3 CE Credits. Special Issue on Prediction (JINS 22:10, 2016): CE Bundle 2
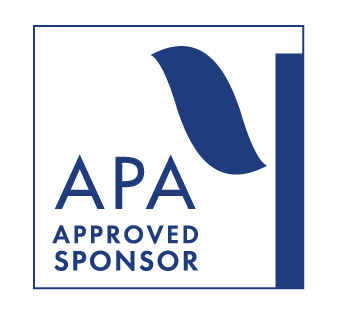
- Describe the similarities and differences in neurocognition between individuals with different psychotic disorders and their relatives.
- Identify potential advantages and disadvantages of intra-individual cognitive variability (IICV) as a marker of risk of subsequent cognitive impairment.
- Select the most powerful index tests to identify students at risk for perceived academic problems following concussion.
Target Audience: | Intermediate |
---|---|
Availability: | Date Available: 2016-11-30 |
You may obtain CE for this JINS package at any time. | |
Offered for CE | Yes |
Cost | Members $30 |
Non-Members $45 | |
Refund Policy | This JINS package is not eligible for refunds |
CE Credits | 3.0 |
Prediction of behavior and related outcomes has long been a principal goal of psychology. The development of intelligence tests in the early 20th century led to new strategies for predicting academic and occupational performance (Sternberg, 1996). Similarly, in the mid-19th century, Fechner’s use of mathematical models for prediction of behavior (Wertheimer, 1987) initiated a long tradition that has continued to the present day, which can be seen in the mathematical models of learning developed by Clark Hull (Hull, 1943), dynamic models of the development of spatial memory abilities (Spencer, Smith, & Thelen, 2001), and others. These ideas also led to information processing theories of memory (Atkinson & Shiffrin, 1968; Raaijmakers & Shiffrin, 1980), which have been used to predict specific stages of memory processing that are impaired in patient groups (Brown et al., 1995; Brown, Woodard, & Rich, 1994). Recently, use of prediction strategies in neuropsychology has turned toward development of new approaches for identification and characterization of the development and disease course of neurocognitive and neuropsychiatric disorders.
Prediction involves making a statement about an event that is uncertain, typically based on some type of known information. Although the term “prediction” brings to mind the forecasting of some future outcome or event, the term prediction can also be used for concurrent diagnostic purposes. Since approximately 2000, the number of research studies focused on prediction of diagnosis and clinical trajectories has increased dramatically. Figure 1 shows the number of publications per year returned from a simple search of the terms “preclinical prediction” in the National Library of Medicine (PubMed) database from 1950 to October 1, 2016. Studies of preclinical prediction in neuropsychology have typically focused on identification of persons at the highest risk for specific cognitive conditions. Early identification of risk, or of subtle signs of incipient disease, opens the possibility for treatments to prevent disease development, or to delay onset or slow progression of clinically significant symptoms. Prediction studies after brain injury have also been important for prognosticating the trajectory of recovery and for planning resource allocation for treatment.
State-of-the-art prediction strategies have been facilitated by at least two significant developments over the past 25 years. First, the availability of high-speed computers and software capable of performing complex statistical analyses has supported the development and validation of complex theoretical predictive models. For instance, machine learning approaches to prediction would not be possible without powerful computing resources (Hey, 2010). Machine learning has been successfully used in the context of diagnosis (Bigler, 2013; Mundt, Freed, & Greist, 2000; Teipel, Meindl, Grinberg, Heinsen, & Hampel, 2008) and prognosis (Gutman et al., 2015; Koutsouleris et al., 2009; Moradi, Pepe, Gaser, Huttunen, & Tohka, 2015; Schmidt-Richberg et al., 2016). Second, the identification of biomarkers of a variety of neurological and psychiatric conditions has provided a set of predictors that are highly sensitive to risk factors and pathological changes leading to these conditions (Chong, Lim, & Sahadevan, 2006; Craig-Schapiro, Fagan, & Holtzman, 2009; Mayeux, 2004; Sharma & Laskowitz, 2012; Shaw, Korecka, Clark, Lee, & Trojanowski, 2007). Such biomarkers can be used to test predictions regarding possible etiologies associated with neuropsychological abnormalities (Ivanoiu et al., 2015; Miller et al., 2008; Wirth et al., 2013). Improved genetic testing has also contributed to more accurate predictions of functional changes when combined with neuropsychological and imaging data (O’Hara et al., 1998; Reiman et al., 2004; Small et al., 1996). Some researchers have argued, based on the literature, that “neuromarkers often provide better predictions (neuroprognosis), alone or in combination with other measures, than traditional behavioral measures” (page 11, Gabrieli, Ghosh, & Whitfield-Gabrieli, 2015). As data increasingly emerge, our field will be enriched by identifying the best combinations of measures to predict disease.
Many neurocognitive disorders are known to evolve from a presymptomatic to mildly clinical state to a fully clinical disorder. In essence, they go through different biological and clinical “stages” (McGorry et al., 2007). Two disorders receiving a great deal of research over the past two decades are Alzheimer’s disease (AD) and schizophrenia. For example, AD is preceded by mild cognitive impairment (MCI), and in many cases schizophrenia is preceded by a clinical high-risk (CHR) state identified by attenuated positive psychotic symptoms (i.e., mild delusions and hallucinations with some degree of intact reality testing; Tsuang et al., 2013; Yung & McGorry, 1996). At least in principle, identifying predictors and mechanisms of transition to AD or to psychosis among individuals showing signs of incipient neurocognitive disorders are critical steps in the search for preventive or early intervention strategies (Woodberry, Shapiro, Bryant, & Seidman, 2016). Interest in early detection and prevention of schizophrenia and other psychotic disorders has led to more than a decade of work studying young people who may be at risk of developing a psychotic illness, and advances have been made in prediction of transition to psychosis from a CHR stage (Cannon et al., 2008, 2016; Carrion et al., 2016), including the usage of neuropsychological measures (Giuliano et al., 2012; Seidman, Giuliano, & Walker, 2010; Seidman et al., 2016).
Studies investigating early detection of neurological and psychiatric conditions have improved understanding of etiology and diagnosis significantly, and they have opened new avenues for management. Presymptomatic detection is also essential to the development of effective intervention strategies, as it provides a window for preventing/delaying onset or reducing severity. This special issue of the Journal of the International Neuropsychological Society includes nine papers describing cutting-edge empirical findings that exemplify key methodological advances for preclinical detection of a variety of neurological, neurodevelopmental, and neuropsychiatric conditions. Methodological approaches taken include assessment of familial and genetic risk analyses, phenotypic characterization using cognitive and/or imaging methods, and evaluation of biomarker effectiveness. These papers provide substantive integrative and synthetic summaries of the current status of preclinical detection methodologies and future directions for the field.
As novel biomarkers of early, disease-related changes are identified, strategies for making optimal use of this information are becoming increasingly important. In this special issue, several papers focus on description of novel methodologies for combining biomarker data with other clinical information for diagnosis or prognosis. In a thorough literature review, Cooper and colleagues describe state-of-the-art objective biomarkers in prodromal Parkinson’s disease (PD), and they discuss several strategies for combining these biomarkers with clinical and genetic data for improving sensitivity and specificity for identification of persons with prodromal PD. Soldan and colleagues demonstrate that beta-amyloid and phosphorylated tau measured in cerebrospinal fluid can predict cognitive functioning as long as 10 years later. Using data from the Alzheimer’s Disease Neuroimaging Initiative, Edmonds and colleagues demonstrate how a novel method for staging preclinical AD using amyloid positron emission tomography (PET) imaging can be combined with detailed cognitive assessment to better characterize preclinical AD. Notably, this study found that considerable amyloid accumulation had already occurred before clinical diagnosis. Finally, in a cross-sectional study, Quenon and colleagues demonstrate the relationships between imaging measures of extent of early AD neuropathology, as indexed by in vivo neuroimaging biomarkers (amyloid PET, hippocampal volume, and measures of cortical thickness) and level of memory performance on the Free and Cued Selective Reminding Test. Although biomarkers of early AD neuropathology predicted overall memory performance, cueing efficiency, which is frequently impaired in AD, demonstrated particularly strong relationships with cortical thickness of regions that are commonly atrophic in early AD.
Assessment of the influence of familial and genetic risk factors has also become an important tool for forecasting diagnostic status. The family high-risk approach allows a defined selection process for ascertaining non-ill subjects in a family in which there is an identified proband with the illness. An advantage of such an approach is that it is not dependent on symptom expression, but rather genetic risk, and thus an unaffected individual could be studied at any age, enabling developmentally guided probes of risk (Agnew-Blais & Seidman, 2013). The “unaffected relatives” are typically offspring or siblings who are considered to be at higher risk for the illness or for phenotypes associated with the illness, because they carry approximately 50% of the genes for the illness. This approach has been used for over half a century, and has been one of the most fruitful ways of identifying components of the vulnerability to various illnesses, particularly schizophrenia. The most typical outcome used in many of these studies originally was “developing the illness” (e.g., schizophrenia, AD, etc.). However, outcomes can also be expressed in a range of phenotypes reflecting the underlying disorder, and outcomes such as functional disability are also very important. A wide range of phenotypes (e.g., working memory or attention problems, smaller hippocampi) can be studied at different ages to evaluate developmental effects, and in different sub-populations (e.g., those with higher vs. lower genetic loading) to study the specific subgroup expression of the phenotypes.
In this issue, Lancaster and colleagues demonstrate that baseline diffusion tensor imaging of the white matter microstructure in the medial temporal lobe can predict longitudinal changes in episodic memory functioning over 3 years in a sample of cognitively healthy older adults with an enriched familial and genetic risk for AD. Koscik and colleagues compare sensitivity for predicting subsequent cognitive impairment using either variability in performance across cognitive tasks or combinations of outcomes from particular tasks (e.g., memory and executive tasks) taken at baseline several years earlier. In an investigation of neuropsychological endophenotypes of familial risk for schizophrenia and affective psychosis, Seidman and colleagues found that working memory impairment was more robust than vigilance for characterizing the cognitive impairment associated with familial risk for schizophrenia. Although persons with familial risk for affective psychosis showed more impaired vigilance relative to other groups, this effect was eliminated after adjustment for several psychopathological symptoms. This work was part of an agenda to identify the most sensitive and specific neuropsychological predictors of risk for different forms of psychosis (see also Seidman et al., 2016). Each of these studies demonstrates novel methodologies for studying the influence of familial and genetic risk for possible diagnosis and prognosis.
Finally, two articles in this issue focus on the use of prediction strategies for prognosticating outcome after brain injury has already occurred in pediatric samples. Ransom and colleagues use evidence-based assessment (EBA) to identify teenage students who are at-risk for post-concussive academic difficulty. Self-reported post-injury symptoms and executive functioning difficulty, rather than parent-reported sequelae, showed the strongest relationships with perceived post-injury academic difficulties. This study demonstrates the utility of the EBA framework within the context of neuropsychological assessment. Till and colleagues studied cognitive, academic, and psychosocial difficulties experienced by children diagnosed with an acquired demyelinating syndrome (ADS), one-third of whom were later diagnosed with multiple sclerosis (MS), over a 6-month follow-up period. Children with ADS were shown to demonstrate a favorable neurocognitive outcome in the short-term, including children diagnosed with MS.
In summary, the papers in this Special Issue present several novel approaches toward developing methodologies for prediction in neuropsychology. Research on optimizing the information obtained from biomarkers will undoubtedly continue to be stimulated by the identification of new biomarkers in the future. Introduction of new frameworks for assessment, such as EBA, and other strategies for evaluating longitudinal cognitive, clinical, and neuroimaging changes in outcome, as presented by several studies in this special issue, will also be helpful for moving the field forward. Capitalization on new developments in genetic analyses and assessment of familial risk factors will also be important tools for improving predictive accuracy.
Nevertheless, we also face challenges with respect to definition of appropriate statistical models used for assessment of change, growth, or decline (Cronbach & Furby, 1970; Francis, Fletcher, Stuebing, Davidson, & Thompson, 1991; Gottman & Rushe, 1993; Harrell, 2015; Singer & Willett, 2003; Steyerberg, 2009; Steyerberg & Harrell, 2016; Steyerberg et al., 2010; Temkin, Heaton, Grant, & Dikmen, 1999). While these issues are certainly not new, continued focus on improving definitions of the change that we are predicting, and on models for assessing the effectiveness of variables predicting this change, are certainly warranted. Despite these challenges, research on preclinical prediction continues to grow, and future studies promise to contribute to improvement in preventative treatments before cognitive decline occurs as well as to more effective treatments and allocation of resources following brain injury.
The degree of overlap between schizophrenia (SCZ) and affective psychosis (AFF) has been a recurring question since Kraepelin’s subdivision of the major psychoses. Studying nonpsychotic relatives allows a comparison of disorder-associated phenotypes, without potential confounds that can obscure distinctive features of the disorder. Because attention and working memory have been proposed as potential endophenotypes for SCZ and AFF, we compared these cognitive features in individuals at familial high-risk (FHR) for the disorders.
Young, unmedicated, first-degree relatives (ages, 13–25 years) at FHR-SCZ (n=41) and FHR-AFF (n=24) and community controls (CCs,n=54) were tested using attention and working memory versions of the Auditory Continuous Performance Test. To determine if schizotypal traits or current psychopathology accounted for cognitive deficits, we evaluated psychosis proneness using three Chapman Scales, Revised Physical Anhedonia, Perceptual Aberration, and Magical Ideation, and assessed psychopathology using the Hopkins Symptom Checklist -90 Revised.
Compared to controls, the FHR-AFF sample was significantly impaired in auditory vigilance, while the FHR-SCZ sample was significantly worse in working memory. Both FHR groups showed significantly higher levels of physical anhedonia and some psychopathological dimensions than controls. Adjusting for physical anhedonia, phobic anxiety, depression, psychoticism, and obsessive-compulsive symptoms eliminated the FHR-AFF vigilance effects but not the working memory deficits in FHR-SCZ.
The working memory deficit in FHR-SZ was the more robust of the cognitive impairments after accounting for psychopathological confounds and is supported as an endophenotype. Examination of larger samples of people at familial risk for different psychoses remains necessary to confirm these findings and to clarify the role of vigilance in FHR-AFF. (JINS, 2016,22, 1026–1037)
- Agnew-Blais, J., & Seidman, L.J. (2013). Neurocognition in youth and young adults under age 30 at familial risk for schizophrenia: A quantitative and qualitative review. Cognitive Neuropsychiatry, 18, 44–82. CrossRef Google Scholar
- Agnew-Blais, J., Buka, S.L., Fitzmaurice, G.M, Smoller, J.W., Goldstein, J.M., & Seidman, L.J. (2015). Early childhood IQ trajectories of adults with schizophrenia and affective psychoses in the New England Family Studies. Schizophrenia Bulletin, 41, 817–823. CrossRef Google Scholar
- American Psychiatric Association. (1994). Diagnostic and statistical manual of mental disorders(4th ed.), Washington, DC: American Psychiatric Association Press. Google Scholar
- Baddeley, A.D., & Hitch, G.J. (1974). Working memory. In G.H. Bower (Ed.), The psychology of learning and motivation: Advances in research and theory (pp. 47–90). New York: Academic Press. Google Scholar
- Baune, B.T., Miller, R., McAfoose, J., Johnson, M., Quirk, F., & Mitchell, D. (2010). The role of cognitive impairment in general functioning in major depression. Psychiatry Research, 176, 183–189. CrossRef Google Scholar PubMed
- Bearden, C.E., Hoffman, K.M., & Cannon, T.D. (2001). The neuropsychology and neuroanatomy of bipolar affective disorder: A critical review. Bipolar Disorders, 3, 106–150. CrossRef Google Scholar PubMed
- Bearden, C.E., Glahn, D.C., Monkul, E.S., Barrett, J., Najt, P.N., Villarreal, V., & Soares, J.C. (2006). Patterns of memory impairment in bipolar disorder and unipolar major depression. Psychiatry Research, 142, 139–150. CrossRef Google Scholar PubMed
- Belanoff, J.K., Kalehzan, M., Sund, B., Fleming Ficek, S.K., & Schatzberg, A.F. (2001). Cortisol activity and cognitive changes in psychotic major depression. American Journal of Psychiatry, 158, 1612–1616. CrossRef Google Scholar
- Berrettini, W.H. (2000). Are schizophrenic and bipolar disorders related? A review of family and molecular studies. Biological Psychiatry, 48, 531–538. CrossRef Google Scholar
- Bora, E., Yücel, M., & Pantelis, C. (2009). Cognitive endophenotypes of bipolar disorder: A meta-analysis of neuropsychological deficits in euthymic patients and their first-degree relatives. Journal of Affective Disorders, 113, 1–20. CrossRef Google Scholar PubMed
- Bora, E., Yücel, M., & Pantelis, C. (2010). Cognitive impairment in affective psychoses: A meta-analysis. Schizophrenia Bulletin, 36, 112–125. CrossRef Google Scholar PubMed
- Bora, E., Lin, A., Wood, S.J, Yung, A.R., McGorry, P.D., & Pantelis, C. (2014). Cognitive deficits in youth with familial and clinical high risk to psychosis: A systematic review and meta-analysis. Acta Psychiatrica Scandinavica, 130, 1–15. CrossRef Google Scholar PubMed
- Burdick, K.E., Goldberg, J.F., Harrow, M., Faull, R.N., & Malhotra, A.K. (2006). Neurocognition as a stable endophenotype in bipolar disorder and schizophrenia. Journal of Nervous and Mental Disease, 194, 255–260. CrossRef Google Scholar
- Burdick, K.E., Gunawardane, N., Woodberry, K., & Malhotra, A.K. (2009). The role of general intelligence as an intermediate phenotype for neuropsychiatric disorders. Cognitive Neuropsychiatry, 14, 299–311. CrossRef Google Scholar PubMed
- Burdick, K.E., Goldberg, T.E., Cornblatt, B.A., Keefe, R.S., Gopin, C.B., DeRosse, P., & Malhotra, A.K (2011). The MATRICS Consensus Cognitive Battery in patients with bipolar I disorder. Neuropsychopharmacology, 36, 1587–1592. CrossRef Google Scholar
- Burdick, K.E., Russo, M., Frangou, S., Mahon, K., Braga, R.J, Shanahan, M., & Malhotra, A.K. (2014). Empirical evidence for discrete neurocognitive subgroups in bipolar disorder: Clinical implications. Psychological Medicine, 44, 3083–3096. CrossRef Google Scholar PubMed
- Cahill, C.M., Green, M.J., Jairam, R., & Malhi, G.S. (2007). Do cognitive deficits in juvenile bipolar disorder persist into adulthood? Journal of Nervous and Mental Disease, 195, 891–896. CrossRef Google Scholar PubMed
- Chapman, L.J., & Chapman, J.P. (1978). The measurement of differential deficit. Journal of Psychiatric Research, 14, 303–311. CrossRef Google Scholar
- Chapman, L.J., Chapman, J.P., & Raulin, M.L. (1976). Scales for physical and social anhedonia. Journal of Abnormal Psychology, 85, 374–382. CrossRef Google Scholar PubMed
- Chapman, L.J., Chapman, J.P., & Raulin, M.L. (1978). Perceptual aberration in schizophrenia. Journal of Abnormal Psychology, 87, 399–407. CrossRef Google Scholar
- Cohen, J.D., Barch, D.M., Carter, C., & Servan-Schreiber, D. (1999). Context-processing deficits in schizophrenia: Converging evidence from three theoretically motivated cognitive tasks. Journal of Abnormal Psychology, 108, 120–133. CrossRef Google Scholar
- Cornblatt, B., Risch, N.J., Faris, G., Friedman, D., & Erlenmeyer-Kimling, L. (1988). The Continuous Performance Test, identical pairs version (CPT-IP): I. New findings about sustained attention in normal families. Psychiatry Research, 26, 223–238. CrossRef Google Scholar PubMed
- Craddock, N., O’Donovan, M.C., & Owen, M.J. (2006). Genes for schizophrenia and bipolar disorder? Implications for psychiatric nosology. Schizophrenia Bulletin, 32, 9–16. CrossRef Google Scholar
- Cross-Disorder Group of the Psychiatric GWAS Consortium. (2013). Identification of risk loci with shared effects on five major psychiatric disorders: A genome-wide analysis. The Lancet, 381, 1371–1379. CrossRef Google Scholar PubMed
- Dean, K., Stevens, H., Mortensen, P.B., Murray, R.M., Walsh, E., & Pedersen, C.B. (2010). Full spectrum of psychiatric outcomes among offspring with parental history of mental disorder. Archives of General Psychiatry, 67, 822–829. CrossRef Google Scholar PubMed
- Dennis, M., Francis, D.J., Cirino, P.T., Schachar, R., Barnes, M.A., & Fletcher, J.A. (2009). Why IQ is not a covariate in cognitive studies of neurodevelopmental disorders. Journal of the International Neuropsychological Society, 15, 331–343. CrossRef Google Scholar
- Derogatis, L.R. (1993). Symptom Checklist-90-R (SCL-90R). Minneapolis, MN: National Computer Systems. Google Scholar
- Dickstein, D.P., Treland, J.E., Snow, J., McClure, E.B., Mehta, M.S., Towbin, K.E., & Leibenluft, E. (2004). Neuropsychological performance in pediatric bipolar disorder. Biological Psychiatry, 55, 32–39. CrossRef Google Scholar
- Diwadkar, V.A., Goradia, D., Hosanagar, A., Mermon, D., Montrose, D.M., Birmaher, B., & Keshavan, M.S. (2011). Working memory and attention deficits in adolescent offspring of schizophrenia or bipolar patients: Comparing vulnerability markers. Progress in Neuro-Psychopharmacology and Biological Psychiatry, 35, 1349–1354. CrossRef Google Scholar
- Doyle, A.E., Wilens, T.E., Kwon, A., Seidman, L.J., Faraone, S.V., Fried, R., & Biederman, J. (2005). Neuropsychological functioning in youth with bipolar disorder. Biological Psychiatry, 58, 540–548. CrossRef Google Scholar PubMed
- Doyle, A.E., Wozniak, J., Wilens, T.E., Henin, A., Seidman, L.J., Petty, C., & Biederman, J. (2009). Neurocognitive impairment in unaffected siblings of youth with bipolar disorder. Psychological Medicine, 39, 1253–1263. CrossRef Google Scholar
- Eckblad, M., & Chapman, L.J. (1983). Magical ideation as an indicator of schizotypy. Journal of Consulting and Clinical Psychology, 51, 215–255. CrossRef Google Scholar PubMed
- Erlenmeyer-Kimling, L., Cornblatt, B.A., Rock, D., Roberts, S., Bell, M., & West, A. (1993). The New York High-Risk Project: Anhedonia, attentional deviance, and psychopathology. Schizophrenia Bulletin, 19, 141–153. CrossRef Google Scholar PubMed
- Erlenmeyer-Kimling, L., Rock, D., Roberts, S.A., Janal, M., Kestenbaum, C., Cornblatt, B., & Gottesman, I.I. (2000). Attention, memory, and motor skills as childhood predictors of schizophrenia-related psychoses: The New York High-Risk Project. American Journal of Psychiatry, 157, 1416–1422. CrossRef Google Scholar PubMed
- Ettinger, U., Mohr, C., Gooding, D.C., Cohen, A.S., Rapp, A., Haenschel, C., & Park, S. (2015). Cognition and brain function in schizotypy: A selective review. Schizophrenia Bulletin, 41(Suppl. 2), S417–S426. CrossRef Google Scholar
- Faraone, S.V., Seidman, L.J., Kremen, W.S., Pepple, J.R., Lyons, M.J., & Tsuang, M.T. (1995). Neuropsychological functioning among the nonpsychotic relatives of schizophrenic patients: A diagnostic efficiency analysis. Journal of Abnormal Psychology, 104, 286–304. CrossRef Google Scholar PubMed
- Ferrier, I.N., Stanton, B.R., Kelly, T.P., & Scott, J. (1999). Neuropsychological function in euthymic patients with bipolar disorder. The British Journal of Psychiatry, 175, 246–251. CrossRef Google Scholar PubMed
- Fleming, S.K., Blasey, C., & Schatzberg, A.F. (2004). Neuropsychological correlates of psychotic features in major depressive disorders: A review and meta-analysis. Journal of Psychiatric Research, 38, 27–35. CrossRef Google Scholar PubMed
- Fusar-Poli, P., Deste, G., Smieskova, R., Barlati, G., Yung, A.R., Howes, O., & Borgwardt, S. (2012). Cognitive functioning in prodromal psychosis: A meta-analysis. Archives of General Psychiatry, 69, 1–10. CrossRef Google Scholar PubMed
- Geller, B., Zimmerman, B., Williams, M., & Frazier, J. (1994). WASH-UKSADS: Washington University at St. Louis Kiddie and Young Adult Schedule for Affective Disorders and Schizophrenia—Lifetime and Present Episode Version for DSM-IV. St. Louis, MO: Washington University School of Medicine. Google Scholar
- Gitlin, M.J., Swendsen, J., Heller, T.L., & Hammen, C. (1995). Relapse and impairment in bipolar disorder. American Journal of Psychiatry, 152, 635–640. Google Scholar PubMed
- Giuliano, A.J., Li, H., Mesholam-Gately, R.I., Sorenson, S.M., Woodberry, K.A., & Seidman, L.J. (2012). Neurocognition in the psychosis risk syndrome: A quantitative and qualitative review. Current Pharmaceutical Design, 18, 399–415. CrossRef Google Scholar PubMed
- Glahn, D.C., Bearden, C.E., Barguil, M., Barrett, J., Reichenberg, A., Bowden, C.L., & Velligan, D.I. (2007). The neurocognitive signature of psychotic bipolar disorder. Biological Psychiatry, 62, 910–916. CrossRef Google Scholar PubMed
- Glatt, S.J., Stone, W.S., Faraone, S.V., Seidman, L.J., & Tsuang, M.T. (2006). Psychopathology, personality traits, and social development of young first-degree relatives of schizophrenia patients. British Journal of Psychiatry, 189, 337–345. CrossRef Google Scholar
- Goldman-Rakic, P.S. (1991). Prefrontal cortical dysfunction in schizophrenia: The relevance of working memory. In B.J. Carroll & J.E. Barnett (Eds.), Psychopathology and the brain (pp. 1–23). New York, NY: Raven Press. Google Scholar
- Goldstein, J.M., Buka, S.L., Seidman, L.J., & Tsuang, M.T. (2010). Specificity of familial transmission of schizophrenia psychosis spectrum and affective psychoses in the New England family study’s high-risk design. Archives of General Psychiatry, 67, 458–467. CrossRef Google Scholar
- Gooding, D.C., Matts, C.W., & Rollmann, E.A. (2006). Sustained attention deficits in relation to psychometrically identified schizotypy: Evaluating a potential endophenotypic marker. Schizophrenia Research, 82, 27–37. CrossRef Google Scholar
- Gruber, S.A., Rosso, I.M., & Yurgelun-Todd, D. (2008). Neuropsychological performance predicts clinical recovery in bipolar patients. Journal of Affective Disorders, 105, 253–260. CrossRef Google Scholar
- Gur, R.E., Calkins, M.E., Gur, R.C., Horan, W.P., Nuechterlein, K.H., Seidman, L.J., & Stone, W.S. (2007). The consortium on the genetics of schizophrenia: Neurocognitive endophenotypes. Schizophrenia Bulletin, 33, 49–68. CrossRef Google Scholar
- Heinrichs, R., & Zakzanis, K. (1998). Neurocognitive deficit in schizophrenia: A quantitative review of the evidence. Neuropsychology, 12, 426–445. CrossRef Google Scholar
- Hill, S.K., Reilly, J.L., Keefe, R.S.E., Gold, J.M., Bishop, J.R., Gershon, E.S., & Sweeney, J.A. (2013). Neuropsychological impairments in schizophrenia and psychotic bipolar disorder: Findings from the Bipolar-Schizophrenia Network on Intermediate Phenotypes (B-SNIP) Study. American Journal of Psychiatry, 170, 1275–1284. CrossRef Google Scholar
- Hollingshead, A.B. (1975). Four factor index of social status. New Haven, CT: Yale University Department of Sociology. Google Scholar
- Huang, S., Seidman, L.J., Rossi, S., & Ahveninen, J. (2013). Distinct cortical networks activated by auditory attention and working memory load. Neuroimage, 83, 1098–1108. CrossRef Google Scholar PubMed
- Ivleva, E.I., Bidesi, A.S., Keshavan, M.S., Pearlson, G.D., Meda, S.A., Dodig, D., & Tamminga, C.A. (2013). Gray matter volume as an intermediate phenotype for psychosis: Bipolar-Schizophrenia Network on Intermediate Phenotypes (B-SNIP). American Journal of Psychiatry, 170, 1285–1296. CrossRef Google Scholar
- Keefe, R.S.E., Perkins, D.O., Gu, H., Zipursky, R.B., Christensen, B.K., & Lieberman, J.A. (2006). A longitudinal study of neurocognitive function in individuals at-risk for psychosis. Schizophrenia Research, 88, 26–35. CrossRef Google Scholar
- Keshavan, M.S., Morris, D.W., Sweeney, J.A., Pearlson, G., Thaker, G., Seidman, L.J., & Tamminga, C. (2011). A dimensional approach to the psychosis spectrum between bipolar disorder and schizophrenia: The Schizo-Bipolar Scale. Schizophrenia Research, 133, 250–254. CrossRef Google Scholar PubMed
- Kraepelin, E. (1919). Dementia praecox and paraphrenia. Edinburgh, Scotland: E. & S. Livingstone. Google Scholar
- Kremen, W.S., Seidman, L.J., Faraone, S.V., Pepple, J.R., Lyons, M.J., & Tsuang, M.T. (1995). The “3 Rs” and neuropsychological function in schizophrenia: A test of the matching fallacy in biological relatives. Psychiatry Research, 56, 135–143. CrossRef Google Scholar
- Kremen, W.S., Seidman, L.J., Faraone, S.V., Toomey, R., & Tsuang, M.T. (2000). The paradox of normal neuropsychological function in schizophrenia. Journal of Abnormal Psychology, 109, 743–752. CrossRef Google Scholar
- Kremen, W.S., Seidman, L.J., Faraone, S.V., Toomey, R., & Tsuang, M.T. (2004). Heterogeneity of schizophrenia: A study of individual neuropsychological profiles. Schizophrenia Research, 71, 307–314. CrossRef Google Scholar PubMed
- Lewandowski, K.E., Cohen, B.M., & Ongur, D. (2011). Evolution of neuropsychological dysfunction during the course of schizophrenia and bipolar disorder. Psychological Medicine, 41, 225–241. CrossRef Google Scholar
- Maalouf, F.T., Klein, C., Clark, L., Sahakian, B.J., Labarbara, E.J., Versace, A., &Hassel, S., Almeida, J.R., & Phillips, M.L. (2010). Impaired sustained attention and executive dysfunction: Bipolar disorder versus depression-specific markers of affective disorders. Neuropsychologia, 48, 1862–1868. CrossRef Google Scholar PubMed
- Maxwell, M. (1996). Family Instrument for Genetic Studies (FIGS). NIMH: Clinical Neurogenetics Branch, Intramural Research Program. Google Scholar
- Mayer, W., Zobel, A., & Wagner, M. (2007). Schizophrenia and bipolar disorder: Differences and overlaps. Current Opinion in Psychiatry, 20, 417–432. Google Scholar
- Maziade, M., Rouleau, N., Gingras, N., Boutin, P., Paradis, M., Jomphe, V., & Roy, M. (2009). Shared neurocognitive dysfunctions in young offspring at extreme risk for schizophrenia or bipolar disorder in Eastern Quebec multigenerational families. Schizophrenia Bulletin, 35, 919–930. CrossRef Google Scholar PubMed
- McIntosh, A.M., Job, D.E., Moorhead, W.J., Harrison, L.K., Whalley, H.C., Johnstone, E.C., & Lawrie, S.M. (2006). Genetic liability to schizophrenia or bipolar disorder and its relationship to brain structure. American Journal of Medical Genetics B: Neuropsychiatric Genetics, 141, 76–83. CrossRef Google Scholar
- Meehl, P.E. (1970). Nuisance variables and the ex post facto design. In M. Radner & S. Winokur (Eds.), Minnesota studies in the philosophy of science (pp. 373–402). Minneapolis: University of Minnesota Press. Google Scholar
- Mesholam-Gately, R., Giuliano, A.J., Faraone, S.V., Goff, K.P., & Seidman, L.J. (2009). Neurocognition in first-episode schizophrenia: A meta-analytic review. Neuropsychology, 23, 315–336. CrossRef Google Scholar PubMed
- Michel, C., Ruhrmann, S., Schimmelmann, B.G., Klosterkotter, J., & Schulze-Lutter, F. (2014). A stratified model for psychosis prediction in clinical practice. Schizophrenia Bulletin, 40, 1533–1542. CrossRef Google Scholar PubMed
- Murrough, J.W., Iacoviello, B., Neumeister, A., Charney, D.S., & Iosifescu, D.V. (2011). Cognitive dysfunction in depression: Neurocircuitry and new therapeutic strategies. Neurobiology of Learning and Memory, 96, 553–563. CrossRef Google Scholar PubMed
- Murray, R.M., Sham, P., van Os, J., Zanelli, J., Cannon, M., & McDonald, C. (2004). A developmental model for similarities and dissimilarities between schizophrenia and bipolar disorder. Schizophrenia Research, 71, 405–416. CrossRef Google Scholar PubMed
- Nurnberger, J.I., Blehar, M.C., Kaufmann, C.A., York-Cooler, C., Simpson, S.G., Harkavy Friedman, J., & Kirch, D. (1994). Diagnostic interview for genetic studies (DIGS): Rationale, unique features, and training. Archives of General Psychiatry, 51, 849–859. CrossRef Google Scholar
- Park, S., Holzman, P.S., & Goldman-Rakic, P.S. (1995). Spatial working memory deficits in the relatives of schizophrenic patients. Archives of General Psychiatry, 52, 821–828. CrossRef Google Scholar PubMed
- Park, S., & Gooding, D.C. (2014). Working memory impairment as an endophenotypic marker of a schizophrenia diathesis. Schizophrenia Research: Cognition, 1, 127–136. CrossRef Google Scholar PubMed
- Phillips, L.K., & Seidman, L.J. (2008). Emotion processing in persons at risk for schizophrenia. Schizophrenia Bulletin, 34, 888–903. CrossRef Google Scholar
- Pousada-Casal, A. (2010). Study about problem solving and working memory in bipolar disorder patients in euthymia. Madrid: University Complutense of Madrid. Google Scholar
- Rasic, D., Hajek, T., Alda, A., & Uher, R. (2014). Risk of mental illness in offspring of parents with schizophrenia, bipolar disorder, and major depressive disorder: A meta-analysis of family high-risk studies. Schizophrenia Bulletin, 40, 28–38. CrossRef Google Scholar
- Reichenberg, A., Harvey, P.D., Bowie, C.R., Mojtabai, R., Rabinowitz, J., Heaton, R.K., & Bromet, E. (2009). Neuropsychological function and dysfunction in schizophrenia and psychotic affective disorders. Schizophrenia Bulletin, 35, 1022–1029. CrossRef Google Scholar PubMed
- Reichenberg, A., Caspi, A., Harrington, H., Houts, R., Keefe, R.S.E., Murray, R.M., & Moffitt, T.E. (2010). Static and dynamic cognitive deficits in childhood preceding adult schizophrenia: A 30-year study. American Journal of Psychiatry, 167, 160–169. CrossRef Google Scholar PubMed
- Riecher-Rossler, A., Pflueger, M.O., Aston, J., Borgwardt, S.J., Brewer, W.J., Gschwandtner, U., & Stieglitz, R.-D. (2009). Efficacy of using cognitive status in predicting psychosis: A 7-year follow-up. Biological Psychiatry, 66, 1023–1030. CrossRef Google Scholar PubMed
- Rosso, I.M., Makris, N., Thermenos, H.W., Hodge, S.M., Brown, A., Kennedy, D., & Seidman, L.J. (2010). Regional prefrontal cortex gray matter volumes in youth at familial risk for schizophrenia from the Harvard Adolescent High Risk Study. Schizophrenia Research, 123, 15–21. CrossRef Google Scholar PubMed
- Rosvold, H.E., Mirsky, A.F., Sarason, I., Bransome, E.D., & Beck, L.H. (1956). A continuous performance test of brain damage. Journal of Consulting Psychology, 20, 343–350. CrossRef Google Scholar
- Sánchez-Morla, E.M., Barabash, A., Martínez-Vizcaíno, V., Tabarés-Seisdedos, R., Balanzá-Martínez, V., Cabranes-Díaz, J.A., & Gómez, J.L. (2009). Comparative study of neurocognitive function in euthymic bipolar patients and stabilized schizophrenic patients. Psychiatry Research, 169, 220–228. CrossRef Google Scholar PubMed
- Scala, S., Pousada, A., Stone, W.S., Thermenos, H.W., Manschreck, T.C., Tsuang, M.T., & Seidman, L.J. (2013). Verbal and visual-spatial memory impairment in youth at familial high risk for schizophrenia or affective psychosis: A pilot study. Schizophrenia Research, 144, 122–128. CrossRef Google Scholar PubMed
- Schatzberg, A.F., Posener, J.A., DeBattista, C., Kalehzan, B.M., Rothschild, A.J., & Shear, P.K. (2000). Neuropsychological deficits in psychotic versus nonpsychotic major depression and no mental illness. American Journal of Psychiatry, 157, 1095–1100. CrossRef Google Scholar
- Schouws, S.N., Zoeteman, J.B., Comijs, H.C., Stek, M.L., & Beekman, A.T. (2007). Cognitive functioning in elderly patients with early onset bipolar disorder. International Journal of Geriatric Psychiatry, 22, 856–861. CrossRef Google Scholar PubMed
- Schubert, E.W., & McNeil, T.F. (2005). Neuropsychological impairment and its neurological correlates in adult offspring with heightened risk for schizophrenia and affective psychosis. American Journal of Psychiatry, 162, 758–766. CrossRef Google Scholar
- Schubert, E.W., & McNeil, T.F. (2007). Neurobehavioral deficits in young adult offspring with heightened risk for psychosis who developed schizophrenia-spectrum disorder. Schizophrenia Research, 94, 107–113. CrossRef Google Scholar PubMed
- Seidman, L.J., Breiter, H., Goodman, J.M., Goldstein, J.M., Woodruff, P., O’Craven, K., & Rosen, B.R. (1998). A functional magnetic resonance imaging study of auditory vigilance with low and high information processing demands. Neuropsychology, 12, 505–518. CrossRef Google Scholar PubMed
- Seidman, L.J., Kremen, W.S., Koren, D., Faraone, S.V., Goldstein, J.M., & Tsuang, M.T. (2002). A comparative profile analysis of neuropsychological functioning in patients with schizophrenia and bipolar psychoses. Schizophrenia Research, 53, 31–44. CrossRef Google Scholar PubMed
- Seidman, L.J., Giuliano, A.J., Smith, C.W., Stone, W.S., Glatt, S.J., Meyer, E., & Cornblatt, B. (2006). Neuropsychological functioning in adolescents and young adults at genetic risk for schizophrenia and affective psychoses: Results from the Harvard and Hillside adolescent high risk studies. Schizophrenia Bulletin, 32, 507–524. CrossRef Google Scholar
- Seidman, L.J., Thermenos, H.W., Koch, J.K., Ward, M., Breiter, H., Goldstein, J.M., & Tsuang, M.T. (2007). Auditory verbal working memory load and thalamic activation in non-psychotic relatives of persons with schizophrenia: An FMRI replication. Neuropsychology, 21, 599–610. CrossRef Google Scholar
- Seidman, L.J., Giuliano, A.J., Meyer, E.C., Addington, J., Cadenhead, K.S., Cannon, T.D., & Cornblatt, B. (2010). Neuropsychology of the prodrome to psychosis in the NAPLS consortium: Relationship to family history and conversion to psychosis. Archives General Psychiatry, 67, 578–588. CrossRef Google Scholar PubMed
- Seidman, L.J., Meyer, E., Giuliano, A.J., Breiter, H., Goldstein, J.M., Kremen, W.S., & Faraone, S.V. (2012). Auditory working memory impairments in individuals at familial high risk for schizophrenia. Neuropsychology, 26, 288–303. CrossRef Google Scholar PubMed
- Seidman, L.J., Cherkerzian, S., Goldstein, J.M., Agnew-Blais, J., Tsuang, M.T., & Buka, S.L. (2013). Neuropsychological performance and family history in children at age 7 who develop adult schizophrenia or bipolar psychosis in the New England High-Risk Family Study. Psychological Medicine, 43, 119–131. CrossRef Google Scholar PubMed
- Sitskoorn, M., Aleman, A., Ebisch, S., Appels, M., & Kahn, R. (2004). Cognitive deficits in relatives of patients with schizophrenia: A meta-analysis. Schizophrenia Research, 71, 285–295. CrossRef Google Scholar PubMed
- Snitz, B.E., Macdonald, A.W. III, & Carter, C.S. (2006). Cognitive deficits in unaffected first-degree relatives of schizophrenia patients: A meta-analytic review of putative endophenotypes. Schizophrenia Bulletin, 32, 179–194. CrossRef Google Scholar PubMed
- Sperry, S.H., O’Connor, L.K., Ongur, D., Cohen, B.M., Keshavan, M.S., & Lewandowski, K.E. (2015). Measuring cognition in bipolar disorder with psychosis using the MATRICS consensus cognitive battery. Journal of the International Neuropsychological Society, 21, 468–472. CrossRef Google Scholar PubMed
- Spring, B. (1985). Distractibility as a marker of vulnerability to schizophrenia. Psychopharmacology Bulletin, 21, 509–512. Google Scholar
- Thermenos, H.W., Seidman, L.J., Breiter, H., Goldstein, J.M., Goodman, J.M., Poldrack, R., & Tsuang, M.T. (2004). Functional MRI during auditory verbal working memory in non-psychotic relatives of persons with schizophrenia: A pilot study. Biological Psychiatry, 55, 490–500. CrossRef Google Scholar
- Tohen, M., Hennen, J., Zarate, C.M. Jr., Baldessarini, R.J., Strakowski, S.M., Stoll, A.L., & Cohen, B.M. (2000). Two-year syndromal and functional recovery in 219 cases of first-episode major affective disorder with psychotic features. American Journal of Psychiatry, 157, 220–228. CrossRef Google Scholar PubMed
- Trandafir, A., Meary, A., Schurhoff, F., Leboyer, M., & Szoke, A. (2006). Memory tests in first-degree adult relatives of schizophrenic patients: A meta-analysis. Schizophrenia Research, 81, 217–226. CrossRef Google Scholar PubMed
- Van Gorp, W.G., Altshuler, L., Theberge, D.C., Wilkins, J., & Dixon, W. (1988). Cognitive impairment in euthymic bipolar patients with and without prior alcohol dependence: A preliminary study. Archives of General Psychiatry, 55, 41–46. CrossRef Google Scholar PubMed
- Wechsler, D. (1991). Manual for the Wechsler Intelligence Scale for Children(3rd ed.), San Antonio, TX: The Psychological Corporation. Google Scholar
- Wechsler, D. (1997). Wechsler Adult Intelligence Scale ((3rd ed.), San Antonio, TX: The Psychological Corporation. Google Scholar
- Wilkinson, G. (1993). Wide Range Achievement Test, Administration Manual ((3rd ed.), Wilmington, DE: Wide Range, Inc. Google Scholar
- Woodberry, K., Giuliano, A.J., & Seidman, L.J. (2008). Premorbid IQ in schizophrenia: A meta-analytic review. American Journal of Psychiatry, 165, 579–587. CrossRef Google Scholar PubMed
- Zhang, R., Picchioni, M., Allen, A., & Toulopoulou, T. (2016). Working memory in unaffected relatives of patients with schizophrenia: A meta-analysis of functional magnetic resonance imaging studies. Schizophrenia Bulletin doi:10.1093/schbul/sbv221 [Epub ahead of print]. CrossRef Google Scholar PubMed
Intraindividual cognitive variability (IICV) has been shown to differentiate between groups with normal cognition, mild cognitive impairment (MCI), and dementia. This study examined whether baseline IICV predicted subsequent mild to moderate cognitive impairment in a cognitively normal baseline sample.
Participants with 4 waves of cognitive assessment were drawn from the Wisconsin Registry for Alzheimer’s Prevention (WRAP;n=684; 53.6(6.6) baseline age; 9.1(1.0) years follow-up; 70% female; 74.6% parental history of Alzheimer’s disease). The primary outcome was Wave 4 cognitive status (“cognitively normal”vs. “impaired”) determined by consensus conference; “impaired” included early MCI (n=109), clinical MCI (n=11), or dementia (n=1). Primary predictors included two IICV variables, each based on the standard deviation of a set of scores: “6 Factor IICV” and “4 Test IICV”. Each IICV variable was tested in a series of logistic regression models to determine whether IICV predicted cognitive status. In exploratory analyses, distribution-based cutoffs incorporating memory, executive function, and IICV patterns were used to create and test an MCI risk variable.
Results were similar for the IICV variables: higher IICV was associated with greater risk of subsequent impairment after covariate adjustment. After adjusting for memory and executive functioning scores contributing to IICV, IICV was not significant. The MCI risk variable also predicted risk of impairment.
While IICV in middle-age predicts subsequent impairment, it is a weaker risk indicator than the memory and executive function scores contributing to its calculation. Exploratory analyses suggest potential to incorporate IICV patterns into risk assessment in clinical settings. (JINS, 2016,22, 1016–1025)
- Aisen, P.S., Petersen, R.C., Donohue, M.C., Gamst, A., Raman, R., Thomas, R.G., & Weiner, M.W. (2010). Clinical core of the Alzheimer’s disease neuroimaging initiative: Progress and plans. Alzheimer’s & Dementia, 6(3), 239–246. http://doi.org/10.1016/j.jalz.2010.03.006. CrossRef Google Scholar
- Albert, M.S., DeKosky, S.T., Dickson, D., Dubois, B., Feldman, H.H., Fox, N.C., & Petersen, R.C. (2011). The diagnosis of mild cognitive impairment due to Alzheimer’s disease: Recommendations from the National Institute on Aging-Alzheimer’s Association workgroups on diagnostic guidelines for Alzheimer’s disease. Alzheimer’s & Dementia, 7(3), 270–279. CrossRef Google Scholar
- Andersson, C., Lindau, M., Almkvist, O., Engfeldt, P., Johansson, S.-E., & Eriksdotter Jönhagen, M. (2006). Identifying patients at high and low risk of cognitive decline using Rey Auditory Verbal Learning Test among middle-aged memory clinic outpatients. Dementia and Geriatric Cognitive Disorders, 21(4), 251–259. CrossRef Google Scholar PubMed
- Bondi, M.W., Edmonds, E.C., Jak, A.J., Clark, L.R., Delano-Wood, L., McDonald, C.R., & Galasko, D. (2014). Neuropsychological criteria for mild cognitive impairment improves diagnostic precision, biomarker associations, and progression rates. Journal of Alzheimer’s Disease, 42(1), 275–289. Google Scholar PubMed
- Clark, L.R., Koscik, R.L., Nicholas, C.R., Okonkwo, O.C., Engelman, C.D., Bratzke, L.C., & Johnson, S.C. (2016). Mild cognitive impairment in late middle age in the Wisconsin Registry for Alzheimer’s Prevention (WRAP) study: Prevalence and characteristics using robust and standard neuropsychological normative data. [Epub ahead of print]. Google Scholar
- Cohen, J. (1992). A power primer. Psychological Bulletin, 112(1), 155. CrossRef Google Scholar PubMed
- Dowling, N.M., Hermann, B., La Rue, A., & Sager, M.A. (2010). Latent structure and factorial invariance of a neuropsychological test battery for the study of preclinical Alzheimer’s disease. Neuropsychology, 24(6), 742–756. http://doi.org/10.1037/a0020176. CrossRef Google Scholar PubMed
- Drebing, C.E., Van Gorp, W.G., Stuck, A.E., Mitrushina, M., & Beck, J. (1994). Early detection of cognitive decline in higher cognitively functioning older adults: Sensitivity and specificity of a neuropsychological screening battery. Neuropsychology, 8(1), 31. CrossRef Google Scholar
- Duara, R., Loewenstein, D.A., Greig, M.T., Potter, E., Barker, W., Raj, A., & Potter, H. (2011). Pre-MCI and MCI: Neuropsychological, clinical, and imaging features and progression rates. The American Journal of Geriatric Psychiatry, 19(11), 951–960. http://doi.org/10.1097/JGP.0b013e3182107c69 CrossRef Google Scholar
- Grice, J.W. (2001). Computing and evaluating factor scores. Psychological Methods, 6(4), 430. CrossRef Google Scholar PubMed
- Heaton, R., Miller, S., Taylor, J.R., & Grant, I. (2004). Comprehensive norms for an expanded Halstead-Reitan battery: Demographically adjusted neuropsychological norms for African American and Caucasian adults (HRB). Professional Manual. Lutz, FL: Psychological Assessment Resources Inc.. Google Scholar
- Hilborn, J.V., Strauss, E., Hultsch, D.F., & Hunter, M.A. (2009). Intraindividual variability across cognitive domains: Investigation of dispersion levels and performance profiles in older adults. Journal of Clinical and Experimental Neuropsychology, 31(4), 412–424. CrossRef Google Scholar PubMed
- Holtzer, R., Verghese, J., Wang, C., Hall, CB., & Lipton, RB. (2008). WIthin-person across-neuropsychological test variability and incident dementia. JAMA, 300(7), 823–830. http://doi.org/10.1001/jama.300.7.823. CrossRef Google Scholar PubMed
- Imtiaz, B., Tolppanen, A.-M., Kivipelto, M., & Soininen, H. (2014). Future directions in Alzheimer’s disease from risk factors to prevention. Biochemical Pharmacology, 88(4), 661–670. CrossRef Google Scholar PubMed
- Jessen, F., Wolfsgruber, S., Wiese, B., Bickel, H., Mösch, E., Kaduszkiewicz, H., & Wagner, M. (2014). AD dementia risk in late MCI, in early MCI, and in subjective memory impairment. Alzheimer’s & Dementia, 10(1), 76–83. http://doi.org/10.1016/j.jalz.2012.09.017. CrossRef Google Scholar PubMed
- Kälin, A.M., Pflüger, M., Gietl, A.F., Riese, F., Jäncke, L., Nitsch, R.M., && Hock, C. (2014). Intraindividual variability across cognitive tasks as a potential marker for prodromal Alzheimer’s disease. Frontiers in Aging Neuroscience, 6, 147. CrossRef Google Scholar PubMed
- Koepsell, T.D., & Monsell, S.E. (2012). Reversion from mild cognitive impairment to normal or near-normal cognition Risk factors and prognosis. Neurology, 79(15), 1591–1598. CrossRef Google Scholar PubMed
- Koscik, R.L., La Rue, A., Jonaitis, E.M., Okonkwo, O.C., Johnson, S.C., Bendlin, B.B., & Sager, M.A. (2014). Emergence of mild cognitive impairment in late middle-aged adults in the Wisconsin registry for Alzheimer’s prevention. Dementia and Geriatric Cognitive Disorders, 38(1–2), 16–30. http://doi.org/10.1159/000355682. CrossRef Google Scholar PubMed
- Lewis, F., Butler, A., & Gilbert, L. (2011). A unified approach to model selection using the likelihood ratio test. Methods in Ecology and Evolution, 2(2), 155–162. http://doi.org/10.1111/j.2041-210X.2010.00063.x CrossRef Google Scholar
- MacDonald, S.W., Hultsch, D.F., & Dixon, R.A. (2003). Performance variability is related to change in cognition: Evidence from the Victoria Longitudinal Study. Psychology and Aging, 18(3), 510. CrossRef Google Scholar PubMed
- Petersen, R.C., Aisen, P.S., Beckett, L.A., Donohue, M.C., Gamst, A.C., Harvey, D.J., & Toga, A.W. (2010). Alzheimer’s disease Neuroimaging Initiative (ADNI) clinical characterization. Neurology, 74(3), 201–209. CrossRef Google Scholar
- Sager, M.A., Hermann, B., & La Rue, A. (2005). Middle-aged children of persons with Alzheimer’s disease: APOE genotypes and cognitive function in the Wisconsin Registry for Alzheimer’s Prevention. Journal of Geriatric Psychiatry and Neurology, 18(4), 245–249. http://doi.org/10.1177/0891988705281882 CrossRef Google Scholar PubMed
- Salthouse, T.A., & Soubelet, A. (2014). Heterogeneous ability profiles may be a unique indicator of impending cognitive decline. Neuropsychology, 28(5), 812. CrossRef Google Scholar PubMed
- Schmidt, M. (1996). Rey auditory verbal learning test: A handbook. Los Angeles: Western Psychological Services. Google Scholar
- Sperling, R.A., Karlawish, J., & Johnson, K.A. (2013). Preclinical Alzheimer disease —the challenges ahead. Nature Reviews. Neurology, 9(1), 54–58. http://doi.org/10.1038/nrneurol.2012.241 CrossRef Google Scholar PubMed
- Vaughan, L., Leng, I., Dagenbach, D., Resnick, S.M., Rapp, S.R., Jennings, J.M., & Coker, L.H. (2013). Intraindividual variability in domain-specific cognition and risk of mild cognitive impairment and dementia. Current Gerontology and Geriatrics Research, 2013, 495793. CrossRef Google Scholar PubMed
- Wechsler, D. (1987). WMS-R: Wechsler memory scale-revised. San Antonio, TX: Psychological Corporation. Google Scholar
- Wilkinson, G.S. (1993). WRAT-3: Wide range achievement test. San Antonio, TX: Pearson. Google Scholar
The aim of this study was to demonstrate the utility of an evidence-based assessment (EBA) model to establish a multimodal set of tools for identifying students at risk for perceived post-injury academic problems.
Participants included 142 students diagnosed with concussion (age:M=14.95;SD=1.80; 59% male), evaluated within 4 weeks of injury (median=16 days). Demographics, pre-injury history, self- and parent-report measures assessing symptom severity and executive functions, and cognitive test performance were examined as predictors of self-reported post-injury academic problems.
Latent class analysis categorized participants into “high” (44%) and “low” (56%) levels of self-reported academic problems. Receiver operating characteristic analyses revealed significant discriminative validity for self- and parent-reported symptom severity and executive dysfunction and self-reported exertional response for identifying students reporting lowversushigh academic problems. Parent-reported symptom ratings [area under the receiver operating characteristic curve (AUC)=.79] and executive dysfunction (AUC=.74), and self-reported ratings of executive dysfunction (AUC=.84), symptoms (AUC=.80), and exertional response (AUC=.70) each classified students significantly better than chance (ps<.001). Hierarchical logistic regression indicated that, of the above, self-reported symptoms and executive dysfunction accounted for the most variance in the prediction of self-reported academic problems.
Post-concussion symptom severity and executive dysfunction significantly predict perceived post-injury academic problems. EBA modeling identified the strongest set of predictors of academic challenges, offering an important perspective in the management of concussion by applying traditional strengths of neuropsychological assessment to clinical decision making. (JINS, 2016,22, 1038–1049)
- Bossuyt, P.M., Reitsma, J.B., Bruns, D.E., Gatsonis, C.A., Glasziou, P.P., Irwig, L.M., & de Vet, H.C.W. (2003). Towards complete and accurate reporting of studies of diagnostic accuracy: The STARD initiative. British Medical Journal, 326, 41–44. CrossRef Google Scholar PubMed
- Brooks, B.L., Kadoura, B., Turley, B., Crawford, S., Mikrogianakis, A., & Barlow, K.M. (2014). Perception of recovery after pediatric mild traumatic brain injury is influenced by the “good old days” bias: Tangible implications for clinical practice and outcomes research. Archives of Clinical Neuropsychology, 29(2), 186–193. http://doi.org/10.1093/arclin/act083 CrossRef Google Scholar
- Center for Disease Control and Prevention, & National Center for Injury Prevention and Control. (2015, February 26). What are the signs and symptoms of concussion? Retrieved from http://www.cdc.gov/concussion/signs_symptoms.html Google Scholar
- Chelune, G.J. (2010). Evidence-based research and practice in clinical neuropsychology. The Clinical Neuropsychologist, 24(3), 454–467. http://doi.org/10.1080/13854040802360574 CrossRef Google Scholar
- Echemendia, R.J., Iverson, G.L., McCrea, M., Macciocchi, S.N., Gioia, G.A., Putukian, M., & Comper, P. (2013). Advances in neuropsychological assessment of sport-related concussion. British Journal of Sports Medicine, 47(5), 294–298. http://doi.org/10.1136/bjsports-2013-092186 CrossRef Google Scholar PubMed
- Findling, R.L., Jo, B., Frazier, T.W., Youngstrom, E.A., Demeter, C.A., Fristad, M.A., & Horwitz, S.M. (2013). The 24-month course of manic symptoms in children. Bipolar Disorders, 15(6), 669–679. http://doi.org/10.1111/bdi.12100 CrossRef Google Scholar PubMed
- Gioia, G.A. (2014). Medical-school partnership in guiding return to school following mild traumatic brain injury in youth. Journal of Child Neurology, 883073814555604. http://doi.org/10.1177/0883073814555604 Google Scholar
- Gioia, G.A. (2015). Multimodal evaluation and management of children with concussion: Using our heads and available evidence. Brain Injury, 29(2), 195–206. http://doi.org/10.3109/02699052.2014.965210 CrossRef Google Scholar
- Gioia, G.A., Isquith, P.K., Guy, S.C., & Kenworthy, L. (2000). TEST REVIEW behavior rating inventory of executive function. Child Neuropsychology, 6(3), 235–238. http://doi.org/10.1076/chin.6.3.235.3152 CrossRef Google Scholar
- Evidence-Based Medicine Working Group. (1992). Evidence-based medicine: A new approach to teaching the practice of medicine. JAMA, 268(17), 2420–2425. http://doi.org/10.1001/jama.1992.03490170092032 CrossRef Google Scholar
- Hagenaars, J.A., & McCutcheon, A.L. (2002). Applied latent class analysis. New York: Cambridge University Press. CrossRef Google Scholar
- Halstead, M.E., McAvoy, K., Devore, C.D., Carl, R., Lee, M., & Logan, K. (2013). Returning to learning following a concussion. Pediatrics, 948–957. http://doi.org/10.1542/peds.2013–2867 CrossRef Google Scholar PubMed
- Hunsley, J., & Mash, E.J. (2007). Evidence-based assessment. Annual Review of Clinical Psychology, 3(1), 29–51. http://doi.org/10.1146/annurev.clinpsy.3.022806.091419 CrossRef Google Scholar PubMed
- Iverson, G.L., Lange, R.T., Brooks, B.L., & Rennison, V.L.A. (2010). “Good old days” bias following mild traumatic brain injury. The Clinical Neuropsychologist, 24(1), 17–37. http://doi.org/10.1080/13854040903190797 CrossRef Google Scholar PubMed
- Kraemer, H.C. (1992). Evaluating medical tests: Objective and quantitative guidelines. Newbury Park, CA: Sage. Google Scholar
- Kraemer, H.C., Kazdin, A.E., Offord, D.R., Kessler, R.C., Jensen, P.S., & Kupfer, D.J. (1999). Measuring the potency of risk factors for clinical or policy significance. Psychological Methods, 4(3), 257–271. http://doi.org/10.1037/1082-989X.4.3.257 CrossRef Google Scholar
- Lovell, M.R., Collins, M., Podell, K., Powell, J., & Maroon, J. (2000). ImPACT: Immediate post-concussion assessment and cognitive testing. Pittsburgh, PA: Neurohealth Systems, LLC. Google Scholar
- McCrory, P., Meeuwisse, W.H., Aubry, M., Cantu, B., Dvořák, J., Echemendia, R.J., & Turner, M. (2013). Consensus statement on concussion in sport: The 4th International Conference on Concussion in Sport held in Zurich, November 2012. British Journal of Sports Medicine, 47(5), 250–258. http://doi.org/10.1136/bjsports-2013-092313 CrossRef Google Scholar PubMed
- McCutcheon, A. (1987). Latent class analysis. Thousand Oaks, CA: Sage. CrossRef Google Scholar PubMed
- McGill, C., Gerst, E., Isquith, P., & Gioia, G. (2011). Evidence of validity for a monitoring version of the Behavior Rating Inventory of Executive Function (BRIEF). Journal of the International Neuropsychological Society, 17(s1), 297. Google Scholar
- Mittenberg, W., DiGiulio, D.V., Perrin, S., & Bass, A.E. (1992). Symptoms following mild head injury: Expectation as aetiology. Journal of Neurology, Neurosurgery, and Psychiatry, 55(3), 200–204. CrossRef Google Scholar PubMed
- Newman, J.B., Reesman, J.H., Vaughan, C.G., & Gioia, G.A. (2013). Assessment of processing speed in children with mild TBI: A “first look” at the validity of pediatric ImPACT. The Clinical Neuropsychologist, 27(5), 779–793. http://doi.org/10.1080/13854046.2013.789552 CrossRef Google Scholar PubMed
- Pepe, M.S. (2004). The statistical evaluation of medical tests for classification and prediction. New York, NY: Oxford University Press. Google Scholar
- R Core Team. (2013). R: A language and environment for statistical computing. Vienna, Austria: R Foundation for Statistical Computing. Retrieved from, http://www.R-project.org/Google Scholar PubMed
- Ransom, D.M., Vaughan, C.G., Pratson, L., Sady, M.D., McGill, C.A., & Gioia, G.A. (2015). Academic effects of concussion in children and adolescents. Pediatrics, 135, 1043–1050. http://doi.org/10.1542/peds.2014-3434 CrossRef Google Scholar
- Rice, M.E., & Harris, G.T. (2005). Comparing effect sizes in follow-up studies: ROC Area, Cohen’s d, and r. Law and Human Behavior, 29(5), 615–620. http://doi.org/10.1007/s10979-005-6832-7 CrossRef Google Scholar PubMed
- Sady, M.D., McGill, C.A., Gerst, E.H., & Gioia, G.A. (2013). Standardized assessment of cognitive exertion in mTBI and non-injured children. Journal of the International Neuropsychological Society, 19(S1), 194. http://doi.org/10.1017/S1355617713000362 Google Scholar
- Sady, M.D., Vaughan, C.G., & Gioia, G.A. (2011). School and the concussed youth: Recommendations for concussion education and management. Physical Medicine and Rehabilitation Clinics of North America, 22(4), 701–719, ix. http://doi.org/10.1016/j.pmr.2011.08.008 CrossRef Google Scholar PubMed
- Sady, M.D., Vaughan, C.G., & Gioia, G.A. (2014). Psychometric characteristics of the postconcussion symptom inventory in children and adolescents. Archives of Clinical Neuropsychology, 29(4), 348–363. http://doi.org/10.1093/arclin/acu014 CrossRef Google Scholar PubMed
- Schatz, P., & Maerlender, A. (2013). A two-factor theory for concussion assessment using ImPACT: Memory and speed. Archives of Clinical Neuropsychology, 28(8), 791–797. http://doi.org/10.1093/arclin/act077 CrossRef Google Scholar
- Straus, S.E., Glasziou, P., Richardson, W.S., & Haynes, R.B. (2011). Evidence-based medicine: How to practice and teach it (4th ed.), Edinburgh: Churchill Livingstone. Google Scholar
- Venkatraman, E.S., & Begg, C.B. (1996). A distribution-free procedure for comparing receiver operating characteristic curves from a paired experiment. Biometrika, 83(4), 835–848. http://doi.org/10.1093/biomet/83.4.835 CrossRef Google Scholar
- Vermunt, J., & Magidson, J. (2005). Latent GOLD Choice 4.0 User’s Guide. Belmont, MA: Statistical Innovations Inc. Google Scholar
- Youngstrom, E.A. (2013). Future directions in psychological assessment: Combining evidence-based medicine innovations with psychology’s historical strengths to enhance utility. Journal of Clinical Child and Adolescent Psychology, 42(1), 139–159. http://doi.org/10.1080/15374416.2012.736358 CrossRef Google Scholar
- Youngstrom, E.A. (2014). A primer on receiver operating characteristic analysis and diagnostic efficiency statistics for pediatric psychology: We are ready to ROC. Journal of Pediatric Psychology, 39, 204–221. http://doi.org/10.1093/jpepsy/jst062 CrossRef Google Scholar PubMed
- Youngstrom, E.A., Meyers, O., Youngstrom, J.K., Calabrese, J.R., & Findling, R.L. (2006). Diagnostic and measurement issues in the assessment of pediatric bipolar disorder: implications for understanding mood disorder across the life cycle. Developmental Psychopathology, 18(4), 989–1021. CrossRef Google Scholar PubMed
- Zhou, X.-H., Obuchowski, N., & McClish, D. (2002). Statistical methods in diagnostic medicine. New York: Wiley. Retrieved from http://onlinelibrary.wiley.com/doi/10.1111/1541-0420.00266/abstract CrossRef Google Scholar