902_5 CrossRef Google Scholar
- Krajcovicova, L., Mikl, M., Marecek, R., & Rektorova, I. (2012). The default mode network integrity in patients with Parkinson’s disease is levodopa equivalent dose-dependent. Journal of Neural Transmission, 119(4), 443–454. doi:10.1007/s00702-011-0723-5 CrossRef Google Scholar PubMed
- Kudlicka, A., Clare, L., & Hindle, J.V. (2011). Executive functions in Parkinson’s disease: Systematic review and meta-analysis. Movement Disorders, 26(13), 2305–2315. doi:10.1002/mds.23868 CrossRef Google Scholar
- Laird, A.R., Fox, P.M., Eickhoff, S.B., Turner, J.A., Ray, K.L., McKay, D.R., & Fox, P.T. (2011). Behavioral interpretations of intrinsic connectivity networks. Journal of Cognitive Neuroscience, 23(12), 4022–4037. doi:10.1162/jocn_a_00077 CrossRef Google Scholar
- Laird, A.R., Lancaster, J.L., & Fox, P.T. (2005). BrainMap: The social evolution of a human brain mapping database. Neuroinformatics, 3(1), 65–78. doi:NI:3:1:065 [pii] CrossRef Google Scholar PubMed
- Lakens, D. (2013). Calculating and reporting effect sizes to facilitate cumulative science: A practical primer for t-tests and ANOVAs. Frontiers in Psychology, 4, 863. doi:10.3389/fpsyg.2013.00863 CrossRef Google Scholar PubMed
- Leech, R., & Sharp, D.J. (2014). The role of the posterior cingulate cortex in cognition and disease. Brain, 137(Pt 1), 12–32. doi:10.1093/brain/awt162 CrossRef Google Scholar PubMed
- Lewis, S.J., Cools, R., Robbins, T.W., Dove, A., Barker, R.A., & Owen, A.M. (2003). Using executive heterogeneity to explore the nature of working memory deficits in Parkinson’s disease. Neuropsychologia, 41(6), 645–654. CrossRef Google Scholar PubMed
- Lewis, S.J., Dove, A., Robbins, T.W., Barker, R.A., & Owen, A.M. (2003). Cognitive impairments in early Parkinson’s disease are accompanied by reductions in activity in frontostriatal neural circuitry. The Journal of Neuroscience, 23(15), 6351–6356. doi:23/15/6351 [pii] Google Scholar PubMed
- Lewis, S.J., Slabosz, A., Robbins, T.W., Barker, R.A., & Owen, A.M. (2005). Dopaminergic basis for deficits in working memory but not attentional set-shifting in Parkinson’s disease. Neuropsychologia, 43(6), 823–832. doi:S0028-3932(04)00266-0 [pii] CrossRef Google Scholar
- Mamikonyan, E., Moberg, P.J., Siderowf, A., Duda, J.E., Have, T.T., Hurtig, H.I., & Weintraub, D. (2009). Mild cognitive impairment is common in Parkinson’s disease patients with normal Mini-Mental State Examination (MMSE) scores. Parkinsonism & Related Disorders, 15(3), 226–231. doi:10.1016/j.parkreldis.2008.05.006 CrossRef Google Scholar PubMed
- Martinu, K., Degroot, C., Madjar, C., Strafella, A.P., & Monchi, O. (2012). Levodopa influences striatal activity but does not affect cortical hyper-activity in Parkinson’s disease. European Journal of Neuroscience, 35, 572–583. doi:10.1111/j.1460-9568.2011 CrossRef Google Scholar
- Menon, V. (2011). Large-scale brain networks and psychopathology: A unifying triple network model. Trends in Cognitive Sciences, 15(10), 483–506. doi:S1364-6613(11)00171-9 [pii] CrossRef Google Scholar PubMed
- Menon, V., & Uddin, L.Q. (2010). Saliency, switching, attention and control: A network model of insula function. Brain Structure & Function, 214(5-6), 655–667. doi:10.1007/s00429-010-0262-0 CrossRef Google Scholar PubMed
- Middleton, F.A., & Strick, P.L. (2000). Basal ganglia and cerebellar loops: Motor and cognitive circuits. Brain Research. Brain Research Reviews, 31(2-3), 236–250. doi:S0165017399000405 [pii] CrossRef Google Scholar PubMed
- Miller, I.N., Neargarder, S., Risi, M.M., & Cronin-Golomb, A. (2013). Frontal and posterior subtypes of neuropsychological deficit in Parkinson’s disease. Behavioral Neuroscience, 127(2), 175–183. doi:10.1037/a0031357 CrossRef Google Scholar PubMed
- Mitrushina, M., Satz, P., Chervinsky, A., & D’Elia, L. (1991). Performance of four age groups of normal elderly on the Rey Auditory-Verbal Learning Test. Journal of Clinical Psychology, 47(3), 351–357.3.0.CO;2-S>CrossRef Google Scholar PubMed
- Monchi, O., Petrides, M., Mejia-Constain, B., & Strafella, A.P. (2006). Cortical activity in Parkinson’s disease during executive processing depends on striatal involvement. Brain, 130(Pt 1), 233–244. doi:awl326 [pii] CrossRef Google Scholar PubMed
- Moustafa, A.A., Krishna, R., Eissa, A.M., & Hewedi, D.H. (2013). Factors underlying probabilistic and deterministic stimulus-response learning performance in medicated and unmedicated patients with Parkinson’s disease. Neuropsychology, 27(4), 498–510. doi:2013-25138-010 [pii] CrossRef Google Scholar
- Narayanan, N.S., Rodnitzky, R.L., & Uc, E.Y. (2013). Prefrontal dopamine signaling and cognitive symptoms of Parkinson’s disease. Reviews in the Neurosciences, 24(3), 267–278. doi:10.1515/revneuro-2013-0004 CrossRef Google Scholar
- Poletti, M., & Bonuccelli, U. (2013). Acute and chronic cognitive effects of levodopa and dopamine agonists on patients with Parkinson’s disease: A review. Therapeutic Advances in Psychopharmacology, 3(2), 101–113. doi:10.1177/2045125312470130 CrossRef Google Scholar PubMed
- Postuma, R.B., & Dagher, A. (2006). Basal ganglia functional connectivity based on a meta-analysis of 126 positron emission tomography and functional magnetic resonance imaging publications. Cerebral Cortex, 16(10), 1508–1521. doi:bhj088 [pii] CrossRef Google Scholar PubMed
- Putcha, D., Ross, R.S., Cronin-Golomb, A., Janes, A.C., & Stern, C.E. (2015). Altered intrinsic functional coupling between core neurocognitive networks in Parkinson’s disease. Neuroimage. Clinical, 7, 449–455. doi:10.1016/j.nicl.2015.01.012 CrossRef Google Scholar PubMed
- Raichle, M.E., MacLeod, A.M., Snyder, A.Z., Powers, W.J., Gusnard, D.A., & Shulman, G.L. (2001). A default mode of brain function. Proceedings of the National Academy of Sciences of the United States of America, 98(2), 676–682. CrossRef Google Scholar PubMed
- Ravina, B., Marek, K., Eberly, S., Oakes, D., Kurlan, R., Ascherio, A., & Shoulson, I. (2012). Dopamine transporter imaging is associated with long-term outcomes in Parkinson’s disease. Movement Disorders, 27(11), 1392–1397. doi:10.1002/mds.25157 CrossRef Google Scholar
- Rey, A. (1964). L’examen clinique en psychologie. Paris: Presses Universitaires de France. Google Scholar
- Schendan, H.E., Tinaz, S., Maher, S.M., & Stern, C.E. (2013). Frontostriatal and mediotemporal lobe contributions to implicit higher-order spatial sequence learning declines in aging and Parkinson’s disease. Behavioral Neuroscience, 127(2), 204–221. doi:2013-11369-003 [pii] CrossRef Google Scholar PubMed
- Seeley, W.W., Menon, V., Schatzberg, A.F., Keller, J., Glover, G.H., Kenna, H., & Greicius, M.D. (2007). Dissociable intrinsic connectivity networks for salience processing and executive control. The Journal of Neuroscience, 27(9), 2349–2356. doi:27/9/2349 [pii] CrossRef Google Scholar PubMed
- Spreng, R.N., Mar, R.A., & Kim, A.S. (2009). The common neural basis of autobiographical memory, prospection, navigation, theory of mind, and the default mode: A quantitative meta-analysis. Journal of Cognitive Neuroscience, 21(3), 489–510. doi:10.1162/jocn.2008.21029 CrossRef Google Scholar
- Sridharan, D., Levitin, D.J., & Menon, V. (2008). A critical role for the right fronto-insular cortex in switching between central-executive and default-mode networks. Proceedings of the National Academy of Sciences of the United States of America, 105(34), 12569–12574. doi:0800005105 [pii] CrossRef Google Scholar
- Strauss, E., Sherman, E., & Spreen, O. (2006). A compendium of neuropsychological tests: Administration, norms, and commentaryed (3rd ed.). Cambridge: Oxford University Press. Google Scholar
- Tessitore, A., Esposito, F., Vitale, C., Santangelo, G., Amboni, M., Russo, A., & Tedeschi, G. (2012). Default-mode network connectivity in cognitively unimpaired patients with Parkinson disease. Neurology, 79(23), 2226–2232. doi:WNL.0b013e31827689d6 [pii] CrossRef Google Scholar PubMed
- Tiffin, J., & Asher, E.J. (1948). The Purdue pegboard; Norms and studies of reliability and validity. Journal of Applied Psychology, 32(3), 234–247. CrossRef Google Scholar
- Tinaz, S., Lauro, P., Hallett, M., & Horovitz, S.G. (2015). Deficits in task-set maintenance and execution networks in Parkinson’s disease. Brain Structure & Function. doi:10.1007/s00429-014-0981-8 Google Scholar
- Tinaz, S., Schendan, H.E., & Stern, C.E. (2008). Fronto-striatal deficit in Parkinson’s disease during semantic event sequencing. Neurobiology of Aging, 29(3), 397–407. doi:S0197-4580(06)00400-3 [pii] CrossRef Google Scholar PubMed
- Tombaugh, T.N. (2004). Trail Making Test A and B: Normative data stratified by age and education. Archives of Clinical Neuropsychology, 19(2), 203–214. doi:10.1016/S0887-6177(03)00039-8 CrossRef Google Scholar
- Uc, E.Y., McDermott, M.P., Marder, K.S., Anderson, S.W., Litvan, I., Como, P.G., … Parkinson Study Group DATATOP Investigators. (2009). Incidence of and risk factors for cognitive impairment in an early Parkinson disease clinical trial cohort. Neurology, 73(18), 1469–1477. doi:10.1212/WNL.0b013e3181bf992f CrossRef Google Scholar
- Uc, E.Y., Rizzo, M., Anderson, S.W., Qian, S., Rodnitzky, R.L., & Dawson, J.D. (2005). Visual dysfunction in Parkinson disease without dementia. Neurology, 65(12), 1907–1913. doi:01.wnl.0000191565.11065.11 [pii] CrossRef Google Scholar
- van Eimeren, T., Monchi, O., Ballanger, B., & Strafella, A.P. (2009). Dysfunction of the default mode network in Parkinson disease: A functional magnetic resonance imaging study. Archives of Neurology, 66(7), 877–883. doi:66/7/877 [pii] CrossRef Google Scholar PubMed
- Weissman, D.H., Roberts, K.C., Visscher, K.M., & Woldorff, M.G. (2006). The neural bases of momentary lapses in attention. Nature Neuroscience, 9(7), 971–978. doi:10.1038/nn1727 CrossRef Google Scholar PubMed
- Williams-Gray, C.H., Evans, J.R., Goris, A., Foltynie, T., Ban, M., Robbins, T.W., & Barker, R.A. (2009). The distinct cognitive syndromes of Parkinson’s disease: 5 year follow-up of the CamPaIGN cohort. Brain, 132(Pt 11), 2958–2969. doi:awp245 [pii] CrossRef Google Scholar PubMed
Processing speed impairment is the most prevalent cognitive deficit in individuals with multiple sclerosis (MS). However, the neural mechanisms associated with processing speed remain under debate. The current investigation provides a dynamic representation of the functioning of the brain network involved in processing speed by examining effective connectivity pattern during a processing speed task in healthy adults and in MS individuals with and without processing speed impairment.
Group assignment (processing speed impairedvs. intact) was based on participants’ performance on the Symbol Digit Modalities test (Parmenter, Testa, Schretlen, Weinstock-Guttman, & Benedict,2010). First, brain regions involved in the processing speed task were determined in healthy participants. Time series from these functional regions of interest of each group of participants were then subjected to the effective connectivity analysis (Independent Multiple-Sample Greedy Equivalence Search and Linear, Non-Gaussian Orientation, Fixed Structure algorithms) that showed causal influences of one region on another during task performance.
The connectivity pattern of the processing speed impaired group was significantly different from the connectivity pattern of the processing speed intact group and of the healthy control group. Differences in the strength of common connections were also observed.
Effective connectivity results reveal that MS individuals with processing speed impairment not only have connections that differ from healthy participants and MS individuals without processing speed impairment, but also have increased strengths of connections. (JINS, 2016,22, 216–224)
- Barker-Collo, S.L. (2006). Quality of life in multiple sclerosis: Does information-processing speed have an independent effect? Archives of Clinical Neuropsychology, 21(2), 167–174. http://doi.org/10.1016/j.acn.2005.08.008 CrossRef Google Scholar PubMed
- Bester, M., Lazar, M., Petracca, M., Babb, J.S., Herbert, J., Grossman, R.I., & Inglese, M. (2013). Tract-specific white matter correlates of fatigue and cognitive impairment in benign multiple sclerosis. Journal of the Neurological Sciences, 330(1-2), 61–66. http://doi.org/10.1016/j.jns.2013.04.005 CrossRef Google Scholar
- Bledowski, C., Kaiser, J., & Rahm, B. (2010). Basic operations in working memory: Contributions from functional imaging studies. Behavioural Brain Research, 214(2), 172–179. http://doi.org/10.1016/j.bbr.2010.05.041 CrossRef Google Scholar PubMed
- Chiaravalloti, N.D., Stojanovic-Radic, J., & DeLuca, J. (2013). The role of speed versus working memory in predicting learning new information in multiple sclerosis. Journal of Clinical and Experimental Neuropsychology, 35(2), 180–191. http://doi.org/10.1080/13803395.2012.760537 CrossRef Google Scholar
- Compston, A., & Coles, A. (2008). Multiple sclerosis. Lancet, 372(9648), 1502–1517. http://doi.org/10.1016/S0140-6736(08)61620-7 CrossRef Google Scholar
- Cox, R.W. (1996). AFNI: Software for analysis and visualization of functional magnetic resonance neuroimages. Computers and Biomedical Research, 29(3), 162–173. CrossRef Google Scholar PubMed
- Denney, D.R., & Lynch, S.G. (2009). The impact of multiple sclerosis on patients’ performance on the Stroop Test: Processing speed versus interference. Journal of the International Neuropsychological Society, 15(3), 451–458. http://doi.org/10.1017/S1355617709090730 CrossRef Google Scholar
- Deshpande, G., & Hu, X. (2012). Investigating effective brain connectivity from fMRI data: Past findings and current issues with reference to Granger causality analysis. Brain Connectivity, 2(5), 235–245. http://doi.org/10.1089/brain.2012.0091 CrossRef Google Scholar
- Forn, C., Belloch, V., Bustamante, J.C., Garbin, G., Parcet-Ibars, M.A., Sanjuan, A., & Avila, C. (2009). A symbol digit modalities test version suitable for functional MRI studies. Neuroscience Letters, 456, 11–14. CrossRef Google Scholar
- Friston, K.J. (1994). Functional and effective connectivity in neuroimaging: A synthesis. Human Brain Mapping, 2(1-2), 56–78. http://doi.org/10.1002/hbm.460020107 CrossRef Google Scholar
- Genova, H.M., DeLuca, J., Chiaravalloti, N., & Wylie, G. (2013). The relationship between executive functioning, processing speed, and white matter integrity in multiple sclerosis. Journal of Clinical and Experimental Neuropsychology, 35(6), 631–641. http://doi.org/10.1080/13803395.2013.806649 CrossRef Google Scholar
- Genova, H.M., Hillary, F.G., Wylie, G., Rypma, B., & Deluca, J. (2009). Examination of processing speed deficits in multiple sclerosis using functional magnetic resonance imaging. Journal of the International Neuropsychological Society, 15(3), 383–393. http://doi.org/10.1017/S1355617709090535 CrossRef Google Scholar PubMed
- Genova, H.M., Lengenfelder, J, Chiaravalloti, N.D, Moore, N.B. & DeLuca, J. (2012). Processing speed versus working memory: contributions to an information-processing task in multiple sclerosis. Appl. Neuropsychol. Adult, 19, 132–140. CrossRef Google Scholar
- Kravits, D.J., Saleem, K.S., Baker, C.I., & Mishkin, M. (2012). A new neural framework for visuospatial processing. Nature Review Neuroscience, 12(4), 217–230. http://doi.org/10.1038/nrn3008.A CrossRef Google Scholar
- Larocque, J.J., Lewis-peacock, J.A., & Postle, B.R. (2014). Multiple neural states of representation in short-term memory? It’s a matter of attention. Frontiers in Human Neuroscience, 8(January), 1–14. http://doi.org/10.3389/fnhum.2014.00005 CrossRef Google Scholar
- Leavitt, V.M., Wylie, G., Genova, H.M., Chiaravalloti, N., & DeLuca, J. (2012). Altered effective connectivity during performance of an information processing speed task in multiple sclerosis. Multiple Sclerosis (Houndmills, Basingstoke, England), 18(4), 409–417. http://doi.org/10.1177/1352458511423651 CrossRef Google Scholar PubMed
- Lee, P., Li, P.-C., Liu, C.-H., & Hsieh, C.-L. (2011). Test-retest reliability of two attention tests in schizophrenia. Archives of Clinical Neuropsychology, 26(5), 405–411. http://doi.org/10.1093/arclin/acr038 CrossRef Google Scholar
- Luckmann, H.C., Jacobs, H.I.L., Sack, A.T., & Lu, H.C. (2014). The cross-functional role of frontoparietal regions in cognition: Internal attention as the overarching mechanism. Progress in Neurobiology, 116, 66–86. CrossRef Google Scholar PubMed
- McDonald, W.I., Compston, A., Edan, G., Goodkin, D., Hartung, H.P., Lublin, F.D., & Wolinsky, J.S. (2001). Recommended diagnostic criteria for multiple sclerosis: Guidelines from the International Panel on the Diagnosis of Multiple Sclerosis. Annals of Neurology, 50, 121–127. http://doi.org/10.1002/ana.1032 CrossRef Google Scholar
- Miller, G.A., & Chapman, J.P. (2001). Misunderstanding analysis of covariance. Journal of Abnormal Psychology, 110(1), 40–48. http://doi.org/10.1037/0021-843X.110.1.40 CrossRef Google Scholar PubMed
- Mumford, J.A., & Ramsey, J.D. (2014). Bayesian networks for fMRI: A primer. Neuroimage, 86, 573–582. CrossRef Google Scholar PubMed
- Papadopoulou, A., Müller-Lenke, N., Naegelin, Y., Kalt, G., Bendfeldt, K., Kuster, P., & Penner, I.-K. (2013). Contribution of cortical and white matter lesions to cognitive impairment in multiple sclerosis. Multiple Sclerosis (Houndmills, Basingstoke, England), 19(10), 1290–1296. http://doi.org/10.1177/1352458513475490 CrossRef Google Scholar PubMed
- Parmenter, B.A., Testa, S.M., Schretlen, D.J., Weinstock-Guttman, B., & Benedict, R.H. (2010). The utility of regression-based norms in interpreting the minimal assessment of cognitive function in multiple sclerosis (MACFIMS). Journal of the International Neuropsychological Society, 16(1), 6–16. http://doi.org/10.1017/S1355617709990750 CrossRef Google Scholar
- Poldrack, R.A. (2007). Region of interest analysis for fMRI. Social Cognitive and Affective Neuroscience, 2(1), 67–70. http://doi.org/10.1093/scan/nsm006 CrossRef Google Scholar
- Poldrack, R.A., Mumford, J.A., & Nichols, T.E. (2011). Handbook of functional MRI: Data analysis. New York, NY: Cambridge University Press. CrossRef Google Scholar
- Ramsey, J.D., Hanson, S.J., & Glymour, C. (2011). Multi-subject search correctly identifies causal connections and most causal directions in the DCM models of the Smith et al. simulation study. Neuroimage, 58(3), 838–848. http://doi.org/10.1016/j.neuroimage.2011.06.068 CrossRef Google Scholar
- Ramsey, J.D., Hanson, S.J., Hanson, C., Halchenko, Y.O., Poldrack, R.A., & Glymour, C. (2010). Six problems for causal inference from fMRI. Neuroimage, 49(2), 1545–1558. http://doi.org/10.1016/j.neuroimage.2009.08.065 CrossRef Google Scholar
- Ramsey, J.D., Sanchez-romero, R., & Glymour, C. (2014). Non-Gaussian methods and high-pass filters in the estimation of effective connections. Neuroimage, 84, 986–1006. http://doi.org/10.1016/j.neuroimage.2013.09.062 CrossRef Google Scholar
- Sheridan, L.K., Fitzgerald, H.E., Adams, K.M., Nigg, J.T., Martel, M.M., Puttler, L.I., & Zucker, R.A. (2006). Normative Symbol Digit Modalities Test performance in a community-based sample. Archives of Clinical Neuropsychology, 21(1), 23–28. http://doi.org/10.1016/j.acn.2005.07.003 CrossRef Google Scholar
- Shomstein, S. (2012). Cognitive functions of the posterior parietal cortex: Top-down and bottom-up attentional control. Frontiers in Integrative Neuroscience, 6(July), 38. http://doi.org/10.3389/fnint.2012.00038 CrossRef Google Scholar PubMed
- Smith, A. (1982). Symbol Digit Modalities Test (SDMT): Manual (revised). Los Angeles: Western Psychological Services. Google Scholar
- Smith, S.M., Bandettini, P.A., Miller, K.L., Behrens, T.E.J., Friston, K.J., David, O., & Nichols, T.E. (2012). The danger of systematic bias in group-level FMRI-lag-based causality estimation. Neuroimage, 59(2), 1228–1229. http://doi.org/10.1016/j.neuroimage.2011.08.015 CrossRef Google Scholar PubMed
- Smith, S.M., Miller, K.L., Salimi-Khorshidi, G., Webster, M., Beckmann, C.F., Nichols, T.E., & Woolrich, M.W. (2011). Network modelling methods for FMRI. Neuroimage, 54(2), 875–891. http://doi.org/10.1016/j.neuroimage.2010.08.063 CrossRef Google Scholar PubMed
- Strober, L.B., Christodoulou, C., Benedict, R.H.B., Westervelt, H.J., Melville, P., Scherl, W.F., & Krupp, L.B. (2012). Unemployment in multiple sclerosis: The contribution of personality and disease. Multiple Sclerosis (Houndmills, Basingstoke, England), 18(5), 647–653. http://doi.org/10.1177/1352458511426735 CrossRef Google Scholar
- Van Schependom, J., D’hooghe, M.B., Cleynhens, K., D’hooge, M., Haelewyck, M.-C., De Keyser, J., & Nagels, G. (2015). Reduced information processing speed as primum movens for cognitive decline in MS. Multiple Sclerosis (Houndmills, Basingstoke, England), 21, 83–91. http://doi.org/10.1177/1352458514537012 CrossRef Google Scholar PubMed
There is a well-known association between memory impairment and major depressive disorder (MDD). Additionally, recent studies are also showing resting-state functional magnetic resonance imaging (rsMRI) abnormalities in active and remitted MDD. However, no studies to date have examined both rs connectivity and memory performance in early course remitted MDD, nor the relationship between connectivity and semantically cued episodic memory.
The rsMRI data from two 3.0 Tesla GE scanners were collected from 34 unmedicated young adults with remitted MDD (rMDD) and 23 healthy controls (HCs) between 18 and 23 years of age using bilateral seeds in the hippocampus. Participants also completed a semantically cued list-learning test, and their performance was correlated with hippocampal seed-based rsMRI. Regression models were also used to predict connectivity patterns from memory performance.
After correcting for sex, rMDD subjects performed worse than HCs on the total number of words recalled and recognized. rMDD demonstrated significant in-network hypoactivation between the hippocampus and multiple fronto-temporal regions, and multiple extra-network hyperconnectivities between the hippocampus and fronto-parietal regions when compared to HCs. Memory performance negatively predicted connectivity in HCs and positively predicted connectivity in rMDD.
Even when individuals with a history of MDD are no longer displaying active depressive symptoms, they continue to demonstrate worse memory performance, disruptions in hippocampal connectivity, and a differential relationship between episodic memory and hippocampal connectivity. (JINS, 2016,22, 225–239)
- Anand, A., Li, Y., Wang, Y., Wu, J., Gao, S., Bukhari, L., & Lowe, M.J. (2005). Activity and connectivity of brain mood regulating circuit in depression: A functional magnetic resonance study. Biological Psychiatry, 57, 1079–1088. CrossRef Google Scholar PubMed
- Beck, A.T., Steer, R.A., & Brown, G.K. (1996). Manual for the Beck Depression Inventory II. San Antonio, TX: Psychology Corporation. Google Scholar
- Behnken, A., Schöning, S., Gerβ, J., Konrad, C., de Jong-Meyer, R., Zwanzger, P., && Arolt, V. (2010). Persistent non-verbal memory impairment in remitted major depression. Journal of Affective Disorders, 122(1-2), 144–148. CrossRef Google Scholar
- Benton, A., Hamsher, K., Varney, N., & Spreen, O. (1978). Contributions to neuropsychological assessment: A clinical manual. New York: Oxford University Press. Google Scholar
- Brett, M., Anton, J.-L., Valabregue, R., & Poline, J.-P. (2002). Region of interest analysis using an SPM toolbox. Poster presented at the 8th International Conference on Functional Mapping of the Human Brain, Sendai, Japan. Google Scholar
- Briceño, E.M., Weisenbach, S.L., Rapport, L.J., Hazlett, K.E., Bieliauskas, L.A., Haase, B.D., & Langenecker, S.A. (2013). Shifted inferior frontal laterality in women with Major Depressive Disorder is related to emotion processing deficits. Psychological Medicine, 43(7), 1433–1445. CrossRef Google Scholar PubMed
- Bora, E., Harrison, B.J., Yücel, M., & Pantelis, C. (2013). Cognitive impairment in euthymic major depressive disorder: A meta-analysis. Psychological Medicine, 43, 2017–2026. CrossRef Google Scholar PubMed
- Brown, J.A. (1958). Some tests of the decay theory of immediate memory. Quarterly Journal of Experimental Psychology, 10, 12–21. CrossRef Google Scholar
- Burt, D.B., Zembar, M.J., & Niederehe, G. (1995). Depression and memory impairment: A meta-analysis of the association, its pattern, and specificity. Psychological Bulletin, 117(2), 285–305. CrossRef Google Scholar PubMed
- Butters, M., Whyte, E., Nebes, R., Begley, A., Dew, M., Mulsant, B., & Becker, J. (2004). The nature and determinants of neuropsychological functioning in late-life depression. Archives of General Psychiatry, 61, 587–595. CrossRef Google Scholar
- Connolly, C.G., Wu, J., Ho, T.C., Hoeft, F., Wolkowitz, O., Eisendrath, S., & Yang, T.T. (2013). Resting-state functional connectivity of subgenual anterior cingulate cortex in depressed adolescents. Biological Psychiatry, 74, 898–907. CrossRef Google Scholar PubMed
- Considine, C.M., Weisenbach, S.L., Walker, S.J., McFadden, E.M., Franti, L.M., Bieliauskas, L.A., & Langenecker, S.A. (2011). Auditory memory decrements, without dissimulation, among patents with major depressive disorder. Archives of Clinical Neuropsychology, 26, 445–453. CrossRef Google Scholar
- Delis, D., Kramer, J., Kaplan, E., & Ober, B. (2000). California Verbal Learning Test - II. San Antonio, TX: The Psychological Corporation. Google Scholar
- Elderkin-Thompson, V., Moody, T., Knowlton, B., Hellemann, G., & Kumar, A. (2011). Explicit and implicit memory in late-life depression. The American Journal of Geriatric Psychiatry, 19(4), 364–373. CrossRef Google Scholar
- Frodl, T., Bokde, A.L.W., Scheuerecker, J., Lisiecka, D., Schoepf, V., Hampel, H., & Meisenzahl, E. (2010). Functional connectivity bias of the orbitofrontal cortex in drug-free patients with major depression. Biological Psychiatry, 67, 161–167. CrossRef Google Scholar
- Grant, D.A., & Berg, E. (1948). A behavioral analysis of degree of reinforcement and ease of shifting to new responses in a Weigl-type card-sorting problem. Journal of Experimental Psychology, 38, 404. CrossRef Google Scholar
- Greicius, M.D., Flores, B.H., Menon, V., Glover, G.H., Solvason, H.B., Kenna,& Schatzberg, A.F. (2007). Resting-state functional connectivity in major depression: Abnormally increased contributions from subgenual cingulate cortex and thalamus. Biological Psychiatry, 62, 429–437. CrossRef Google Scholar
- Hamilton, J.P., Chen, G., Thomason, M.E., Schwartz, M.E., & Gottlib, I.H. (2011). Investigating neural primacy in Major Depressive Disorder: Multivariate granger causality analysis of resting-state fMRI time-series data. Molecular Psychiatry, 16(7), 763–772. CrossRef Google Scholar PubMed
- Hamilton, M. (1960). A rating scale for depression. Journal of Neurology and Neurosurgery in Psychiatry, 23, 56–62. CrossRef Google Scholar
- Hasselbalch, B.J., Knorr, U., & Kessing, L.V. (2011). Cognitive impairment in the remitted state of unipolar depressive disorder: A systematic review. Journal of Affective Disorders, 134(1-3), 20–31. CrossRef Google Scholar PubMed
- Hermens, D.F., Naismith, S.L., Redoblado-Hodge, M.A., Scott, E.M., & Hickie, I.B. (2010). Impaired verbal memory in young adults with unipolar and bipolar depression. Early Intervention in Psychiatry, 3, 227–233. CrossRef Google Scholar
- Jacobs, R.H., Jenkins, L.M., Gabriel, L.B., Barba, A., Ryan, K.A., Weisenbach, S.L., & Welsh, R.C. (2014). Increased coupling of intrinsic networks in remitted depressed youth predicts ruminations and cognitive control. PLoS One, 9(8), e104366. CrossRef Google Scholar
- Kassel, M.T., Rao, J.A., Walker, S.J., Briceño, E.M., Gabriel, L.B., Welson, A.L., & Langenecker, S.A. (2015). Decreased fronto-limbic activation and disrupted semantic cued list learning in Major Depressive Disorder. Journal of the International Neuropsychological Society, in press. doi: 10.1017/S1355617716000023. Google Scholar
- Kramer, J.H., Yaffe, K., Lengenfelder, J., & Delis, D.C. (2003). Age and gender interactions on verbal memory performance. Journal of the International Neuropsychological Society, 9(1), 97–102. CrossRef Google Scholar PubMed
- Langenecker, S.A., Caveney, A.F., Persad, C.P., & Giordani, B. (2004). Semantic list learning test. Ann Arbor, MI: University of Michigan. Google Scholar
- Langenecker, S.A., Weisenbach, S.L., Giordani, B., Briceño, E.M., Guidotti Breting, L.M., Schallmo, M.P., & Starkman, M.N. (2012). Impact of chronic hypercortisolemia on affective processing. Neuropharmacology, 62, 217–225. CrossRef Google Scholar
- Lewin, C., Wolgers, G., & Herlitz, A. (2001). Sex differences favoring women in verbal but not in visuospatial episodic memory. Neuropsychology, 15(2), 165–173. CrossRef Google Scholar PubMed
- Lezak, M. (1995). Neuropsychological assessment (3rd ed.), New York: Oxford University Press. Google Scholar PubMed
- Liu, S., Wu, W., Qiu, L., Yang, X., Kuang, W., Chan, R.C.K., & Gong, Q. (2011). Resting-state functional connectivity treatment-resistant depression. American Journal of Psychiatry, 168, 642–648. Google Scholar
- Liu, X., Li, L., Xiao, J., Yang, J., & Jiang, X. (2013). Abnormalities of autobiographical memory of patients with depressive disorders: A meta-analysis. Psychology and Psychotherapy: Theory, Research and Practice, 86(4), 353–373. CrossRef Google Scholar
- Marcos, T., Salamero, M., Gutierrez, F., Catalan, R., Gasto, C., & Lazaro, L. (1994). Cognitive dysfunctions in recovered melancholic patients. Journal of Affective Disorders, 32, 133–137. CrossRef Google Scholar PubMed
- Mulligan, N.W. (2011). Implicit memory and depression: Preserved conceptual priming in subclinical depression. Cognition and Emotion, 25(4), 730–739. CrossRef Google Scholar
- Paelecke-Habermann, Y., Pohl, J., & Leplow, B. (2005). Attention and executive functions in remitted major depression patients. Journal of Affective Disorders, 89(1-3), 125–135. CrossRef Google Scholar
- Papez, J.W. (1937). A proposed mechanism of emotion. Archives of Neurology and Psychiatry, 7, 103–112. Google Scholar PubMed
- Pasterski, V., Zwierzynska, K., & Estes, Z. (2011). Sex differences in semantic categorization. Archives of Sexual Behavior, 40(6), 1183–1187. CrossRef Google Scholar PubMed
- Peters, A.T., Jacobs, R.H., Crane, N.A., Ryan, K.A., Weisenbach, S.L., Ajilore, O., & Langenecker, S.A. (2015). Domain-specific impairment in cognitive control among remitted youth with a history of major depression. Early Intervention in Psychiatry. [Epub ahead of print]. CrossRef Google Scholar PubMed
- Peterson, L.R., & Peterson, M.J. (1959). Short-term retention of individual verbal items. Journal of Experimental Psychology, 58, 193–198. CrossRef Google Scholar PubMed
- Porter, R.J., Gallagher, P., Thompson, J.M., & Young, A.H. (2003). Neurocognitive impairment in drug-free patients with Major Depressive Disorder. British Journal of Psychiatry, 182, 214–220. CrossRef Google Scholar PubMed
- Reitan, R.M. (1992). Trail Making Test: Manual for administration and scoring. Mesa, AZ: Reitan Neuropsychology Laboratory. Google Scholar
- Rohling, M., Green, P., Allen, L., & Iverson, G. (2002). Depressive symptoms and neurocognitive test scores in patients passing symptom validity tests. Archives of Clinical Neuropsychology, 17, 205–222. CrossRef Google Scholar PubMed
- Schallmo, M.P., Kassel, M.T., Weisenbach, S.L., Walker, S.J., Guidotti-Breting, L.M., Rao, J.A., & Langenecker, S.A. (2015). A new semantic list learning task to probe functioning of the Papez circuit. Journal of Clinical and Experimental Neuropsychology, 37(8), 816–833. CrossRef Google Scholar PubMed
- Schmidt, M. (1996). Rey auditory verbal learning test: RAVLT: A handbook. Los Angeles, CA: Western Psychological Services. Google Scholar
- Sheline, Y.I., Price, J.L., Yan, Z., & Mintun, M.A. (2010). Resting-state functional MRI in depression unmasks increased connectivity between networks via the dorsal nexus. Proceedings of the National Academy of Sciences of the United States of America, 107(24), 11020–11025. CrossRef Google Scholar PubMed
- Shipley, W.C., Gruber, C.P., Martin, T.A., & Klein, A.M. (2009). Shipley-2 manual. Los Angeles, CA: Western Psychological Services. Google Scholar
- Snyder, H. (2013). Major Depressive Disorder is associated with broad impairments on neuropsychological measures of executive function: A meta-analysis and review. Psychological Bulletin, 139(1), 81–132. CrossRef Google Scholar PubMed
- Stroop, J.R. (1938). Factors affecting speed in serial verbal reactions. Psychological Monographs, 50, 38. CrossRef Google Scholar
- Tahmasian, M., Knight, D.C., Manoliu, A., Schwerthöffer, D., Scherr, M., Chun, M., & Sorg, C. (2013). Aberrant intrinsic connectivity of hippocampus and amygdala overlap in the fronto-insular and dorsomedial-prefrontal cortex in major depressive disorder. Frontiers in Human Neuroscience, 7, 639. CrossRef Google Scholar
- Wechsler, D. (2008). Wechsler adult intelligence scale (4th ed.), (WAIS–IV). San Antonio, TX: NCS Pearson. Google Scholar
- Weiland-Fiedler, P., Erickson, K., Waldeck, T., Luckenbaugh, D.A., Pike, D., Bonne, O., & Neumeister, A. (2004). Evidence for continuing neuropsychological impairments in depression. Journal of Affective Disorders, 82(2), 253–258. CrossRef Google Scholar PubMed
- Weisenbach, S., Kassel, M., Rao, J., Weldon, A., Avery, E., Briceño, E., & Langenecker, S. (2014). Differential prefrontal and subcortical circuitry engagement during encoding of semantically related words in patients with late life depression. International Journal of Geriatric Psychiatry, 29(11), 1104–1115. CrossRef Google Scholar PubMed
- Zhang, J., Wang, J., Wu, Q., Kuang, W., Huang, X., He, Y., &&Gong, Q. (2011). Disrupted brain connectivity networks in drug-naïve first-episode Major Depressive Disorder. Biological Psychiatry, 70, 334–342. CrossRef Google Scholar PubMed
One of the most prominent features of schizophrenia is relatively lower general cognitive ability (GCA). An emerging approach to understanding the roots of variation in GCA relies on network properties of the brain. In this multi-center study, we determined global characteristics of brain networks using graph theory and related these to GCA in healthy controls and individuals with schizophrenia.
Participants (N=116 controls, 80 patients with schizophrenia) were recruited from four sites. GCA was represented by the first principal component of a large battery of neurocognitive tests. Graph metrics were derived from diffusion-weighted imaging.
The global metrics of longer characteristic path length and reduced overall connectivity predicted lower GCA across groups, and group differences were noted for both variables. Measures of clustering, efficiency, and modularity did not differ across groups or predict GCA. Follow-up analyses investigated three topological types of connectivity—connections among high degree “rich club” nodes, “feeder” connections to these rich club nodes, and “local” connections not involving the rich club. Rich club and local connectivity predicted performance across groups. In a subsample (N=101 controls, 56 patients), a genetic measure reflecting mutation load, based on rare copy number deletions, was associated with longer characteristic path length.
Results highlight the importance of characteristic path lengths and rich club connectivity for GCA and provide no evidence for group differences in the relationships between graph metrics and GCA. (JINS, 2016,22, 240–249)
- Andreasen, N.C. (1984a). Scale for the assessment of negative symptoms. Iowa City, IA: University of Iowa. Google Scholar PubMed
- Andreasen, N.C. (1984b). The scale for the assessment of positive symptoms. Iowa City, IA: The University of Iowa. Google Scholar
- Andreasen, N.C., Flaum, M., & Arndt, S. (1992). The Comprehensive Assessment of Symptoms and History (CASH) - An instrument for assessing diagnosis and psychopathology. Archives of General Psychiatry, 49(8), 615–623. CrossRef Google Scholar
- Bassett, D.S., Meyer-Lindenberg, A., Achard, S., Duke, T., & Bullmore, E. (2006). Adaptive reconfiguration of fractal small-world human functional networks. Proceedings of the National Academy of Sciences, 103(51), 19518–19523. CrossRef Google Scholar PubMed
- Bathelt, J., O’Reilly, H., Clayden, J.D., Cross, J.H., & de Haan, M. (2013). Functional brain network organisation of children between 2 and 5 years derived from reconstructed activity of cortical sources of high-density EEG recordings. Neuroimage, 82, 595–604. CrossRef Google Scholar PubMed
- Blair, C. (2006). How similar are fluid cognition and general intelligence? A developmental neuroscience perspective on fluid cognition as an aspect of human cognitive ability. The Behavioral and Brain Sciences, 29(2), 109–125; discussion 125–160. Google Scholar PubMed
- Bohlken, M.M., Mandl, R.C.W., Brouwer, R.M., van den Heuvel, M.P., Hedman, A.M., Kahn, R.S., & Hulshoff Pol, H.E. (2014). Heritability of structural brain network topology: A DTI study of 156 twins. Human Brain Mapping, 35(10), 5295–5305. CrossRef Google Scholar PubMed
- Bullmore, E., & Sporns, O. (2009). Complex brain networks: Graph theoretical analysis of structural and functional systems. Nature Reviews Neuroscience, 10(3), 186–198. CrossRef Google Scholar PubMed
- Caspi, A., Houts, R.M., Belsky, D.W., Goldman-Mellor, S.J., Harrington, H., Israel, S., & Moffitt, T.E. (2013). The p Factor: One general psychopathology factor in the structure of psychiatric disorders? Clinical Psychological Science, 2(2), 119–137. CrossRef Google Scholar
- Chang, L.C., Jones, D.K., & Pierpaoli, C. (2005). RESTORE: Robust estimation of tensors by outlier rejection. Magnetic Resonance in Medicine, 53, 1088–1095. CrossRef Google Scholar PubMed
- Chen, J., Liu, J., & Calhoun, V.D. (2010). Correction of copy number data using principal component analysis. 2010 IEEE International Conference on Bioinformatics and Biomedicine Workshops, 827–828. Google Scholar
- Chen, J., Liu, J., & Calhoun, V.D. (2011). A pipeline for copy number variation detection based on principal components analysis. Proceedings IEEE Bioinformatics and Biomedicine, 2011, 6975–6978. Google Scholar
- Colizza, V., Flammini, A., Serrano, M.A., & Vespignani, A. (2006). Detecting rich-club ordering in complex networks. Nature Physics, 2(2), 110–115. CrossRef Google Scholar
- De Reus, M.A., Saenger, V.M., Kahn, R.S., & van den Heuvel, M.P. (2014). An edge-centric perspective on the human connectome: Link communities in the brain. Philosophical Transactions of the Royal Society B: Biological Sciences, 369(1653), 20130527. CrossRef Google Scholar
- De Reus, M., & van den Heuvel, M.P. (2014). Simulated rich club lesioning in brain networks: A scaffold for communication and integration? Frontiers in Human Neuroscience, 8, 647. CrossRef Google Scholar
- Deary, I.J. (2012). Intelligence. Annual Review of Psychology, 63, 453–482. CrossRef Google Scholar PubMed
- Dennis, E.L., Jahanshad, N., Toga, A.W., McMahon, K.L., de Zubicaray, G.I., Martin, N.G., & Thompson, P.M. (2013). Test-retest reliability of graph theory measures of structural brain connectivity. Medical Imaging and Computer Assisted Intervention, 15, 305–312. Google Scholar
- Dickinson, D., & Harvey, P.D. (2009). Systemic hypotheses for generalized cognitive deficits in schizophrenia: A new take on an old problem. Schizophrenia Bulletin, 35(2), 403–414. CrossRef Google Scholar PubMed
- Fair, D.A., Cohen, A.L., Power, J.D., Dosenbach, N.U.F., Church, J.A., Miezin, F.M., & Petersen, S.E. (2009). Functional brain networks develop from a “local to distributed” organization. PLoS Computational Biology, 5(5), e1000381. CrossRef Google Scholar
- First, M., Spitzer, R.L., Gibbon, M., & Williams, J.B. (1997). Structured clinical interview for DSM-IV-TR axis I disorders. Washington, DC: American Psychiatric Press, Inc. Google Scholar
- Fischer, F.U., Wolf, D., Scheurich, A., & Fellgiebel, A. (2014). Association of structural global brain network properties with intelligence in normal aging. PloS One, 9(1), e86258. CrossRef Google Scholar
- Fischl, B., van der Kouwe, A., Destrieux, C., Halgren, E., Segoone, F., Salat, D.H., & Dale, A.M. (2004). Automatically parcellating the human cerebral cortex. Cerebral Cortex, 14(1), 11–22. CrossRef Google Scholar PubMed
- Gollub, R.L., Shoemaker, J.M., King, M.D., White, T., Ehrlich, S., Sponheim, S.R., & Andreasen, N.C. (2013). The MCIC collection: A shared repository of multi-modal, multi-site brain image data from a clinical investigation of schizophrenia. Neuroinformatics, 11(3), 367–388. CrossRef Google Scholar
- Hagmann, P., Cammoun, L., Gigandet, X., Meuli, R., Honey, C.J., Wedeen, V.J., & Sporns, O. (2008). Mapping the structural core of human cerebral cortex. PLoS Biol, 6(7), e159. CrossRef Google Scholar PubMed
- Haier, R.J., Colom, R., Schroeder, D.H., Condon, C.A., Tang, C., Eaves, E., & Head, K. (2009). Gray matter and intelligence factors: Is there a neuro-g? Intelligence, 37(2), 136. CrossRef Google Scholar
- Iturria-Medina, Y., Sotero, R.C., Canales-Rodriguez, E.J., Alemán-Gómez, Y., & Melie-Garcia, L. (2008). Studying the human brain anatomical network via diffusion-weighted MRI and graph theory. Neuroimage, 40(3), 1064–1076. CrossRef Google Scholar PubMed
- Johnson, W., Bouchard, T.J., Krueger, R.F., McGue, M., & Gottesman, I.I. (2004). Just one g: Conistent results from three test batteries. Intelligence, 32(1), 85–107. CrossRef Google Scholar
- Kahn, R.S., & Keefe, R.S.E. (2013). Schizophrenia is a cognitive illness: Time for a change. JAMA Psychiatry, 70(10), 1107–1112. CrossRef Google Scholar PubMed
- Latora, V., & Marchiori, M. (2001). Efficient behavior of small-world networks. Physical Review Letters, 87(19), 198701. CrossRef Google Scholar PubMed
- Lencz, T., Knowles, E., Davies, G., Guha, S., Liewald, D.C., Starr, J.M., & Malhotra, A.K. (2014). Molecular genetic evidence for overlap between general cognitive ability and risk for schizophrenia: A report from the Cognitive Genomics consorTium (COGENT). Molecular Psychiatry, 19(2), 168–174. CrossRef Google Scholar
- Li, Y., Liu, Y., Li, J., Qin, W., Li, K., Yu, C., & Jiang, T. (2009). Brain anatomical network and intelligence. PLoS Computational Biology, 5(5), e1000395. CrossRef Google Scholar PubMed
- Martin, A.K., Robinson, G., Reutens, D., & Mowry, B. (2014). Cognitive and structural neuroimaging characteristics of schizophrenia patients with large, rare copy number deletions. Psychiatry Research: Neuroimaging, 224, 311–318. CrossRef Google Scholar
- McAuley, J.J., da Fontoura Costa, L., & Caetano, T.S. (2007). Rich-club phenomenon across complex network hierarchies. Applied Physics Letters, 91(8), 084103. CrossRef Google Scholar
- Messé, A., Marrelec, G., Bellec, P., Perlbarg, V., Doyon, J., Pélégrini-Issac, M., & Benali, H. (2012). Comparing structural and functional graph theory features in the human brain using multimodal MRI. Irbm, 33(4), 244–253. CrossRef Google Scholar
- Mori, S., & van Zijl, P.C. (2002). Fiber tracking: Principles and strategies - A technical review. NMR Biomedicine, 15, 468–480. CrossRef Google Scholar
- Nisbett, R.E., Aronson, J., Blair, C., Dickens, W., Flynn, J., Halpern, D.F., & Turkheimer, E. (2012). Intelligence: New findings and theoretical developments. American Psychologist, 67(2), 130–159. CrossRef Google Scholar PubMed
- Raznahan, A., Greenstein, D., Lee, N.R., Clasen, L.S., & Giedd, J.N. (2012). Prenatal growth in humans and postnatal brain maturation into late adolescence. Proceedings of the National Academy of Sciences, 109, 11366–11371. CrossRef Google Scholar PubMed
- Rubinov, M., & Sporns, O. (2010). Complex network measures of brain connectivity: Uses and interpretations. Neuroimage, 52(3), 1059–1069. doi:10.1016/j.neuroimage.2009.10.003 CrossRef Google Scholar PubMed
- Ryman, S.G., Vakhtin, A.A., Yeo, R.A., van den Heuvel, M.P., de Reus, M., Flores, R.A., Caprihan, A., & Jung, R.E. (n.d.). The cost of intelligence: Graph analysis of white matter connectivity in a large normal cohort. Under review. Google Scholar
- Ryman, S.G., van den Heuvel, M.P., Yeo, R.A., Caprihan, A., Carrasco, J., Vakhtin, A.A., Jung, R.E. (2014). Sex differences in the relationship between white matter connectivity and creativity. Neuroimage, 101, 380–389. CrossRef Google Scholar PubMed
- Schaefer, J., Giangrande, E., Weinberger, D.R., & Dickinson, D. (2013). The global cognitive impairment in schizophrenia: Consistent over decades and around the world. Schizophrenia Research, 150(1), 42–50. CrossRef Google Scholar
- Selig, J.P., & Preacher, K. (2008). Monte Carlo method for assessing mediation: An interactive tool for creating confidence intervals for indirect effects [Computer software]. Retrieved from http://quantpsy.org/. Google Scholar
- Senden, M., Deco, G., de Reus, M.A., Goebel, R., & van den Heuvel, M.P. (2014). Rich club organization supports a diverse set of functional network configurations. Neuroimage, 96, 174–182. CrossRef Google Scholar PubMed
- Sponheim, S.R., Jung, R.E., Seidman, L.J., Mesholam-Gately, R.I., Manoach, D.S., O’Leary, D.S., & Schulz, S.C. (2010). Cognitive deficits in recent-onset and chronic schizophrenia. Journal of Psychiatric Research, 44(7), 421–428. CrossRef Google Scholar PubMed
- Van den Heuvel, M.P., & Fornito, A. (2014). Brain networks in schizophrenia. Neuropsychology Review, 24(1), 32–48. CrossRef Google Scholar
- Van den Heuvel, M.P., Kahn, R.S., Goñi, J., & Sporns, O. (2012). High-cost, high capacity backbone for global brain communication. Proceedings of the National Academy of Sciences of the United States of America, 109, 11372–11377. CrossRef Google Scholar PubMed
- Van den Heuvel, M.P., & Sporns, O. (2011). Rich-club organization of the human connectome. The Journal of Neuroscience, 31(44), 15775–15786. doi:10.1523/JNEUROSCI.3539-11.2011 CrossRef Google Scholar PubMed
- Van den Heuvel, M.P., Sporns, O., Collin, G., Scheewe, T., Mandl, R.C.W., Cahn, W., & Kahn, R.S. (2013). Abnormal rich club organization and functional brain dynamics in schizophrenia. JAMA Psychiatry, 70(8), 783–792. doi:10.1001/jamapsychiatry.2013.1328 CrossRef Google Scholar
- Van den Heuvel, M.P., Stam, C.J., Kahn, R.S., & Hulshoff Pol, H.E. (2009). Efficiency of functional brain networks and intellectual performance. Journal of Neuroscience, 29(23), 7619–7624. CrossRef Google Scholar
- Von Ehrenstein, O.S., Mikolajczyk, R.T., & Zhang, J. (2009). Timing and trajectories of fetal growth related to cognitive development in childhood. American Journal of Epidemiology, 170(11), 1388–1395. CrossRef Google Scholar PubMed
- Watts, D.J., & Strogatz, S.H. (1998). Collective dynamics of “small-world” networks. Nature, 393, 440–442. CrossRef Google Scholar PubMed
- Yeo, R.A., Gangestad, S.W., Walton, E., Ehrlich, S., Pommy, J., Turner, J.A., & Calhoun, V.D. (2014). Genetic influences on cognitive endophenotypes in schizophrenia. Schizophrenia Research, 156(1), 71–75. CrossRef Google Scholar
- Yeo, R.A., & Gangestad, S.W. (2015). Developmental instability, mutation load, and neurodevelopmental disorders. In K.J. Mitchell (Ed.), Genetics of neurodevelopmental disorders (pp. 81–110). Hoboken, NJ: Wiley-Blackwell. CrossRef Google Scholar
- Yeo, R.A., Gangestad, S.W., Liu, J., Ehrlich, S., Thoma, R.J., Pommy, J.M., & Calhoun, V.D. (2013). The impact of copy number deletions on general cognitive ability and ventricle size in patients with schizophrenia and healthy control subjects. Biological Psychiatry, 73(6), 540–545. CrossRef Google Scholar
- Yeo, R.A., Martinez, D., Pommy, J., Ehrlich, S., Schulz, S.C., Ho, B.-C., & Calhoun, V.D. (2013). The impact of parent socio-economic status on executive functioning and cortical morphology in individuals with schizophrenia and healthy controls. Psychological Medicine, 44, 1257–1265. CrossRef Google Scholar
- Yu, Q., Plis, S.M., Erhardt, E.B., Allen, E.A., Sui, J., Kiehl, K.A., & Calhoun, V.D. (2011). Modular organization of functional network connectivity in healthy controls and patients with schizophrenia during the resting state. Frontiers in Systems Neuroscience, 5, 103. Google Scholar PubMed
- Yu, Q., Plis, S.M., Erhardt, E.B., Allen, E.A., Sui, J., Kiehl, K.A., & Calhoun, V.D. (2013). Disrupted correlation between low frequency power and connectivity strength of resting state brain networks in schizophrenia. Schizophrenia Research, 143(1), 165–171. CrossRef Google Scholar
- Zalesky, A., Fornito, A., Seal, M.L., Cocchi, L., Westin, C.-F., Bullmore, E.T., & Pantelis, C. (2011). Disrupted axonal fiber connectivity in schizophrenia. Biological Psychiatry, 69(1), 80–89. CrossRef Google Scholar
- Zhang, F., Gu, W., Hurles, M.E., & Lupski, J.R. (2009). Copy number variation in human health, disease, and evolution. Annual Review of Genomics and Human Genetics, 10, 451–481. CrossRef Google Scholar PubMed
Behavioral variant frontotemporal dementia (bvFTD) is characterized by early atrophy in the frontotemporoinsular regions. These regions overlap with networks that are engaged in social cognition-executive functions, two hallmarks deficits of bvFTD. We examine (i) whether Network Centrality (a graph theory metric that measures how important a node is in a brain network) in the frontotemporoinsular network is disrupted in bvFTD, and (ii) the level of involvement of this network in social-executive performance.
Patients with probable bvFTD, healthy controls, and frontoinsular stroke patients underwent functional MRI resting-state recordings and completed social-executive behavioral measures.
Relative to the controls and the stroke group, the bvFTD patients presented decreased Network Centrality. In addition, this measure was associated with social cognition and executive functions. To test the specificity of these results for the Network Centrality of the frontotemporoinsular network, we assessed the main areas from six resting-state networks. No group differences or behavioral associations were found in these networks. Finally, Network Centrality and behavior distinguished bvFTD patients from the other groups with a high classification rate.
bvFTD selectively affects Network Centrality in the frontotemporoinsular network, which is associated with high-level social and executive profile. (JINS, 2016,22, 250–262)
- Agosta, F., Sala, S., Valsasina, P., Meani, A., Canu, E., Magnani, G., & Filippi, M. (2013). Brain network connectivity assessed using graph theory in frontotemporal dementia. Neurology, 81(2), 134–143. doi:10.1212/WNL.0b013e31829a33f8 CrossRef Google Scholar PubMed
- Altman, N.S. (1992). An introduction to kernel and nearest-neighbor nonparametric regression. The American Statistician, 46(3), 175–185. doi:10.1080/00031305.1992.10475879 Google Scholar
- Baez, S., Couto, B., Torralva, T., Sposato, L.A., Huepe, D., Montanes, P., & Ibanez, A. (2014). Comparing moral judgments of patients with frontotemporal dementia and frontal stroke. JAMA Neurology, 71(9), 1172–1176. doi:10.1001/jamaneurol.2014.347 CrossRef Google Scholar PubMed
- Baggio, H.C., Sala-Llonch, R., Segura, B., Marti, M.J., Valldeoriola, F., Compta, Y., & Junque, C. (2014). Functional brain networks and cognitive deficits in Parkinson’s disease. Human Brain Mapping, 35(9), 4620–4634. doi:10.1002/hbm.22499 CrossRef Google Scholar PubMed
- Baron-Cohen, S., Jolliffe, T., Mortimore, C., & Robertson, M. (1997). Another advanced test of theory of mind: Evidence from very high functioning adults with autism or asperger syndrome. Journal of Child Psychology and Psychiatry, and Allied Disciplines, 38(7), 813–822. CrossRef Google Scholar PubMed
- Barttfeld, P., Wicker, B., Cukier, S., Navarta, S., Lew, S., Leiguarda, R., & Sigman, M. (2012). State-dependent changes of connectivity patterns and functional brain network topology in autism spectrum disorder. Neuropsychologia, 50(14), 3653–3662. doi:10.1016/j.neuropsychologia.2012.09.047 CrossRef Google Scholar PubMed
- Barttfeld, P., Wicker, B., McAleer, P., Belin, P., Cojan, Y., Graziano, M., & Sigman, M. (2013). Distinct patterns of functional brain connectivity correlate with objective performance and subjective beliefs. Proceedings of the National Academy of Sciences of the United Sates of America, 110(28), 11577–11582. doi:10.1073/pnas.1301353110 CrossRef Google Scholar PubMed
- Beckmann, C.F., DeLuca, M., Devlin, J.T., & Smith, S.M. (2005). Investigations into resting-state connectivity using independent component analysis. Philosophical Transactions of the Royal Society of London, Series B, Biological Sciences, 360(1457), 1001–1013. doi:10.1098/rstb.2005.1634 CrossRef Google Scholar PubMed
- Brandes, U. (2001). A faster algorithm for betweenness centrality. Journal of Mathematical Sociology, 25(2), 163–177. CrossRef Google Scholar
- Brier, M.R., Thomas, J.B., Fagan, A.M., Hassenstab, J., Holtzman, D.M., Benzinger, T.L., & Ances, B.M. (2014). Functional connectivity and graph theory in preclinical Alzheimer’s disease. Neurobiol Aging, 35(4), 757–768. doi:10.1016/j.neurobiolaging.2013.10.081 CrossRef Google Scholar PubMed
- Buckner, R.L., Sepulcre, J., Talukdar, T., Krienen, F.M., Liu, H., Hedden, T., & Johnson, K.A. (2009). Cortical hubs revealed by intrinsic functional connectivity: Mapping, assessment of stability, and relation to Alzheimer’s disease. The Journal of Neuroscience, 29(6), 1860–1873. doi:10.1523/JNEUROSCI.5062-08.2009 CrossRef Google Scholar PubMed
- Butman, J., Allegri, R.F., Harris, P., & Drake, M. (2000). Spanish verbal fluency. Normative data in Argentina. Medicina (B Aires), 60(5 Pt 1), 561–564. Google Scholar PubMed
- Couto, B., Manes, F., Montanes, P., Matallana, D., Reyes, P., Velasquez, M., & Ibanez, A. (2013). Structural neuroimaging of social cognition in progressive non-fluent aphasia and behavioral variant of frontotemporal dementia. Frontiers in Human Neuroscience, 7, 467, doi:10.3389/fnhum.2013.00467 CrossRef Google Scholar PubMed
- Chow, T.W., Hynan, L.S., & Lipton, A.M. (2006). MMSE scores decline at a greater rate in frontotemporal degeneration than in AD. Dementia and Geriatric Cognitive Disorders, 22(3), 194–199. doi:10.1159/000094870 CrossRef Google Scholar
- Damoiseaux, J.S., Rombouts, S.A., Barkhof, F., Scheltens, P., Stam, C.J., Smith, S.M., & Beckmann, C.F. (2006). Consistent resting-state networks across healthy subjects. Proceedings of the National Academy of Sciences of the United Sates of America, 103(37), 13848–13853. doi:10.1073/pnas.0601417103 CrossRef Google Scholar PubMed
- Day, G.S., Farb, N.A., Tang-Wai, D.F., Masellis, M., Black, S.E., Freedman, M., & Chow, T.W. (2013). Salience network resting-state activity: Prediction of frontotemporal dementia progression. JAMA Neurology, 70(10), 1249–1253. doi:10.1001/jamaneurol.2013.3258 Google Scholar PubMed
- de Haan, W., Pijnenburg, Y.A., Strijers, R.L., van der Made, Y., van der Flier, W.M., Scheltens, P., & Stam, C.J. (2009). Functional neural network analysis in frontotemporal dementia and Alzheimer’s disease using EEG and graph theory. BMC Neuroscience, 10, 101, doi:10.1186/1471-2202-10-101 CrossRef Google Scholar PubMed
- Decety, J. (2011). The neuroevolution of empathy. Annals of the New York Academy of Sciences, 1231, 35–45. doi:10.1111/j.1749-6632.2011.06027.x CrossRef Google Scholar PubMed
- Eslinger, P.J., Moore, P., Anderson, C., & Grossman, M. (2011). Social cognition, executive functioning, and neuroimaging correlates of empathic deficits in frontotemporal dementia. The Journal of Neuropsychiatry and Clinical Neurosciences, 23(1), 74–82. doi:10.1176/appi.neuropsych.23.1.74 CrossRef Google Scholar PubMed
- Farb, N.A., Grady, C.L., Strother, S., Tang-Wai, D.F., Masellis, M., Black, S., & Chow, T.W. (2013). Abnormal network connectivity in frontotemporal dementia: Evidence for prefrontal isolation. Cortex, 49(7), 1856–1873. doi:10.1016/j.cortex.2012.09.008 CrossRef Google Scholar PubMed
- Filippi, M., Agosta, F., Scola, E., Canu, E., Magnani, G., Marcone, A., & Falini, A. (2013). Functional network connectivity in the behavioral variant of frontotemporal dementia. Cortex, 49(9), 2389–2401. doi:10.1016/j.cortex.2012.09.017 CrossRef Google Scholar PubMed
- Freeman, L.C. (1977). A set of measures of centrality based on betweenness. Sociometry, 40(1), 35–41. CrossRef Google Scholar
- Garcia-Cordero, I., Sedeño, L., Fraiman, D., Craiem, D., de la Fuente, L.A., Salamone, P., & Ibanez, A. (2015). Stroke and neurodegeneration induce different connectivity aberrations in the insula. Stroke, doi:10.1161/STROKEAHA.115.009598 CrossRef Google Scholar PubMed
- Gleichgerrcht, E., Roca, M., Manes, F., & Torralva, T. (2011). Comparing the clinical usefulness of the Institute of Cognitive Neurology (INECO) Frontal Screening (IFS) and the Frontal Assessment Battery (FAB) in frontotemporal dementia. Journal of Clinical and Experimental Neuropsychology, 33(9), 997–1004. doi:10.1080/13803395.2011.589375 CrossRef Google Scholar
- Goch, C.J., Stieltjes, B., Henze, R., Hering, J., Poustka, L., Meinzer, H.P., & Maier-Hein, K.H. (2014). Quantification of changes in language-related brain areas in autism spectrum disorders using large-scale network analysis. International Journal of Computer Assisted Radiology and Surgery, 9(3), 357–365. doi:10.1007/s11548-014-0977-0 CrossRef Google Scholar PubMed
- Grefkes, C., & Fink, G.R. (2014). Connectivity-based approaches in stroke and recovery of function. Lancet Neurology, 13(2), 206–216. doi:10.1016/S1474-4422(13)70264-3 CrossRef Google Scholar PubMed
- He, Y., Chen, Z., & Evans, A. (2008). Structural insights into aberrant topological patterns of large-scale cortical networks in Alzheimer’s disease. The Journal of Neuroscience, 28(18), 4756–4766. doi:10.1523/JNEUROSCI.0141-08.2008 CrossRef Google Scholar PubMed
- Ibanez, A., & Manes, F. (2012). Contextual social cognition and the behavioral variant of frontotemporal dementia. Neurology, 78(17), 1354–1362. CrossRef Google Scholar PubMed
- Kalcher, K., Huf, W., Boubela, R.N., Filzmoser, P., Pezawas, L., Biswal, B., & Windischberger, C. (2012). Fully exploratory network independent component analysis of the 1000 functional connectomes database. Frontiers in Human Neuroscience, 6, 301. doi:10.3389/fnhum.2012.00301 CrossRef Google Scholar PubMed
- Kennedy, D.P., & Adolphs, R. (2012). The social brain in psychiatric and neurological disorders. Trends in Cognitive Sciences, 16(11), 559–572. doi:10.1016/j.tics.2012.09.006 CrossRef Google Scholar PubMed
- Kipps, C.M., Nestor, P.J., Acosta-Cabronero, J., Arnold, R., & Hodges, J.R. (2009). Understanding social dysfunction in the behavioural variant of frontotemporal dementia: The role of emotion and sarcasm processing. Brain, 132(3), 592–603. doi:10.1093/brain/awn314 CrossRef Google Scholar PubMed
- Kril, J.J., Macdonald, V., Patel, S., Png, F., & Halliday, G.M. (2005). Distribution of brain atrophy in behavioral variant frontotemporal dementia. Journal of the Neurological Sciences, 232(1-2), 83–90. doi:10.1016/j.jns.2005.02.003 CrossRef Google Scholar PubMed
- Kumfor, F., Irish, M., Leyton, C., Miller, L., Lah, S., Devenney, E., & Piguet, O. (2014). Tracking the progression of social cognition in neurodegenerative disorders. Journal of Neurology, Neurosurgery, and Psychiatry, 85(10), 1076–1083. doi:10.1136/jnnp-2013-307098 CrossRef Google Scholar PubMed
- Lambon Ralph, M.A., Cipolotti, L., Manes, F., & Patterson, K. (2010). Taking both sides: Do unilateral anterior temporal lobe lesions disrupt semantic memory? Brain, 133(11), 3243–3255. CrossRef Google Scholar PubMed
- Li, Y., Qin, Y., Chen, X., & Li, W. (2013). Exploring the functional brain network of Alzheimer’s disease: Based on the computational experiment. PLoS One, 8(9), e73186. doi:10.1371/journal.pone.0073186 CrossRef Google Scholar PubMed
- Liu, Z., Zhang, Y., Yan, H., Bai, L., Dai, R., Wei, W., & Tian, J. (2012). Altered topological patterns of brain networks in mild cognitive impairment and Alzheimer’s disease: A resting-state fMRI study. Psychiatry Research, 202(2), 118–125. doi:10.1016/j.pscychresns.2012.03.002 CrossRef Google Scholar PubMed
- MacQueen, J.B. (1967). Some methods for classification and analysis of multivariate observations. Proceedings of the Fifth Berkeley Symposium on Mathematical Statistics and Probability, 1, 281–297. Google Scholar
- Maris, E., & Oostenveld, R. (2007). Nonparametric statistical testing of EEG- and MEG-data. Journal of Neuroscience Methods, 164(1), 177–190. doi:10.1016/j.jneumeth.2007.03.024 CrossRef Google Scholar PubMed
- McDonald, S., Flanagan, S., Rollins, J., & Kinch, J. (2003). TASIT: A new clinical tool for assessing social perception after traumatic brain injury. The Journal of Head Trauma Rehabilitation, 18(3), 219–238. CrossRef Google Scholar PubMed
- Mesulam, M.M. (1986). Frontal cortex and behavior. Annals of Neurology, 19(4), 320–325. doi:10.1002/ana.410190403 CrossRef Google Scholar PubMed
- Nestor, P.J. (2013). Degenerator tau/TDP-43: Rise of the machines. Journal of Neurology, Neurosurgery, and Psychiatry, 84(9), 945. doi:10.1136/jnnp-2012-304681 CrossRef Google Scholar PubMed
- Nichols, T.E., & Holmes, A.P. (2002). Nonparametric permutation tests for functional neuroimaging: A primer with examples. Human Brain Mapping, 15(1), 1–25. CrossRef Google Scholar PubMed
- Oostenveld, R., Fries, P., Maris, E., & Schoffelen, J.M. (2011). FieldTrip: Open source software for advanced analysis of MEG, EEG, and invasive electrophysiological data. Computational Intelligence and Neuroscience, 2011, 156869. doi:10.1155/2011/156869 CrossRef Google Scholar PubMed
- Opsahl, T., Agneessens, F., & Skvoretz, J. (2010). Node centrality in weighted networks: Generalizing degree and shortest path. Social Networks, 32(3), 245–251. CrossRef Google Scholar
- Pievani, M., de Haan, W., Wu, T., Seeley, W.W., & Frisoni, G.B. (2011). Functional network disruption in the degenerative dementias. Lancet Neurology, 10(9), 829–843. doi:10.1016/S1474-4422(11)70158-2 CrossRef Google Scholar PubMed
- Piguet, O., Hornberger, M., Mioshi, E., & Hodges, J.R. (2011). Behavioural-variant frontotemporal dementia: Diagnosis, clinical staging, and management. Lancet Neurology, 10(2), 162–172. doi:10.1016/S1474-4422(10)70299-4 CrossRef Google Scholar PubMed
- Possin, K.L., Feigenbaum, D., Rankin, K.P., Smith, G.E., Boxer, A.L., Wood, K., & Kramer, J.H. (2013). Dissociable executive functions in behavioral variant frontotemporal and Alzheimer dementias. Neurology, 80(24), 2180–2185. doi:10.1212/WNL.0b013e318296e940 CrossRef Google Scholar PubMed
- Rankin, K.P., Kramer, J.H., & Miller, B.L. (2005). Patterns of cognitive and emotional empathy in frontotemporal lobar degeneration. Cognitive and Behavioral Neurology, 18(1), 28–36. CrossRef Google Scholar PubMed
- Rascovsky, K., Hodges, J.R., Knopman, D., Mendez, M.F., Kramer, J.H., Neuhaus, J., & Miller, B.L. (2011). Sensitivity of revised diagnostic criteria for the behavioural variant of frontotemporal dementia. Brain, 134(9), 2456–2477. doi:10.1093/brain/awr179 CrossRef Google Scholar PubMed
- Rascovsky, K., Salmon, D.P., Lipton, A.M., Leverenz, J.B., DeCarli, C., Jagust, W.J., & Galasko, D. (2005). Rate of progression differs in frontotemporal dementia and Alzheimer disease. Neurology, 65(3), 397–403. doi:10.1212/01.wnl.0000171343.43314.6e CrossRef Google Scholar PubMed
- Rosen, H.J., Gorno-Tempini, M.L., Goldman, W.P., Perry, R.J., Schuff, N., Weiner, M., & Miller, B.L. (2002). Patterns of brain atrophy in frontotemporal dementia and semantic dementia. Neurology, 58(2), 198–208. CrossRef Google Scholar PubMed
- Rytty, R., Nikkinen, J., Paavola, L., Abou Elseoud, A., Moilanen, V., Visuri, A., & Remes, A.M. (2013). GroupICA dual regression analysis of resting state networks in a behavioral variant of frontotemporal dementia. Frontiers in Human Neuroscience, 7, 461. doi:10.3389/fnhum.2013.00461 CrossRef Google Scholar
- Sajjadi, S.A., Acosta-Cabronero, J., Patterson, K., Diaz-de-Grenu, L.Z., Williams, G.B., & Nestor, P.J. (2013). Diffusion tensor magnetic resonance imaging for single subject diagnosis in neurodegenerative diseases. Brain, 136(7), 2253–2261. doi:10.1093/brain/awt118 CrossRef Google Scholar PubMed
- Sanz-Arigita, E.J., Schoonheim, M.M., Damoiseaux, J.S., Rombouts, S.A., Maris, E., Barkhof, F., & Stam, C.J. (2010). Loss of ‘small-world’ networks in Alzheimer’s disease: Graph analysis of FMRI resting-state functional connectivity. PLoS One, 5(11), e13788. doi:10.1371/journal.pone.0013788 CrossRef Google Scholar PubMed
- Sedeño, L., Couto, B., Melloni, M., Canales-Johnson, A., Yoris, A., Baez, S., & Ibanez, A. (2014). How do you feel when you can’t feel your body? Interoception, functional connectivity and emotional processing in depersonalization-derealization disorder. PLoS One, 9(6), e98769. doi:10.1371/journal.pone.0098769 CrossRef Google Scholar PubMed
- Seeley, W.W., Crawford, R., Rascovsky, K., Kramer, J.H., Weiner, M., Miller, B.L., & Gorno-Tempini, M.L. (2008). Frontal paralimbic network atrophy in very mild behavioral variant frontotemporal dementia. Archives of Neurology, 65(2), 249–255. doi:10.1001/archneurol.2007.38 CrossRef Google Scholar PubMed
- Seo, E.H., Lee, D.Y., Lee, J.M., Park, J.S., Sohn, B.K., Lee, D.S., & Woo, J.I. (2013). Whole-brain functional networks in cognitively normal, mild cognitive impairment, and Alzheimer’s disease. PLoS One, 8(1), e53922. doi:10.1371/journal.pone.0053922 CrossRef Google Scholar PubMed
- Singer, T. (2006). The neuronal basis and ontogeny of empathy and mind reading: Review of literature and implications for future research. Neuroscience and Biobehavioral Reviews, 30(6), 855–863. doi:10.1016/j.neubiorev.2006.06.011 CrossRef Google Scholar PubMed
- Singer, T., & Lamm, C. (2009). The social neuroscience of empathy. Annals of the New York Academy of Sciences, 1156, 81–96. doi:10.1111/j.1749-6632.2009.04418.x CrossRef Google Scholar PubMed
- Smith, S.M., Fox, P.T., Miller, K.L., Glahn, D.C., Fox, P.M., Mackay, C.E., & Beckmann, C.F. (2009). Correspondence of the brain’s functional architecture during activation and rest. Proceedings of the National Academy of Sciences of the United Sates of America, 106(31), 13040–13045. doi:10.1073/pnas.0905267106 CrossRef Google Scholar
- Sporns, O. (2014). Contributions and challenges for network models in cognitive neuroscience. Nature Neuroscience, 17(5), 652–660. doi:10.1038/nn.3690 CrossRef Google Scholar PubMed
- Sporns, O., & Zwi, J.D. (2004). The small world of the cerebral cortex. Neuroinformatics, 2(2), 145–162. doi:10.1385/NI:2:2:145 CrossRef Google Scholar PubMed
- Stanley, D.A., & Adolphs, R. (2013). Toward a neural basis for social behavior. Neuron, 80(3), 816–826. doi:10.1016/j.neuron.2013.10.038 CrossRef Google Scholar
- Supekar, K., & Menon, V. (2012). Developmental maturation of dynamic causal control signals in higher-order cognition: A neurocognitive network model. PLoS Computational Biology, 8(2), e1002374. doi:10.1371/journal.pcbi.1002374 CrossRef Google Scholar PubMed
- Supekar, K., Menon, V., Rubin, D., Musen, M., & Greicius, M.D. (2008). Network analysis of intrinsic functional brain connectivity in Alzheimer’s disease. PLoS Computational Biology, 4(6), e1000100. doi:10.1371/journal.pcbi.1000100 CrossRef Google Scholar PubMed
- Tian, L., Wang, J., Yan, C., & He, Y. (2011). Hemisphere- and gender-related differences in small-world brain networks: A resting-state functional MRI study. Neuroimage, 54(1), 191–202. doi:10.1016/j.neuroimage.2010.07.066 CrossRef Google Scholar PubMed
- Torralva, T., Roca, M., Gleichgerrcht, E., Lopez, P., & Manes, F. (2009). INECO Frontal Screening (IFS): A brief, sensitive, and specific tool to assess executive functions in dementia. Journal of the International Neuropsychological Society, 15(5), 777–786. doi:10.1017/S1355617709990415 CrossRef Google Scholar PubMed
- Tzourio-Mazoyer, N., Landeau, B., Papathanassiou, D., Crivello, F., Etard, O., Delcroix, N., & Joliot, M. (2002). Automated anatomical labeling of activations in SPM using a macroscopic anatomical parcellation of the MNI MRI single-subject brain. Neuroimage, 15(1), 273–289. doi:10.1006/nimg.2001.0978 CrossRef Google Scholar PubMed
- van den Heuvel, M., Mandl, R., & Hulshoff Pol, H. (2008). Normalized cut group clustering of resting-state FMRI data. PLoS One, 3(4), e2001. doi:10.1371/journal.pone.0002001 CrossRef Google Scholar PubMed
- Van Dijk, K.R., Sabuncu, M.R., & Buckner, R.L. (2012). The influence of head motion on intrinsic functional connectivity MRI. Neuroimage, 59(1), 431–438. doi:10.1016/j.neuroimage.2011.07.044 CrossRef Google Scholar PubMed
- Whitwell, J.L., Josephs, K.A., Avula, R., Tosakulwong, N., Weigand, S.D., Senjem, M.L., & Jack, C.R. Jr (2011). Altered functional connectivity in asymptomatic MAPT subjects: A comparison to bvFTD. Neurology, 77(9), 866–874. doi:10.1212/WNL.0b013e31822c61f2 CrossRef Google Scholar PubMed
- Whitwell, J.L., Przybelski, S.A., Weigand, S.D., Ivnik, R.J., Vemuri, P., Gunter, J.L., & Josephs, K.A. (2009). Distinct anatomical subtypes of the behavioural variant of frontotemporal dementia: A cluster analysis study. Brain, 132(11), 2932–2946. doi:10.1093/brain/awp232 CrossRef Google Scholar PubMed
- Xiang, J., Guo, H., Cao, R., Liang, H., & Chen, J. (2013). An abnormal resting-state functional brain network indicates progression towards Alzheimer’s disease. Neural Regeneration Research, 8(30), 2789–2799. doi:10.3969/j.issn.1673-5374.2013.30.001 Google Scholar PubMed
- Yao, Z., Zhang, Y., Lin, L., Zhou, Y., Xu, C., Jiang, T., & Alzheimer’s Disease Neuroimaging, I. (2010). Abnormal cortical networks in mild cognitive impairment and Alzheimer’s disease. PLoS Computational Biology, 6(11), e1001006. doi:10.1371/journal.pcbi.1001006 CrossRef Google Scholar PubMed
- Zhou, J., Greicius, M.D., Gennatas, E.D., Growdon, M.E., Jang, J.Y., Rabinovici, G.D., & Seeley, W.W. (2010). Divergent network connectivity changes in behavioural variant frontotemporal dementia and Alzheimer’s disease. Brain, 133(5), 1352–1367. doi:10.1093/brain/awq075 CrossRef Google Scholar PubMed
- Zuo, X.N., Ehmke, R., Mennes, M., Imperati, D., Castellanos, F.X., Sporns, O., & Milham, M.P. (2012). Network centrality in the human functional connectome. Cerebral Cortex, 22(8), 1862–1875. doi:10.1093/cercor/bhr269 CrossRef Google Scholar PubMed
Individuals with chronic traumatic brain injury (TBI) often show detrimental deficits in higher order cognitive functions requiring coordination of multiple brain networks. Although assessing TBI-related deficits in higher order cognition in the context of network dysfunction is promising, few studies have systematically investigated altered interactions among multiple networks in chronic TBI.
We characterized disrupted resting-state functional connectivity of the default mode network (DMN), dorsal attention network (DAN), and frontoparietal control network (FPCN) whose interactions are required for internally and externally focused goal-directed cognition in chronic TBI. Specifically, we compared the network interactions of 40 chronic TBI individuals (8 years post-injury on average) with those of 17 healthy individuals matched for gender, age, and years of education.
The network-based statistic (NBS) on DMN-DAN-FPCN connectivity of these groups revealed statistically significant (pNBS<.05; |Z|>2.58) reductions in within-DMN, within-FPCN, DMN-DAN, and DMN-FPCN connectivity of the TBI group over healthy controls. Importantly, such disruptions occurred prominently in between-network connectivity. Subsequent analyses further exhibited the disrupted connectivity patterns of the chronic TBI group occurring preferentially in long-range and inter-hemispheric connectivity of DMN-DAN-FPCN. Most importantly, graph-theoretic analysis demonstrated relative reductions in global, local and cost efficiency (p<.05) as a consequence of the network disruption patterns in the TBI group.
Our findings suggest that assessing multiple networks-of-interest simultaneously will allow us to better understand deficits in goal-directed cognition and other higher order cognitive phenomena in chronic TBI. Future research will be needed to better understand the behavioral consequences related to these network disruptions. (JINS, 2016,22, 263–279)
- Achard, S., & Bullmore, E. (2007). Efficiency and cost of economical brain functional networks. PLoS Computational Biology, 3, e17. doi:10.1371/journal.pcbi.0030017 CrossRef Google Scholar PubMed
- Anderson, J.S., Druzgal, T.J., Lopez-Larson, M., Jeong, E.-K., Desai, K., & Yurgelun-Todd, D. (2011). Network anticorrelations, global regression, and phase-shifted soft tissue correction. Human Brain Mapping, 32, 919–934. CrossRef Google Scholar
- Arciniegas, D., Olincy, A., Topkoff, J., McRae, K., Cawthra, E., Filley, C.M., & Adler, L.E. (2000). Impaired auditory gating and P50 nonsuppression following traumatic brain injury. The Journal of Neuropsychiatry and Clinical Neurosciences, 12, 77–85. CrossRef Google Scholar PubMed
- Baird, B., Smallwood, J., & Schooler, J.W. (2011). Back to the future: Autobiographical planning and the functionality of mind-wandering. Consciousness and Cognition, 20, 1604–1611. CrossRef Google Scholar PubMed
- Beck, A.T., Steer, R.A. & Brown, G.K. (1996). BDI-II, Beck depression inventory: Manual (2nd ed). San Antonio, TX: Psychological Corporation, and Boston: Harcourt Brace. Google Scholar
- Bengtsson, S.L., Dolan, R.J., & Passingham, R.E. (2011). Priming for self-esteem influences the monitoring of one’s own performance. Social Cognitive and Affective Neuroscience, 6, 417–425. CrossRef Google Scholar PubMed
- Bonnelle, V., Ham, T.E., Leech, R., Kinnunen, K.M., Mehta, M.A., Greenwood, R.J., & Sharp, D.J. (2012). Salience network integrity predicts default mode network function after traumatic brain injury. Proceedings of the National Academy of Sciences of the United States of America, 109, 4690–4695. CrossRef Google Scholar
- Bonnelle, V., Leech, R., Kinnunen, K.M., Ham, T.E., Beckmann, C.F., De Boissezon, X., & Sharp, D.J. (2011). Default mode network connectivity predicts sustained attention deficits after traumatic brain injury. Journal of Neuroscience, 31, 13442–13451. CrossRef Google Scholar
- Caeyenberghs, K., Leemans, A., Heitger, M.H., Leunissen, I., Dhollander, T., Sunaert, S., & Swinnen, S.P. (2012). Graph analysis of functional brain networks for cognitive control of action in traumatic brain injury. Brain, 135, 1293–1307. CrossRef Google Scholar PubMed
- Caeyenberghs, K., Leemans, A., Leunissen, I., Michiels, K., & Swinnen, S.P. (2013). Topological correlations of structural and functional networks in patients with traumatic brain injury. Frontiers in Human Neuroscience, 7, 726. doi:10.3389/fnhum.2013.00726 CrossRef Google Scholar PubMed
- Castellanos, N.P., Paul, N., Ordonez, V.E., Demuynck, O., Bajo, R., Campo, P., & Maestu, F. (2010). Reorganization of functional connectivity as a correlate of cognitive recovery in acquired brain injury. Brain, 133, 2365–2381. CrossRef Google Scholar PubMed
- Chai, X.J., Castanon, A.N., Ongur, D., & Whitfield-Gabrieli, S. (2012). Anticorrelations in resting state networks without global signal regression. Neuroimage, 59, 1420–1428. CrossRef Google Scholar PubMed
- Chang, C., & Glover, G.H. (2009). Effects of model-based physiological noise correction on default mode network anti-correlations and correlations. Neuroimage, 47, 1448–1459. CrossRef Google Scholar PubMed
- Chen, G., Chen, G., Xie, C., & Li, S.-J. (2011). Negative functional connectivity and its dependence on the shortest path length of positive network in the resting-state human brain. Brain Connectivity, 1, 195–206. CrossRef Google Scholar PubMed
- Corbetta, M., & Shulman, G.L. (2002). Control of goal-directed and stimulus-driven attention in the brain. Nature Reviews Neuroscience, 3, 215–229. CrossRef Google Scholar
- Corrigan, J.D., & Bogner, J. (2007). Initial reliability and validity of the Ohio State University TBI identification method. Journal of Head Trauma Rehabilitation, 22, 318–329. CrossRef Google Scholar
- Cox, R.W. (1996). AFNI: Software for analysis and visualization of functional magnetic resonance neuroimages. Computers and Biomedical Research, 29, 162–173. CrossRef Google Scholar
- Crepeau, F., & Scherzer, P. (1993). Predictors and indicators of work status after traumatic brain injury: A meta-analysis. Neuropsychological Rehabilitation, 3, 5–35. CrossRef Google Scholar
- Delis, D.C., Kaplan, E., & Kramer, J.H. (2001). D-KEFS Executive Function System: Examiners manual. San Antonio, TX: Pearson Education, Inc. Google Scholar
- Diener, E., Emmons, R.A., Larsen, R.J., & Griffin, S. (1985). The satisfaction with life scale. Journal of Personality Assessment, 49, 71–75. CrossRef Google Scholar PubMed
- Dixon, M.L., Fox, K.C.R., & Christoff, K. (2014). A framework for understanding the relationship between externally and internally directed cognition. Neuropsychologia, 62, 321–330. CrossRef Google Scholar PubMed
- Dritschel, B.H., Kogan, L., Burton, A., Burton, E., & Goddard, L. (1998). Everyday planning difficulties following traumatic brain injury: A role for autobiographical memory. Brain Injury, 12, 875–886. Google Scholar PubMed
- Ellamil, M., Dobson, C., Beeman, M., & Christoff, K. (2012). Evaluative and generative modes of thought during the creative process. Neuroimage, 59, 1783–1794. CrossRef Google Scholar PubMed
- Evans, A.C., Collins, D.L., Mills, S.R., Brown, E.D., Kelly, R.L., & Peters, T.M. (1993). 3D statistical neuroanatomical models from 305 MRI volumes. In Proceedings of the IEEE Nuclear Science Symposium and Medical Imaging (pp. 1813–1817). IEEE. doi:10.1109/NSSMIC.1993.373602 Google Scholar
- Fox, M.D., Snyder, A.Z., Vincent, J.L., Corbetta, M., Van Essen, D.C., & Raichle, M.E. (2005). From the cover: The human brain is intrinsically organized into dynamic, anticorrelated functional networks. Proceedings of the National Academy of Sciences of the United States of America, 102, 9673–9678. CrossRef Google Scholar
- Fox, M.D., Zhang, D., Snyder, A.Z., & Raichle, M.E. (2009). The global signal and observed anticorrelated resting state brain networks. Journal of Neurophysiology, 101, 3270–3283. CrossRef Google Scholar
- Gerlach, K.D., Spreng, R.N., Gilmore, A.W., & Schacter, D.L. (2011). Solving future problems: Default network and executive activity associated with goal-directed mental simulations. Neuroimage, 55, 1816–1824. CrossRef Google Scholar PubMed
- Gerlach, K.D., Spreng, R.N., Madore, K.P., & Schacter, D.L. (2014). Future planning: Default network activity couples with frontoparietal control network and reward-processing regions during process and outcome simulations. Social Cognitive and Affective Neuroscience, 9, 1942–1951. CrossRef Google Scholar PubMed
- Ham, T.E., Bonnelle, V., Hellyer, P., Jilka, S., Robertson, I.H., Leech, R., & Sharp, D.J. (2014). The neural basis of impaired self-awareness after traumatic brain injury. Brain, 137, 586–597. CrossRef Google Scholar PubMed
- Han, K., Mac Donald, C.L., Johnson, A.M., Barnes, Y., Wierzechowski, L., Zonies, D., & Brody, D.L. (2014). Disrupted modular organization of resting-state cortical functional connectivity in U.S. military personnel following concussive “mild” blast-related traumatic brain injury. Neuroimage, 84, 76–96. CrossRef Google Scholar PubMed
- Hanten, G., Chapman, S.B., Gamino, J.F., Zhang, L., Benton, S.B., Stallings-Roberson, G., & Levin, H.S. (2004). Verbal selective learning after traumatic brain injury in children. Annals of Neurology, 56, 847–853. CrossRef Google Scholar PubMed
- Hillary, F.G., Rajtmajer, S.M., Roman, C.A., Medaglia, J.D., Slocomb-Dluzen, J.E., Calhoun, V.D., & Wylie, G. R. (2014). The rich get richer: Brain injury elicits hyperconnectivity in core subnetworks. PLoS One, 9, e104021. doi:10.1371/journal.pone.0104021 CrossRef Google Scholar PubMed
- Honey, C.J., Sporns, O., Cammoun, L., Gigandet, X., Thiran, J.P., Meuli, R., & Hagmann, P. (2009). Predicting human resting-state functional connectivity from structural connectivity. Proceedings of the National Academy of Sciences of the United States of America, 106, 2035–2040. CrossRef Google Scholar PubMed
- Jo, H.J., Saad, Z.S., Simmons, W.K., Milbury, L.A., & Cox, R.W. (2010). Mapping sources of correlation in resting state FMRI, with artifact detection and removal. Neuroimage, 52, 571–582. CrossRef Google Scholar PubMed
- Joel, S.E., Caffo, B.S., van Zijl, P.C.M., & Pekar, J.J. (2011). On the relationship between seed-based and ICA-based measures of functional connectivity. Magnetic Resonance in Medicine, 66, 644–657. CrossRef Google Scholar PubMed
- Johnstone, T., Walsh, K.S.O., Greischar, L.L., Alexander, A.L., Fox, A.S., Davidson, R.J., & Oakes, T.R. (2006). Motion correction and the use of motion covariates in multiple-subject fMRI analysis. Human Brain Mapping, 27, 779–788. CrossRef Google Scholar
- Kelly, A.M.C., Uddin, L.Q., Biswal, B.B., Castellanos, F.X., & Milham, M.P. (2008). Competition between functional brain networks mediates behavioral variability. Neuroimage, 39, 527–537. CrossRef Google Scholar PubMed
- Kinnunen, K.M., Greenwood, R., Powell, J.H., Leech, R., Hawkins, P.C., Bonnelle, V., & Sharp, D.J. (2010). White matter damage and cognitive impairment after traumatic brain injury. Brain, 134, 449–463. CrossRef Google Scholar PubMed
- Krawczyk, D.C., de la Plata, C.M., Schauer, G.F., Vas, A.K., Keebler, M., Tuthill, S., & Chapman, S.B. (2013). Evaluating the effectiveness of reasoning training in military and civilian chronic traumatic brain injury patients: Study protocol. Trials, 14, 29. doi:10.1186/1745-6215-14-29 CrossRef Google Scholar PubMed
- Kumar, S., Rao, S.L., Chandramouli, B.A., & Pillai, S.V. (2009). Reduction of functional brain connectivity in mild traumatic brain injury during working memory. Journal of Neurotrauma, 26, 665–675. CrossRef Google Scholar PubMed
- Latora, V., & Marchiori, M. (2001). Efficient behavior of small-world networks. Physical Review Letters, 87, 198701. CrossRef Google Scholar PubMed
- Levine, B., Robertson, I.H., Clare, L., Carter, G., Hong, J., Wilson, B.A., & Stuss, D.T. (2000). Rehabilitation of executive functioning: An experimental-clinical validation of goal management training. Journal of the International Neuropsychological Society, 6, 299–312. CrossRef Google Scholar PubMed
- Levine, B., Stuss, D.T., Milberg, W.P., Alexander, M.P., Schwartz, M., & Macdonald, R. (1998). The effects of focal and diffuse brain damage on strategy application: Evidence from focal lesions, traumatic brain injury and normal aging. Journal of the International Neuropsychological Society, 4, 247–264. Google Scholar PubMed
- Mac Donald, C.L., Johnson, A.M., Cooper, D., Nelson, E.C., Werner, N.J., Shimony, J.S., & Brody, D.L. (2011). Detection of blast-related traumatic brain injury in U.S. military personnel. New England Journal of Medicine, 364, 2091–2100. CrossRef Google Scholar PubMed
- Malec, J.F., Testa, J.A., Rush, B.K., Brown, A.W., & Moessner, A.M. (2007). Self-assessment of impairment, impaired self-awareness, and depression after traumatic brain injury. Journal of Head Trauma Rehabilitation, 22, 156–166. CrossRef Google Scholar PubMed
- Marquez de la Plata, C.D., Garces, J., Shokri Kojori, E., Grinnan, J., Krishnan, K., Pidikiti, R., & Diaz-Arrastia, R. (2011). Deficits in functional connectivity of hippocampal and frontal lobe circuits after traumatic axonal injury. Archives of Neurology, 68, 74–84. CrossRef Google Scholar PubMed
- Mateer, C.A., Sohlberg, M.M., & Crinean, J. (1987). Focus on clinical research: Perceptions of memory function in individuals with closed-head injury. Journal of Head Trauma Rehabilitation, 2, 74–84. CrossRef Google Scholar
- Mayer, A.R., Mannell, M.V., Ling, J., Gasparovic, C., & Yeo, R.A. (2011). Functional connectivity in mild traumatic brain injury. Human Brain Mapping, 32, 1825–1835. CrossRef Google Scholar PubMed
- Messe, A., Caplain, S., Paradot, G., Garrigue, D., Mineo, J.-F., Soto Ares, G., & Lehericy, S. (2011). Diffusion tensor imaging and white matter lesions at the subacute stage in mild traumatic brain injury with persistent neurobehavioral impairment. Human Brain Mapping, 32, 999–1011. CrossRef Google Scholar PubMed
- Murphy, K., Birn, R.M., Handwerker, D.A., Jones, T.B., & Bandettini, P.A. (2009). The impact of global signal regression on resting state correlations: Are anti-correlated networks introduced? Neuroimage, 44, 893–905. CrossRef Google Scholar PubMed
- Nakamura, T., Hillary, F.G., & Biswal, B.B. (2009). Resting network plasticity following brain injury. PLoS One, 4, e8220. doi:10.1371/journal.pone.0008220 CrossRef Google Scholar PubMed
- Niogi, S.N., & Mukherjee, P. (2010). Diffusion tensor imaging of mild traumatic brain injury. Journal of Head Trauma Rehabilitation, 25, 241–255. CrossRef Google Scholar PubMed
- Niogi, S.N., Mukherjee, P., Ghajar, J., Johnson, C., Kolster, R.A., Sarkar, R., & McCandliss, B.D. (2008). Extent of microstructural white matter injury in postconcussive syndrome correlates with impaired cognitive reaction time: A 3T diffusion tensor imaging study of mild traumatic brain injury. AJNR American Journal of Neuroradiology, 29, 967–973. CrossRef Google Scholar PubMed
- O’Reilly, J.X., Croxson, P.L., Jbabdi, S., Sallet, J., Noonan, M.P., Mars, R.B., & Baxter, M.G. (2013). Causal effect of disconnection lesions on interhemispheric functional connectivity in rhesus monkeys. Proceedings of the National Academy of Sciences of the United States of America, 110, 13982–13987. CrossRef Google Scholar PubMed
- Pandit, A.S., Expert, P., Lambiotte, R., Bonnelle, V., Leech, R., Turkheimer, F.E., & Sharp, D.J. (2013). Traumatic brain injury impairs small-world topology. Neurology, 80, 1826–1833. CrossRef Google Scholar PubMed
- Power, J.D., Barnes, K.A., Snyder, A.Z., Schlaggar, B.L., & Petersen, S.E. (2012). Spurious but systematic correlations in functional connectivity MRI networks arise from subject motion. Neuroimage, 59, 2142–2154. CrossRef Google Scholar PubMed
- Power, J.D., Cohen, A.L., Nelson, S.M., Wig, G.S., Barnes, K.A., Church, J.A., & Petersen, S.E. (2011). Functional network organization of the human brain. Neuron, 72, 665–678. CrossRef Google Scholar PubMed
- Power, J.D., Mitra, A., Laumann, T.O., Snyder, A.Z., Schlaggar, B.L., & Petersen, S.E. (2014). Methods to detect, characterize, and remove motion artifact in resting state fMRI. Neuroimage, 84, 320–341. CrossRef Google Scholar PubMed
- Raichle, M.E., MacLeod, A.M., Snyder, A.Z., Powers, W.J., Gusnard, D.A., & Shulman, G.L. (2001). A default mode of brain function. Proceedings of the National Academy of Sciences of the United States of America, 98, 676–682. CrossRef Google Scholar PubMed
- Rigon, A., Duff, M.C., McAuley, E., Kramer, A., & Voss, M.W. (2015). “Is traumatic brain injury associated with reduced inter-hemispheric functional connectivity? A study of large-scale resting state networks following traumatic brain injury.” Journal of Neurotrauma, doi:10.1089/neu.2014.3847 [Epub ahead of print]. Google Scholar
- Robertson, I.H., Manly, T., Andrade, J., Baddeley, B.T., & Yiend, J. (1997). ‘Oops!’: Performance correlates of everyday attentional failures in traumatic brain injured and normal subjects. Neuropsychologia, 35, 747–758. CrossRef Google Scholar
- Rubinov, M., & Sporns, O. (2010). Complex network measures of brain connectivity: Uses and interpretations. Neuroimage, 52, 1059–1069. CrossRef Google Scholar PubMed
- Saad, Z.S., Gotts, S.J., Murphy, K., Chen, G., Jo, H.J., Martin, A., & Cox, R.W. (2012). Trouble at rest: How correlation patterns and group differences become distorted after global signal regression. Brain Connectivity, 2, 25–32. CrossRef Google Scholar PubMed
- Satterthwaite, T.D., Wolf, D.H., Loughead, J., Ruparel, K., Elliott, M.A., Hakonarson, H., & Gur, R.E. (2012). Impact of in-scanner head motion on multiple measures of functional connectivity: Relevance for studies of neurodevelopment in youth. Neuroimage, 60, 623–632. CrossRef Google Scholar
- Schönberger, M., Ponsford, J., Reutens, D., Beare, R., Clarke, D., & O’Sullivan, R. (2011). The relationship between mood disorders and MRI findings following traumatic brain injury. Brain Injury, 25, 543–550. CrossRef Google Scholar PubMed
- Seeley, W.W., Menon, V., Schatzberg, A.F., Keller, J., Glover, G.H., Kenna, H., & Greicius, M.D. (2007). Dissociable intrinsic connectivity networks for salience processing and executive control. Journal of Neuroscience, 27, 2349–2356. CrossRef Google Scholar PubMed
- Shallice, T. (1982). Specific impairments of planning. Philosophical Transactions of the Royal Society B: Biological Sciences, 298, 199–209. CrossRef Google Scholar PubMed
- Sharp, D.J., Beckmann, C.F., Greenwood, R., Kinnunen, K.M., Bonnelle, V., De Boissezon, X., & Leech, R. (2011). Default mode network functional and structural connectivity after traumatic brain injury. Brain, 134, 2233–2247. CrossRef Google Scholar
- Sharp, D.J., Scott, G., & Leech, R. (2014). Network dysfunction after traumatic brain injury. Nature Reviews Neurology, 10, 156–166. CrossRef Google Scholar
- Singh, M., Jeong, J., Hwang, D., Sungkarat, W., & Gruen, P. (2010). Novel diffusion tensor imaging methodology to detect and quantify injured regions and affected brain pathways in traumatic brain injury. Magnetic Resonance Imaging, 28, 22–40. CrossRef Google Scholar PubMed
- Slobounov, S.M., Gay, M., Zhang, K., Johnson, B., Pennell, D., Sebastianelli, W., & Hallett, M. (2011). Alteration of brain functional network at rest and in response to YMCA physical stress test in concussed athletes: RsFMRI study. Neuroimage, 55, 1716–1727. CrossRef Google Scholar PubMed
- Smallwood, J., Brown, K., Baird, B., & Schooler, J.W. (2012). Cooperation between the default mode network and the frontal–parietal network in the production of an internal train of thought. Brain Research, 1428, 60–70. CrossRef Google Scholar
- Smith, D.H., Meaney, D.F., & Shull, W.H. (2003). Diffuse axonal injury in head trauma. Journal Head Trauma Rehabilitation, 18, 307–316. CrossRef Google Scholar PubMed
- Smits, M., Houston, G.C., Dippel, D.W.J., Wielopolski, P.A., Vernooij, M.W., Koudstaal, P.J., & van der Lugt, A. (2011). Microstructural brain injury in post-concussion syndrome after minor head injury. Neuroradiology, 53, 553–563. CrossRef Google Scholar PubMed
- Sours, C., Rosenberg, J., Kane, R., Roys, S., Zhuo, J., Shanmuganathan, K., & Gullapalli, R.P. (2015). Associations between interhemispheric functional connectivity and the Automated Neuropsychological Assessment Metrics (ANAM) in civilian mild TBI. Brain Imaging and Behavior, 9, 190–203. CrossRef Google Scholar PubMed
- Sours, C., Zhuo, J., Janowich, J., Aarabi, B., Shanmuganathan, K., & Gullapalli, R.P. (2013). Default mode network interference in mild traumatic brain injury – A pilot resting state study. Brain Research, 1537, 201–215. CrossRef Google Scholar PubMed
- Spreng, R.N., Sepulcre, J., Turner, G.R., Stevens, W.D., & Schacter, D.L. (2013). Intrinsic architecture underlying the relations among the default, dorsal Attention, and frontoparietal control networks of the human brain. Journal of Cognitive Neuroscience, 25, 74–86. CrossRef Google Scholar PubMed
- Spreng, R.N., Stevens, W.D., Chamberlain, J.P., Gilmore, A.W., & Schacter, D.L. (2010). Default network activity, coupled with the frontoparietal control network, supports goal-directed cognition. Neuroimage, 53, 303–317. CrossRef Google Scholar PubMed
- Tang, L., Ge, Y., Sodickson, D.K., Miles, L., Zhou, Y., Reaume, J., & Grossman, R.I. (2011). Thalamic resting-state functional networks: Disruption in patients with mild traumatic brain injury. Radiology, 260, 831–840. CrossRef Google Scholar PubMed
- Teasdale, G., & Jennett, B. (1974). Assessment of coma and impaired consciousness. A practical scale. Lancet, 2, 81–84. CrossRef Google Scholar PubMed
- van Dijk, K.R.A., Hedden, T., Venkataraman, A., Evans, K.C., Lazar, S.W., & Buckner, R.L. (2010). Intrinsic functional connectivity as a tool for human connectomics: Theory, properties, and optimization. Journal of Neurophysiology, 103, 297–321. CrossRef Google Scholar
- van Dijk, K.R.A., Sabuncu, M.R., & Buckner, R.L. (2012). The influence of head motion on intrinsic functional connectivity MRI. Neuroimage, 59, 431–438. CrossRef Google Scholar PubMed
- Vincent, J.L., Kahn, I., Snyder, A.Z., Raichle, M.E., & Buckner, R.L. (2008). Evidence for a frontoparietal control system revealed by intrinsic functional connectivity. Journal of Neurophysiology, 100, 3328–3342. CrossRef Google Scholar PubMed
- Weathers, F., Litz, B., Herman, D., Huska, J., & Keane, T. (1993). The PTSD Checklist (PCL): Reliability, Validity, and Diagnostic Utility. In Annual Meeting of the International Society of Traumatic Stress Studies. San Antonio, TX: International Society for Traumatic Stress Studies. Google Scholar
- Wechsler, D. (1997). Wechsler Adult Intelligence Scale – Third Edition (WAIS–III). San Antonio, TX: Pearson Education, Inc. Google Scholar
- Wechsler, D. (1999). Wechsler Abbreviated Scale of Intelligence (WASI). San Antonio, TX: Pearson Education, Inc. Google Scholar
- Wechsler, D. (2001). Wechsler test of adult reading. San Antonio, TX: The Psychological Corporation. Google Scholar
- Wechsler, D. (2008). Wechsler Memory Scale – Fourth Edition (WMS-IV). San Antonio, TX: Pearson Education, Inc. Google Scholar
- Whyte, J., Polansky, M., Cavallucci, C., Fleming, M., Lhulier, J., & Coslett, H.B. (1996). Inattentive behavior after traumatic brain injury. Journal of the International Neuropsychological Society, 2, 274–281. CrossRef Google Scholar PubMed
- Wilson, J.T.L., Pettigrew, L.E.L., & Teasdale, G.M. (1998). Structured interviews for the Glasgow outcome scale and the extended Glasgow outcome scale: Guidelines for their use. Journal of Neurotrauma, 15, 573–585. CrossRef Google Scholar
- Xia, M., Wang, J., & He, Y. (2013). BrainNet viewer: A network visualization tool for human brain connectomics. PLoS One, 8, e68910. doi:10.1371/journal.pone.0068910 CrossRef Google Scholar PubMed
- Zalesky, A., Fornito, A., & Bullmore, E.T. (2010). Network-based statistic: Identifying differences in brain networks. Neuroimage, 53, 1197–1207. CrossRef Google Scholar PubMed
- Zgaljardic, D.J., Seale, G.S., Schaefer, L.A., Temple, R.O., Foreman, J., & Elliott, T.R. (2015). Psychiatric disease and post-acute traumatic brain injury. Journal of Neurotrauma, 32, 1911–1925. CrossRef Google Scholar PubMed