2 CE Credits. Special Issue on Prediction (JINS 22:10, 2016): CE Bundle 3
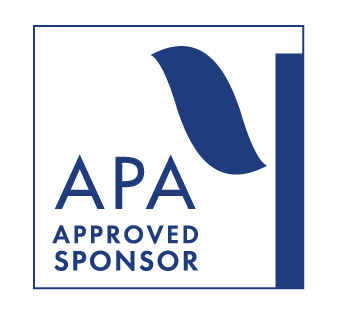
- Be able to describe cognitive, academic, and psychosocial outcomes after an incident demyelinating event in childhood.
- Describe the role of white matter integrity in the maintenance of episodic memory.
- List biomarkers associated with prodromal Parkinson’s Disease.
Target Audience: | Intermediate |
---|---|
Availability: | Date Available: 2016-11-30 |
You may obtain CE for this JINS package at any time. | |
Offered for CE | Yes |
Cost | Members $20 |
Non-Members $30 | |
Refund Policy | This JINS package is not eligible for refunds |
CE Credits | 2.0 |
Prediction of behavior and related outcomes has long been a principal goal of psychology. The development of intelligence tests in the early 20th century led to new strategies for predicting academic and occupational performance (Sternberg, 1996). Similarly, in the mid-19th century, Fechner’s use of mathematical models for prediction of behavior (Wertheimer, 1987) initiated a long tradition that has continued to the present day, which can be seen in the mathematical models of learning developed by Clark Hull (Hull, 1943), dynamic models of the development of spatial memory abilities (Spencer, Smith, & Thelen, 2001), and others. These ideas also led to information processing theories of memory (Atkinson & Shiffrin, 1968; Raaijmakers & Shiffrin, 1980), which have been used to predict specific stages of memory processing that are impaired in patient groups (Brown et al., 1995; Brown, Woodard, & Rich, 1994). Recently, use of prediction strategies in neuropsychology has turned toward development of new approaches for identification and characterization of the development and disease course of neurocognitive and neuropsychiatric disorders.
Prediction involves making a statement about an event that is uncertain, typically based on some type of known information. Although the term “prediction” brings to mind the forecasting of some future outcome or event, the term prediction can also be used for concurrent diagnostic purposes. Since approximately 2000, the number of research studies focused on prediction of diagnosis and clinical trajectories has increased dramatically. Figure 1 shows the number of publications per year returned from a simple search of the terms “preclinical prediction” in the National Library of Medicine (PubMed) database from 1950 to October 1, 2016. Studies of preclinical prediction in neuropsychology have typically focused on identification of persons at the highest risk for specific cognitive conditions. Early identification of risk, or of subtle signs of incipient disease, opens the possibility for treatments to prevent disease development, or to delay onset or slow progression of clinically significant symptoms. Prediction studies after brain injury have also been important for prognosticating the trajectory of recovery and for planning resource allocation for treatment.
State-of-the-art prediction strategies have been facilitated by at least two significant developments over the past 25 years. First, the availability of high-speed computers and software capable of performing complex statistical analyses has supported the development and validation of complex theoretical predictive models. For instance, machine learning approaches to prediction would not be possible without powerful computing resources (Hey, 2010). Machine learning has been successfully used in the context of diagnosis (Bigler, 2013; Mundt, Freed, & Greist, 2000; Teipel, Meindl, Grinberg, Heinsen, & Hampel, 2008) and prognosis (Gutman et al., 2015; Koutsouleris et al., 2009; Moradi, Pepe, Gaser, Huttunen, & Tohka, 2015; Schmidt-Richberg et al., 2016). Second, the identification of biomarkers of a variety of neurological and psychiatric conditions has provided a set of predictors that are highly sensitive to risk factors and pathological changes leading to these conditions (Chong, Lim, & Sahadevan, 2006; Craig-Schapiro, Fagan, & Holtzman, 2009; Mayeux, 2004; Sharma & Laskowitz, 2012; Shaw, Korecka, Clark, Lee, & Trojanowski, 2007). Such biomarkers can be used to test predictions regarding possible etiologies associated with neuropsychological abnormalities (Ivanoiu et al., 2015; Miller et al., 2008; Wirth et al., 2013). Improved genetic testing has also contributed to more accurate predictions of functional changes when combined with neuropsychological and imaging data (O’Hara et al., 1998; Reiman et al., 2004; Small et al., 1996). Some researchers have argued, based on the literature, that “neuromarkers often provide better predictions (neuroprognosis), alone or in combination with other measures, than traditional behavioral measures” (page 11, Gabrieli, Ghosh, & Whitfield-Gabrieli, 2015). As data increasingly emerge, our field will be enriched by identifying the best combinations of measures to predict disease.
Many neurocognitive disorders are known to evolve from a presymptomatic to mildly clinical state to a fully clinical disorder. In essence, they go through different biological and clinical “stages” (McGorry et al., 2007). Two disorders receiving a great deal of research over the past two decades are Alzheimer’s disease (AD) and schizophrenia. For example, AD is preceded by mild cognitive impairment (MCI), and in many cases schizophrenia is preceded by a clinical high-risk (CHR) state identified by attenuated positive psychotic symptoms (i.e., mild delusions and hallucinations with some degree of intact reality testing; Tsuang et al., 2013; Yung & McGorry, 1996). At least in principle, identifying predictors and mechanisms of transition to AD or to psychosis among individuals showing signs of incipient neurocognitive disorders are critical steps in the search for preventive or early intervention strategies (Woodberry, Shapiro, Bryant, & Seidman, 2016). Interest in early detection and prevention of schizophrenia and other psychotic disorders has led to more than a decade of work studying young people who may be at risk of developing a psychotic illness, and advances have been made in prediction of transition to psychosis from a CHR stage (Cannon et al., 2008, 2016; Carrion et al., 2016), including the usage of neuropsychological measures (Giuliano et al., 2012; Seidman, Giuliano, & Walker, 2010; Seidman et al., 2016).
Studies investigating early detection of neurological and psychiatric conditions have improved understanding of etiology and diagnosis significantly, and they have opened new avenues for management. Presymptomatic detection is also essential to the development of effective intervention strategies, as it provides a window for preventing/delaying onset or reducing severity. This special issue of the Journal of the International Neuropsychological Society includes nine papers describing cutting-edge empirical findings that exemplify key methodological advances for preclinical detection of a variety of neurological, neurodevelopmental, and neuropsychiatric conditions. Methodological approaches taken include assessment of familial and genetic risk analyses, phenotypic characterization using cognitive and/or imaging methods, and evaluation of biomarker effectiveness. These papers provide substantive integrative and synthetic summaries of the current status of preclinical detection methodologies and future directions for the field.
As novel biomarkers of early, disease-related changes are identified, strategies for making optimal use of this information are becoming increasingly important. In this special issue, several papers focus on description of novel methodologies for combining biomarker data with other clinical information for diagnosis or prognosis. In a thorough literature review, Cooper and colleagues describe state-of-the-art objective biomarkers in prodromal Parkinson’s disease (PD), and they discuss several strategies for combining these biomarkers with clinical and genetic data for improving sensitivity and specificity for identification of persons with prodromal PD. Soldan and colleagues demonstrate that beta-amyloid and phosphorylated tau measured in cerebrospinal fluid can predict cognitive functioning as long as 10 years later. Using data from the Alzheimer’s Disease Neuroimaging Initiative, Edmonds and colleagues demonstrate how a novel method for staging preclinical AD using amyloid positron emission tomography (PET) imaging can be combined with detailed cognitive assessment to better characterize preclinical AD. Notably, this study found that considerable amyloid accumulation had already occurred before clinical diagnosis. Finally, in a cross-sectional study, Quenon and colleagues demonstrate the relationships between imaging measures of extent of early AD neuropathology, as indexed by in vivo neuroimaging biomarkers (amyloid PET, hippocampal volume, and measures of cortical thickness) and level of memory performance on the Free and Cued Selective Reminding Test. Although biomarkers of early AD neuropathology predicted overall memory performance, cueing efficiency, which is frequently impaired in AD, demonstrated particularly strong relationships with cortical thickness of regions that are commonly atrophic in early AD.
Assessment of the influence of familial and genetic risk factors has also become an important tool for forecasting diagnostic status. The family high-risk approach allows a defined selection process for ascertaining non-ill subjects in a family in which there is an identified proband with the illness. An advantage of such an approach is that it is not dependent on symptom expression, but rather genetic risk, and thus an unaffected individual could be studied at any age, enabling developmentally guided probes of risk (Agnew-Blais & Seidman, 2013). The “unaffected relatives” are typically offspring or siblings who are considered to be at higher risk for the illness or for phenotypes associated with the illness, because they carry approximately 50% of the genes for the illness. This approach has been used for over half a century, and has been one of the most fruitful ways of identifying components of the vulnerability to various illnesses, particularly schizophrenia. The most typical outcome used in many of these studies originally was “developing the illness” (e.g., schizophrenia, AD, etc.). However, outcomes can also be expressed in a range of phenotypes reflecting the underlying disorder, and outcomes such as functional disability are also very important. A wide range of phenotypes (e.g., working memory or attention problems, smaller hippocampi) can be studied at different ages to evaluate developmental effects, and in different sub-populations (e.g., those with higher vs. lower genetic loading) to study the specific subgroup expression of the phenotypes.
In this issue, Lancaster and colleagues demonstrate that baseline diffusion tensor imaging of the white matter microstructure in the medial temporal lobe can predict longitudinal changes in episodic memory functioning over 3 years in a sample of cognitively healthy older adults with an enriched familial and genetic risk for AD. Koscik and colleagues compare sensitivity for predicting subsequent cognitive impairment using either variability in performance across cognitive tasks or combinations of outcomes from particular tasks (e.g., memory and executive tasks) taken at baseline several years earlier. In an investigation of neuropsychological endophenotypes of familial risk for schizophrenia and affective psychosis, Seidman and colleagues found that working memory impairment was more robust than vigilance for characterizing the cognitive impairment associated with familial risk for schizophrenia. Although persons with familial risk for affective psychosis showed more impaired vigilance relative to other groups, this effect was eliminated after adjustment for several psychopathological symptoms. This work was part of an agenda to identify the most sensitive and specific neuropsychological predictors of risk for different forms of psychosis (see also Seidman et al., 2016). Each of these studies demonstrates novel methodologies for studying the influence of familial and genetic risk for possible diagnosis and prognosis.
Finally, two articles in this issue focus on the use of prediction strategies for prognosticating outcome after brain injury has already occurred in pediatric samples. Ransom and colleagues use evidence-based assessment (EBA) to identify teenage students who are at-risk for post-concussive academic difficulty. Self-reported post-injury symptoms and executive functioning difficulty, rather than parent-reported sequelae, showed the strongest relationships with perceived post-injury academic difficulties. This study demonstrates the utility of the EBA framework within the context of neuropsychological assessment. Till and colleagues studied cognitive, academic, and psychosocial difficulties experienced by children diagnosed with an acquired demyelinating syndrome (ADS), one-third of whom were later diagnosed with multiple sclerosis (MS), over a 6-month follow-up period. Children with ADS were shown to demonstrate a favorable neurocognitive outcome in the short-term, including children diagnosed with MS.
In summary, the papers in this Special Issue present several novel approaches toward developing methodologies for prediction in neuropsychology. Research on optimizing the information obtained from biomarkers will undoubtedly continue to be stimulated by the identification of new biomarkers in the future. Introduction of new frameworks for assessment, such as EBA, and other strategies for evaluating longitudinal cognitive, clinical, and neuroimaging changes in outcome, as presented by several studies in this special issue, will also be helpful for moving the field forward. Capitalization on new developments in genetic analyses and assessment of familial risk factors will also be important tools for improving predictive accuracy.
Nevertheless, we also face challenges with respect to definition of appropriate statistical models used for assessment of change, growth, or decline (Cronbach & Furby, 1970; Francis, Fletcher, Stuebing, Davidson, & Thompson, 1991; Gottman & Rushe, 1993; Harrell, 2015; Singer & Willett, 2003; Steyerberg, 2009; Steyerberg & Harrell, 2016; Steyerberg et al., 2010; Temkin, Heaton, Grant, & Dikmen, 1999). While these issues are certainly not new, continued focus on improving definitions of the change that we are predicting, and on models for assessing the effectiveness of variables predicting this change, are certainly warranted. Despite these challenges, research on preclinical prediction continues to grow, and future studies promise to contribute to improvement in preventative treatments before cognitive decline occurs as well as to more effective treatments and allocation of resources following brain injury.
White matter (WM) integrity within the mesial temporal lobe (MTL) is important for episodic memory (EM) functioning. The current study investigated the ability of diffusion tensor imaging (DTI) in MTL WM tracts to predict 3-year changes in EM performance in healthy elders at disproportionately higher genetic risk for Alzheimer’s disease (AD).
Fifty-one cognitively intact elders (52% with family history (FH) of dementia and 33% possessing an Apolipoprotein E ε4 allelle) were administered the Rey Auditory Verbal Learning Test (RAVLT) at study entry and at 3-year follow-up. DTI scanning, conducted at study entry, examined fractional anisotropy and mean, radial and axial diffusion within three MTL WM tracts: uncinate fasciculus (UNC), cingulate-hippocampal (CHG), and fornix-stria terminalis (FxS). Correlations were performed between residualized change scores computed from RAVLT trials 1–5, immediate recall, and delayed recall scores and baseline DTI measures; MTL gray matter (GM) and WM volumes; demographics; and AD genetic and metabolic risk factors.
Higher MTL mean and axial diffusivity at baseline significantly predicted 3-year changes in EM, whereas baseline MTL GM and WM volumes, FH, and metabolic risk factors did not. Both ε4 status and DTI correlated with change in immediate recall.
Longitudinal EM changes in cognitively intact, healthy elders can be predicted by disruption of the MTL WM microstructure. These results are derived from a sample with a disproportionately higher genetic risk for AD, suggesting that the observed WM disruption in MTL pathways may be related to early neuropathological changes associated with the preclinical stage of AD. (JINS, 2016,22, 1005–1015)
- Acosta-Cabronero, J., Williams, G.B., Pengas, G., & Nestor, P.J. (2010). Absolute diffusivities define the landscape of white matter degeneration in Alzheimer’s disease. Brain, 133, 529–539. CrossRef Google Scholar PubMed
- Agosta, F., Pievani, M., Sala, S., Geroldi, C., Galluzzi, S., Frisoni, G.B., & Filippi, M. (2011). White matter damage in Alzheimer disease and its relationship to gray matter atrophy. Radiology, 258(3), 853–863. doi: 10.1148/radiol.10101284 CrossRef Google Scholar PubMed
- Albert, M.S., Moss, M.B., Tanzi, R., & Jones, K. (2001). Preclinical prediction of AD using neuropsychological tests. Journal of the International Neuropsychological Society, 7(5), 631–639. CrossRef Google Scholar PubMed
- Alves, G.S., O’Dwyer, L., Jurcoane, A., Oertel-Knochel, V., Knochel, C., Prvulovic, D., & Laks, J. (2012). Different patterns of white matter degeneration using multiple diffusion indices and volumetric data in mild cognitive impairment and Alzheimer patients. PLoS One, 7(12), e52859. doi: 10.1371/journal.pone.0052859 CrossRef Google Scholar PubMed
- Backman, L., Small, B.J., & Fratiglioni, L. (2001). Stability of the preclinical episodic memory deficit in Alzheimer’s disease. Brain, 124(Pt 1), 96–102. CrossRef Google Scholar
- Balthazar, M.L., Yasuda, C.L., Cendes, F., & Damasceno, B.P. (2010). Learning, retrieval, and recognition are compromised in aMCI and mild AD: Are distinct episodic memory processes mediated by the same anatomical structures? Journal of the International Neuropsychological Society, 16(1), 205–209. doi: 10.1017/s1355617709990956 CrossRef Google Scholar PubMed
- Bartzokis, G., Sultzer, D., Lu, P.H., Nuechterlein, K.H., Mintz, J., & Cummings, J.L. (2004). Heterogeneous age-related breakdown of white matter structural integrity: Implications for cortical “disconnection” in aging and Alzheimer’s disease. Neurobiology of Aging, 25, 843–851. CrossRef Google Scholar PubMed
- Behrens, M.W., Woolrich, M., Jenkinson, H., Johansen-Berg, R.G., Nunes, S., & Clare, P.M. (2003). Characterization and propagation of uncertainty in diffusion-weighted MR imaging. Magnetic Resonance in Medicine, 50, 1077–1088. CrossRef Google Scholar PubMed
- Benjamini, Y., & Hochberg, Y. (1995). Controlling the false discovery rate: A practical and powerful approach to multiple testing. Journal of the Royal Statistical Society: Series B (Statistical Methodology), 57, 289–300. Google Scholar
- Bennett, I.J., & Madden, D.J. (2014). Disconnected aging: Cerebral white matter integrity and age-related differences in cognition. Neuroscience, 276, 187–205. doi: 10.1016/j.neuroscience.2013.11.026 CrossRef Google Scholar PubMed
- Blacker, D., Lee, H., Muzikansky, A., Martin, E.C., Tanzi, R., McArdle, J.J., & Albert, M. (2007). Neuropsychological measures in normal individuals that predict subsequent cognitive decline. Archives of Neurology, 64(6), 862–871. doi: 10.1001/archneur.64.6.862 CrossRef Google Scholar
- Bondi, M.W., Salmon, D.P., Galasko, D., Thomas, R.G., & Thal, L.J. (1999). Neuropsychological function and apolipoprotein E genotype in the preclinical detection of Alzheimer’s disease. Psychology and Aging, 14(2), 295–303. CrossRef Google Scholar PubMed
- Canu, E., McLaren, D.G., Fitzgerald, M.E., Bendlin, B.B., Zoccatelli, G., Alessandrini, F., & Frisoni, G.B. (2010). Microstructural diffusion changes are independent of macrostructural volume loss in moderate to severe Alzheimer’s disease. Journal of Alzheimer’s Disease, 19(3), 963–976. doi: 10.3233/jad-2010-1295 CrossRef Google Scholar PubMed
- Cardenas, V.A., Chao, L.L., Studholme, C., Yaffe, K., Miller, B.L., Madison, C., & Weiner, M.W. (2011). Brain atrophy associated with baseline and longitudinal measures of cognition. Neurobiology of Aging, 32(4), 572–580. doi: 10.1016/j.neurobiolaging.2009.04.011 CrossRef Google Scholar PubMed
- Caselli, R.J., Reiman, E.M., Osborne, D., Hentz, J.G., Baxter, L.C., Hernandez, J.L., & Alexander, G.G. (2004). Longitudinal changes in cognition and behavior in asymptomatic carriers of the APOE e4 allele. Neurology, 62(11), 1990–1995. CrossRef Google Scholar PubMed
- Christidi, F., Zalonis, I., Kyriazi, S., Rentzos, M., Karavasilis, E., Wilde, E.A., & Evdokimidis, I. (2014). Uncinate fasciculus microstructure and verbal episodic memory in amyotrophic lateral sclerosis: A diffusion tensor imaging and neuropsychological study. Brain Imaging and Behavior, 8(4), 497–505. doi: 10.1007/s11682-013-9271-y CrossRef Google Scholar PubMed
- Cox, R. (1996). AFNI: Sotware for analysis and visualization of functional magnetic resonance images. Computers and Biomedical Research, 29, 162–173. CrossRef Google Scholar
- den Heijer, T., Geerlings, M.I., Hoebeek, F.E., Hofman, A., Koudstaal, P.J., & Breteler, M.M. (2006). Use of hippocampal and amygdalar volumes on magnetic resonance imaging to predict dementia in cognitively intact elderly people. Archives of General Psychiatry, 63(1), 57–62. doi: 10.1001/archpsyc.63.1.57 CrossRef Google Scholar PubMed
- Dickerson, B.C., & Eichenbaum, H. (2010). The episodic memory system: Neurocircuitry and disorders. Neuropsychopharmacology, 35(1), 86–104. doi: 10.1038/npp.2009.126 CrossRef Google Scholar PubMed
- Edmonds, E.C., Delano-Wood, L., Galasko, D.R., Salmon, D.P., & Bondi, M.W. (2015). Subtle cognitive decline and biomarker staging in preclinical Alzheimer’s disease. Journal of Alzheimer’s Disease, 47(1), 231–242. doi: 10.3233/jad-150128 CrossRef Google Scholar
- Ellis, K.A., Lim, Y.Y., Harrington, K., Ames, D., Bush, A.I., Darby, D., … AIBL Research Group. (2013). Decline in cognitive function over 18 months in healthy older adults with high amyloid-beta. Journal of Alzheimer’s Disease, 34(4), 861–871. doi: 10.3233/jad-122170 Google Scholar
- Ezzati, A., Katz, M.J., Lipton, M.L., Zimmerman, M.E., & Lipton, R.B. (2016). Hippocampal volume and cingulum bundle fractional anisotropy are independently associated with verbal memory in older adults. Brain Imaging and Behavior, 10, 652–659. doi: 10.1007/s11682-015-9452-y CrossRef Google Scholar
- Fagan, A.M., Roe, C.M., Xiong, C., Mintun, M.A., Morris, J.C., & Holtzman, D.M. (2007). Cerebrospinal fluid tau/beta-amyloid(42) ratio as a prediction of cognitive decline in nondemented older adults. Archives of Neurology, 64(3), 343–349. doi: 10.1001/archneur.64.3.noc60123 CrossRef Google Scholar
- Fischl, B., Salat, D.H., Busa, E., Albert, M., Dieterich, M., & Haselgrove, C. (2004). Whole brain segmentation: Automated labeling of neuroanatomical structures in the human brain. Neuron, 33, 341–355. CrossRef Google Scholar PubMed
- Fletcher, E., Raman, M., Huebner, P., Liu, A., Mungas, D., Carmichael, O., & DeCarli, C. (2013). Loss of fornix white matter volume as a predictor of cognitive impairment in cognitively normal elderly individuals. JAMA Neurology, 70(11), 1389–1395. doi: 10.1001/jamaneurol.2013.3263 CrossRef Google Scholar PubMed
- Folstein, M.F., Folstein, S.E., & McHugh, P.R. (1975). “Mini-Mental State”: A practical method for grading the cognitive state of patients for the clinician. Journal of Psychiatry Research, 12, 189–198. CrossRef Google Scholar PubMed
- Fu, J.L., Liu, Y., Li, Y.M., Chang, C., & Li, W.B. (2014). Use of diffusion tensor imaging for evaluating changes in the microstructural integrity of white matter over 3 years in patients with amnesic-type mild cognitive impairment converting to Alzheimer’s disease. Journal of Neuroimaging, 24(4), 343–348. doi: 10.1111/jon.12061 CrossRef Google Scholar PubMed
- Geschwind, N. (1965a). Disconnexion syndromes in animals and man. I. Brain, 88(2), 237–294. CrossRef Google Scholar PubMed
- Geschwind, N. (1965b). Disconnexion syndromes in animals and man. II. Brain, 88(3), 585–644. CrossRef Google Scholar PubMed
- Golomb, J., Kluger, A., de Leon, M.J., Ferris, S.H., Mittelman, M., Cohen, J., & George, A.E. (1996). Hippocampal formation size predicts declining memory performance in normal aging. Neurology, 47(3), 810–813. CrossRef Google Scholar PubMed
- Hamel, R., Kohler, S., Sistermans, N., Koene, T., Pijnenburg, Y., van der Flier, W., & Ramakers, I. (2015). The trajectory of cognitive decline in the pre-dementia phase in memory clinic visitors: Findings from the 4C-MCI study. Psychological Medicine, 45(7), 1509–1519. doi: 10.1017/s0033291714002645 CrossRef Google Scholar PubMed
- Hiyoshi-Taniguchi, K., Oishi, N., Namiki, C., Miyata, J., Murai, T., Cichocki, A., & Fukuyama, H. (2015). The uncinate fasciculus as a predictor of conversion from aMCI to Alzheimer disease. Journal of Neuroimaging, 25, 748–753. doi: 10.1111/jon.12196 CrossRef Google Scholar PubMed
- Jurica, P.J., Leittten, C.L., & Mattis, S. (2001). Dementia Rating Scale-2 professional manual. Lutz, FL: Psychological Assessment Resources. Google Scholar
- Lawton, M.P., & Brody, E.M. (1969). Assessment of older people: Self-maintaining instrumental activities of daily living. Gerontologist, 9, 179–186. CrossRef Google Scholar PubMed
- Li, W., Muftuler, L.T., Chen, G., Ward, B.D., Budde, M.D., Jones, J.L., & Goveas, J.S. (2014). Effects of the coexistence of late-life depression and mild cognitive impairment on white matter microstructure. Journal of the Neurological Sciences, 338(1-2), 46–56. doi: 10.1016/j.jns.2013.12.016 CrossRef Google Scholar PubMed
- Lim, Y.Y., Pietrzak, R.H., Ellis, K.A., Jaeger, J., Harrington, K., Ashwood, T., & Maruff, P. (2013). Rapid decline in episodic memory in healthy older adults with high amyloid-beta. Journal of Alzheimer’s Disease, 33(3), 675–679. doi: 10.3233/jad-2012-121516 Google Scholar
- Loewenstein, D.A., Barker, W.W., Chang, J.Y., Apicella, A., Yoshii, F., Kothari, P., & Duara, R. (1989). Predominant left hemisphere metabolic dysfunction in dementia. Archives of Neurology, 46(2), 146–152. CrossRef Google Scholar PubMed
- Mattsson, P., Forsberg, A., Persson, J., Nyberg, L., Nilsson, L.G., Halldin, C., & Farde, L. (2015). beta-Amyloid binding in elderly subjects with declining or stable episodic memory function measured with PET and [(1)(1)C]AZD2184. European Journal of Nuclear Medicine and Molecular Imaging, 42(10), 1507–1511. doi: 10.1007/s00259-015-3103-9 CrossRef Google Scholar
- McSweeny, A.J., Naugle, R.I., Chelune, G.J., & Luders, H. (1993). “T scores for change”: An illustration of a regression approach to depicting change in clinical neuropsychology. The Clinical Neuropsychologist, 7, 300–312. CrossRef Google Scholar
- Mesulam, M.M. (1990). Large-scale neurocognitive networks and distributed processing for attention, language, and memory. Annals of Neurology, 28(5), 597–613. doi: 10.1002/ana.410280502 CrossRef Google Scholar
- Metzler-Baddeley, C., Hunt, S., Jones, D.K., Leemans, A., Aggleton, J.P., & O’Sullivan, M.J. (2012). Temporal association tracts and the breakdown of episodic memory in mild cognitive impairment. Neurology, 79(23), 2233–2240. doi: 10.1212/WNL.0b013e31827689e8 CrossRef Google Scholar PubMed
- Mistridis, P., Krumm, S., Monsch, A.U., Berres, M., & Taylor, K.I. (2015). The 12 years preceding mild cognitive impairment due to Alzheimer’s disease: The temporal emergence of cognitive decline. Journal of Alzheimer’s Disease, 48(4), 1095–1107. doi: 10.3233/jad-150137 CrossRef Google Scholar PubMed
- Moghekar, A., Li, S., Lu, Y., Li, M., Wang, M.C., & Albert, M., … Biocard Research Team. (2013). CSF biomarker changes precede symptom onset of mild cognitive impairment. Neurology, 81(20), 1753–1758. doi: 10.1212/01.wnl.0000435558.98447.17 CrossRef Google Scholar PubMed
- Mori, S., Oishi, K., Jiang, H., Jiang, L., Li, X., Akhter, K., & Mazziotta, J. (2008). Stereotaxic white matter atlas based on diffusion tensor imaging in an ICBM template. Neuroimage, 40(2), 570–582. doi: 10.1016/j.neuroimage.2007.12.035 CrossRef Google Scholar
- Nir, T.M., Jahanshad, N., Villalon-Reina, J.E., Toga, A.W., Jack, C.R., & Weiner, M.W., … Alzheimer’s Disease Neuroimaging Initiative. (2013). Effectiveness of regional DTI measures in distinguishing Alzheimer’s disease, MCI, and normal aging. Neuroimage: Clinical, 3, 180–195. doi: 10.1016/j.nicl.2013.07.006 CrossRef Google Scholar PubMed
- O’Dwyer, L., Lamberton, F., Bokde, A.L., Ewers, M., Faluyi, Y.O., Tanner, C., & Hampel, H. (2011). Multiple indices of diffusion identifies white matter damage in mild cognitive impairment and Alzheimer’s disease. PLoS One, 6(6), e21745. doi: 10.1371/journal.pone.0021745 CrossRef Google Scholar PubMed
- Oishi, K., Faria, A., van Zijl, P.C.M., & Mori, S. (2011). MRI atlas of human white matter, (2nd ed.). London: Elsevier. Google Scholar
- Papp, K.V., Amariglio, R.E., Mormino, E.C., Hedden, T., Dekhytar, M., Johnson, K.A., & Rentz, D.M. (2015). Free and cued memory in relation to biomarker-defined abnormalities in clinically normal older adults and those at risk for Alzheimer’s disease. Neuropsychologia, 73, 169–175. doi: 10.1016/j.neuropsychologia.2015.04.034 CrossRef Google Scholar PubMed
- Pierpaoli, C., Barnett, A., Pajevic, S., Chen, R., Penix, L.R., Virta, A., & Basser, P. (2001). Water diffusion changes in Wallerian degeneration and their dependence on white matter architecture. Neuroimage, 13(6 Pt 1), 1174–1185. doi: 10.1006/nimg.2001.0765 CrossRef Google Scholar PubMed
- Ray, N.J., Metzler-Baddeley, C., Khondoker, M.R., Grothe, M.J., Teipel, S., Wright, P., & O’Sullivan, M.J. (2015). Cholinergic basal forebrain structure influences the reconfiguration of white matter connections to support residual memory in mild cognitive impairment. Journal of Neuroscience, 35(2), 739–747. doi: 10.1523/jneurosci.3617-14.2015 CrossRef Google Scholar PubMed
- Raz, N., & Rodrigue, K.M. (2006). Differential aging of the brain: Patterns, cognitive correlates and modifiers. Neuroscience and Biobehavioral Reviews, 30(6), 730–748. doi: 10.1016/j.neubiorev.2006.07.001 CrossRef Google Scholar PubMed
- Remy, F., Vayssiere, N., Saint-Aubert, L., Barbeau, E., & Pariente, J. (2015). White matter disruption at the prodromal stage of Alzheimer’s disease: Relationships with hippocampal atrophy and episodic memory performance. Neuroimage: Clinical, 7, 482–492. doi: 10.1016/j.nicl.2015.01.014 CrossRef Google Scholar PubMed
- Rey, A. (1958). L’examen clinique en psychologie. Paris: Presses Universitaires de France. Google Scholar
- Rosen, A.C., Prull, M.W., Gabrieli, J.D., Stoub, T., O’Hara, R., Friedman, L., & deToledo-Morrell, L. (2003). Differential associations between entorhinal and hippocampal volumes and memory performance in older adults. Behavioral Neuroscience, 117(6), 1150–1160. doi: 10.1037/0735-7044.117.6.1150 CrossRef Google Scholar PubMed
- Salat, D.H., Tuch, D.S., van der Kouwe, A.J., Greve, D.N., Pappu, V., & Lee, S.Y. (2010). White matter pathology isolates the hippocampal formation in Alzheimer’s disease. Neurobiology of Aging, 31, 244–256. CrossRef Google Scholar
- Saunders, A.M., Hulette, O., Welsh-Bohmer, K.A., Schmechel, D.E., Crain, B., & Burke, J.R. (1996). Specificity, sensitivity, and predictive value of apolipoprotein-E genotyping for sporadic Alzheimer’s disease. Lancet, 348, 90–93. CrossRef Google Scholar PubMed
- Schaeffer, D.J., Krafft, C.E., Schwarz, N.F., Chi, L., Rodrigue, A.L., Pierce, J.E., & McDowell, J.E. (2014). The relationship between uncinate fasciculus white matter integrity and verbal memory proficiency in children. Neuroreport, 25(12), 921–925. doi: 10.1097/wnr.0000000000000204 CrossRef Google Scholar PubMed
- Seidenberg, M., Guidotti, L., Nielson, K.A., Woodard, J.L., Durgerian, S., Antuono, P., & Rao, S.M. (2009). Semantic memory activation in individuals at risk for developing Alzheimer disease. Neurology, 73(8), 612–620. doi: 10.1212/WNL.0b013e3181b389ad CrossRef Google Scholar PubMed
- Smith, S.M. (2002). Fast robust automated brain extraction. Human Brain Mapping, 17, 143–155. CrossRef Google Scholar PubMed
- Smith, S.M., Jenkinson, M., Johansen-Berg, H., Ruckert, D., Nichols, T.E., & Mackay, C.E. (2006). Tract-based spatial statistics: Voxelwise analysis of multi-subject diffusion data. Neuroimage, 31, 1487–1505. CrossRef Google Scholar PubMed
- Song, S.K., Sun, S.W., Ju, W.K., Lin, S.J., Cross, A.H., & Neufeld, A.H. (2003). Diffusion tensor imaging detects and differentiates axon and myelin degeneration in mouse optic nerve after retinal ischemia. Neuroimage, 20, 1714–1722. CrossRef Google Scholar PubMed
- Song, S.K., Sun, S.W., Ramsbottom, M.J., Chang, C., Russell, J., & Cross, A.H. (2002). Dysmyelination revealed through MRI as increased radial (but unchanged axial) diffusion of water. Neuroimage, 17(3), 1429–1436. CrossRef Google Scholar
- Sperling, R.A., Aisen, P.S., Beckett, L.A., Bennett, D.A., Craft, S., Fagan, A.M., & Phelps, C.H. (2011). Toward defining the preclinical stages of Alzheimer’s disease: Recommendations from the National Institute on Aging-Alzheimer’s Association workgroups on diagnostic guidelines for Alzheimer’s disease. Alzheimers and Dementia, 7(3), 280–292. doi: 10.1016/j.jalz.2011.03.003 CrossRef Google Scholar PubMed
- Thai, C., Lim, Y.Y., Villemagne, V.L., Laws, S.M., Ames, D., & Ellis, K.A., … Australian Imaging, Biomarkers and Lifestyle (AIBL) Research Group. (2015). Amyloid-related memory decline in preclinical Alzheimer’s disease is dependent on APOE epsilon4 and is detectable over 18-months. PLoS One, 10(10), e0139082. doi: 10.1371/journal.pone.0139082 CrossRef Google Scholar PubMed
- Thompson, P.M., Hayashi, K.M., de Zubicaray, G., Janke, A.L., Rose, S.E., Semple, J., & Toga, A.W. (2003). Dynamics of gray matter loss in Alzheimer’s disease. Journal of Neuroscience, 23(3), 994–1005. Google Scholar PubMed
- Tucker, L.R., Damarin, F., & Messick, S. (1966). A base-free measure of change. Psychometrika, 31(4), 457–473. CrossRef Google Scholar
- Von Der Heide, R.J., Skipper, L.M., Klobusicky, E., & Olson, I.R. (2013). Dissecting the uncinate fasciculus: Disorders, controversies and a hypothesis. Brain, 136(6), 1692–1707. doi: 10.1093/brain/awt094 CrossRef Google Scholar
- Vyhnalek, M., Nikolai, T., Andel, R., Nedelska, Z., Rubinova, E., Markova, H., & Hort, J. (2014). Neuropsychological correlates of hippocampal atrophy in memory testing in nondemented older adults. Journal of Alzheimer’s Disease, 42(Suppl 3), S81–S90. doi: 10.3233/jad-132642 Google Scholar PubMed
- Wang, R., Fratiglioni, L., Laukka, E.J., Lovden, M., Kalpouzos, G., Keller, L., & Qiu, C. (2015). Effects of vascular risk factors and APOE epsilon4 on white matter integrity and cognitive decline. Neurology, 84(11), 1128–1135. doi: 10.1212/wnl.0000000000001379 CrossRef Google Scholar
- Wheeler-Kingshott, C.A., & Cercignani, M. (2009). About “axial” and “radial” diffusivities. Magnetic Resonance in Medicine, 61(5), 1255–1260. doi: 10.1002/mrm.21965 CrossRef Google Scholar PubMed
- Wisse, L.E., Reijmer, Y.D., ter Telgte, A., Kuijf, H.J., Leemans, A., & Luijten, P.R., … Utrecht Vascular Cognitive Impairment Study Group. (2015). Hippocampal disconnection in early Alzheimer’s disease: A 7 tesla MRI study. Journal of Alzheimer’s Disease, 45(4), 1247–1256. doi: 10.3233/jad-142994 Google Scholar PubMed
- Woodard, J.L., Seidenberg, M., Nielson, K.A., Antuono, P., Guidotti, L., Durgerian, S., & Rao, S.M. (2009). Semantic memory activation in amnestic mild cognitive impairment. Brain, 132(Pt 8), 2068–2078. doi: 10.1093/brain/awp157 CrossRef Google Scholar PubMed
- Woodard, J.L., Seidenberg, M., Nielson, K.A., Smith, J.C., Antuono, P., Durgerian, S., & Rao, S.M. (2010). Prediction of cognitive decline in healthy older adults using fMRI. Journal of Alzheimer’s Disease, 21, 871–885. CrossRef Google Scholar PubMed
- Woodard, J.L., Sugarman, M.A., Nielson, K.A., Smith, J.C., Seidenberg, M., Durgerian, S., & Rao, S.M. (2012). Lifestyle and genetic contributions to cognitive decline and hippocampal structure and function in healthy aging. Current Alzheimer’s Research, 9(4), 436–446. CrossRef Google Scholar PubMed
- Yasmin, H., Nakata, Y., Aoki, S., Abe, O., Sato, N., Nemoto, K., & Ohtomo, K. (2008). Diffusion abnormalities of the uncinate fasciculus in Alzheimer’s disease: Diffusion tensor tract-specific analysis using a new method to measure the core of the tract. Neuroradiology, 50(4), 293–299. doi: 10.1007/s00234-007-0353-7 CrossRef Google Scholar PubMed
- Yesavage, J.A., Brink, T.L., Rose, T.L., Lum, O., Huang, V., & Adey, M. (1983). Development and validation of a geriatric depression screening scale: A preliminary report. Journal of Psychiatry Research, 17, 37–49. CrossRef Google Scholar PubMed
- Zhang, Y., Schuff, N., Jahng, G.H., Bayne, W., Mori, S., Schad, L., & Weiner, M.W. (2007). Diffusion tensor imaging of cingulum fibers in mild cognitive impairment and Alzheimer disease. Neurology, 68(1), 13–19. doi: 10.1212/01.wnl.0000250326.77323.01 CrossRef Google Scholar PubMed
- Zhuang, L., Sachdev, P.S., Trollor, J.N., Kochan, N.A., Reppermund, S., Brodaty, H., & Wen, W. (2012). Microstructural white matter changes in cognitively normal individuals at risk of amnestic MCI. Neurology, 79(8), 748–754. doi: 10.1212/WNL.0b013e3182661f4d CrossRef Google Scholar PubMed
- Zhuang, L., Sachdev, P.S., Trollor, J.N., Reppermund, S., Kochan, N.A., Brodaty, H., & Wen, W. (2013). Microstructural white matter changes, not hippocampal atrophy, detect early amnestic mild cognitive impairment. PLoS One, 8(3), e58887. doi: 10.1371/journal.pone.0058887 CrossRef Google Scholar
The aim of this study was to describe cognitive, academic, and psychosocial outcomes after an incident demyelinating event (acquired demyelinating syndromes, ADS) in childhood and to investigate the contribution of brain lesions and confirmed MS diagnosis on outcome.
Thirty-six patients with ADS (mean age=12.2 years,SD=2.7, range: 7–16 years) underwent brain MRI scans at presentation and at 6-months follow-up. T2-weighted lesions on MRI were assessed using a binary classification. At 6-months follow-up, patients underwent neuropsychological evaluation and were compared with 42 healthy controls.
Cognitive, academic, and behavioral outcomes did not differ between the patients with ADS and controls. Three of 36 patients (8.3%) were identified with cognitive impairment, as determined by performance falling ≤1.5SDbelow normative values on more than four independent tests in the battery. Poor performance on a visuomotor integration task was most common, observed among 6/32 patients, but this did not differ significantly from controls. Twelve of 36 patients received a diagnosis of MS within 3 years post-ADS. Patients with MS did not differ from children with monophasic ADS in terms of cognitive performance at the 6-months follow-up. Fatigue symptoms were reported in 50% of patients, irrespective of MS diagnosis. Presence of brain lesions at onset and 6 months post-incident demyelinating event did not associate with cognitive outcome.
Children with ADS experience a favorable short-term neurocognitive outcome, even those confirmed to have MS. Longitudinal evaluations of children with monophasic ADS and MS are required to determine the possibility of late-emerging sequelae and their time course. (JINS, 2016,22, 1050–1060)
- Amato, M.P., Goretti, B., Ghezzi, A., Lori, S., Zipoli, V., Moiola, L., & Trojano, M. (2010). Cognitive and psychosocial features in childhood and juvenile MS: Two-year follow-up. Neurology, 75(13), 1134–1140. http://doi.org/10.1212/WNL.0b013e3181f4d821 CrossRef Google Scholar PubMed
- Amato, M.P., Goretti, B., Ghezzi, A., Lori, S., Zipoli, V., & Portaccio, E., . . . Multiple Sclereosis Study Group of the Italian Neurological Society. (2008). Cognitive and psychosocial features of childhood and juvenile MS. Neurology, 70(20), 1891–1897. http://doi.org/10.1212/01.wnl.0000312276.23177.fa CrossRef Google Scholar PubMed
- Amato, M.P., Goretti, B., Ghezzi, A., Niccolai, C., Lori, S., Moiola, L., & Trojano, M. (2014). Neuropsychological features in childhood and juvenile multiple sclerosis. Neurology, 83(16), 1432–1438. http://doi.org/doi:10.1212/WNL.0000000000000885 CrossRef Google Scholar PubMed
- Aubert-Broche, B., Fonov, V., Ghassemi, R., Narayanan, S., Arnold, D.L., Banwell, B., & Collins, D.L. (2011). Regional brain atrophy in children with multiple sclerosis. Neuroimage, 58(2), 409–415. http://doi.org/10.1016/j.neuroimage.2011.03.025 CrossRef Google Scholar PubMed
- Banwell, B., Bar-Or, A., Arnold, D.L., Sadovnick, D., Narayanan, S., McGowan, M., & Marrie, R.A. (2011). Clinical, environmental, and genetic determinants of multiple sclerosis in children with acute demyelination: A prospective national cohort study. Lancet Neurology, 10(5), 436–445. http://doi.org/10.1016/S1474-4422(11)70045-X CrossRef Google Scholar PubMed
- Banwell, B., Kennedy, J., Sadovnick, D., Arnold, D.L., Magalhaes, S., Wambera, K., & Bar-Or, A. (2009). Incidence of acquired demyelination of the CNS in Canadian children. Neurology, 72(3), 232–239. http://doi.org/10.1212/01.wnl.0000339482.84392.bd CrossRef Google Scholar PubMed
- Banwell, B.L., & Anderson, P.E. (2005). The cognitive burden of multiple sclerosis in children. Neurology, 64(5), 891–894. Retrieved from http://www.ncbi.nlm.nih.gov/entrez/query.fcgi?cmd=Retrieve&db=PubMed&dopt=Citation&list_uids=15753431 CrossRef Google Scholar PubMed
- Barratt, W. (2006). The Barratt Simplified Measure of Social Status. Available at: http://wbarratt.indstate.edu. Google Scholar
- Beery, K. (1997). The Beery-Buktenica developmental test of visual-motor integration (4th ed.). Parsippany: Modern Curriculum Press. Google Scholar
- Brooks, B.L., Sherman, E.M.S., & Iverson, G.L. (2010). Healthy children get low scores too: Prevalence of low scores on the nepsy-ii in preschoolers, children, and adolescents. Archives of Clinical Neuropsychology, 25(3), 182–190. http://doi.org/10.1093/arclin/acq005 CrossRef Google Scholar PubMed
- Buchanan, R.J., Chakravorty, B.J., Tyry, T., Hatcher, W., & Vollmer, T. (2009). Age-related comparisons of people with multiple sclerosis: Demographic, disease, and treatment characteristics. Neurorehabilitation, 25(4), 271–278. http://doi.org/T446800094610453 [pii]/r10.3233/NRE-2009-0525 Google Scholar PubMed
- Charvet, L.E., O’Donnell, E.H., Belman, A.L., Chitnis, T., Ness, J.M., Parrish, J., & Krupp, L.B. (2014). Longitudinal evaluation of cognitive functioning in pediatric multiple sclerosis: Report from the US Pediatric Multiple Sclerosis Network. Multiple Sclerosis, 20(11), 1502–1510. http://doi.org/10.1177/1352458514527862 CrossRef Google Scholar PubMed
- Cohen, J.A., Reingold, S.C., Polman, C.H., & Wolinsky, J.S. (2012). Disability outcome measures in multiple sclerosis clinical trials: Current status and future prospects. The Lancet Neurology, 11(5), 467–476. http://doi.org/10.1016/S1474-4422(12)70059-5 CrossRef Google Scholar PubMed
- Dale, R.C., Brilot, F., & Banwell, B. (2009). Pediatric central nervous system inflammatory demyelination: acute disseminated encephalomyelitis, clinically isolated syndromes, neuromyelitis optica, and multiple sclerosis. Current Opinion in Neurology, 22(3), 233–240. http://doi.org/10.1097/WCO.0b013e32832b4c47 CrossRef Google Scholar PubMed
- Deery, B., Anderson, V., Jacobs, R., Neale, J., & Kornberg, A. (2010). Childhood MS and ADEM: Investigation and comparison of neurocognitive features in children. Developmental Neuropsychology, 35(5), 506–521. http://doi.org/10.1080/87565641.2010.494921 CrossRef Google Scholar PubMed
- Delis, D.C., Kaplan, E., & Kramer, J.H. (2001). Delis–Kaplan Executive Function SystemTM (D–KEFS). San Antonio: Pearson. Google Scholar
- Eskildsen, S.F., Coupé, P., Fonov, V., Manjón, J.V., Leung, K.K., Guizard, N., & Collins, D.L. (2012). BEaST: Brain extraction based on nonlocal segmentation technique. Neuroimage, 59(3), 2362–2373. http://doi.org/10.1016/j.neuroimage.2011.09.012 CrossRef Google Scholar PubMed
- Evans, A., & Brain Development Cooperative Group. (2006). The NIH MRI study of normal brain development. Neuroimage, 30, 184–202. CrossRef Google Scholar PubMed
- Fonov, V., Evans, A.C., Botteron, K., Almli, C.R., McKinstry, R.C., Collins, D.L., & Brain Development Cooperative Group. (2011). Unbiased average age-appropriate atlases for pediatric studies. Neuroimage, 54(1), 313–327. http://doi.org/10.1016/j.neuroimage.2010.07.033 CrossRef Google Scholar PubMed
- Ghassemi, R., Antel, S.B., Narayanan, S., Francis, S.J., Bar-Or, A., & Sadovnick, A.D., . . . Canadian Pediatric Demyelinating Disease Study Group. (2008). Lesion distribution in children with clinically isolated syndromes. Annals of Neurology, 63(3), 401–405. http://doi.org/10.1002/ana.21322 CrossRef Google Scholar PubMed
- Hahn, C.D., Miles, B.S., MacGregor, D.L., Blaser, S.I., Banwell, B.L., & Hetherington, C.R. (2003). Neurocognitive outcome after acute disseminated encephalomyelitis. Pediatric Neurology, 29(2), 117–123. Retrieved from http://www.ncbi.nlm.nih.gov/entrez/query.fcgi?cmd=Retrieve&db=PubMed&dopt=Citation&list_uids=14580654 CrossRef Google Scholar PubMed
- Harder, L.L., Holland, A.A., Frohman, E., Graves, D., & Greenberg, B.M. (2013). Cognitive functioning in pediatric transverse myelitis. Multiple Sclerosis, 19(7), 947–952. http://doi.org/10.1177/1352458512466606; 10.1177/1352458512466606 CrossRef Google Scholar PubMed
- Hosseini, B., Flora, D.B., Banwell, B.L., & Till, C. (2014). Age of onset as a moderator of cognitive decline in pediatric-onset multiple sclerosis. Journal of the International Neuropsychological Society, 20, 796–804. http://doi.org/10.1017/S1355617714000642 CrossRef Google Scholar PubMed
- Ingraham, L.G., & Aiken, C.B. (1996). Empirical approach to determining criteria for abnormality in test batteries with multiple measures. Neuropsychology, 10(1), 120–124. CrossRef Google Scholar
- Jacobs, R.K., Anderson, V.A., Neale, J.L., Shield, L.K., & Kornberg, A.J. (2004). Neuropsychological outcome after acute disseminated encephalomyelitis: Impact of age at illness onset. Pediatric Neurology, 31(3), 191–197. Retrieved from http://www.ncbi.nlm.nih.gov/entrez/query.fcgi?cmd=Retrieve&db=PubMed&dopt=Citation&list_uids=15351018 CrossRef Google Scholar PubMed
- Jayakrishnan, M.P., & Krishnakumar, P. (2010). Clinical profile of acute disseminated encephalomyelitis in children. Journal of Pediatric Neurosciences, 5(2), 111–114. http://doi.org/10.4103/1817-1745.76098; 10.4103/1817-1745.76098 CrossRef Google Scholar PubMed
- Julian, L., Serafin, D., Charvet, L., Ackerson, J., Benedict, R., & Braaten, E., . . . Network of Pediatric MS Centers of Excellence. (2012). Cognitive impairment occurs in children and adolescents with multiple sclerosis: Results from a United States network. Journal of Child Neurology, 28(1), 102–107. http://doi.org/10.1177/0883073812464816; 10.1177/0883073812464816 CrossRef Google Scholar PubMed
- Kerbrat, A., Aubert-Broche, B., Fonov, V., Narayanan, S., Sled, J.G., Arnold, D.A., & Collins, D.L. (2012). Reduced head and brain size for age and disproportionately smaller thalami in child-onset MS. Neurology, 78(3), 194–201. http://doi.org/10.1212/WNL.0b013e318240799a CrossRef Google Scholar PubMed
- Krupp, L.B., Tardieu, M., Amato, M.P., Banwell, B., Chitnis, T., Dale, R.C., & Wassmer, E. (2013). International Pediatric Multiple Sclerosis Study Group criteria for pediatric multiple sclerosis and immune-mediated central nervous system demyelinating disorders: revisions to the 2007 definitions. Multiple Sclerosis, 19(10), 1261–1267. CrossRef Google Scholar
- Kuni, B.J., Banwell, B.L., & Till, C. (2012). Cognitive and behavioral outcomes in individuals with a history of acute disseminated encephalomyelitis (ADEM). Developmental Neuropsychology, 37(8), 682–696. http://doi.org/10.1080/87565641.2012.690799 CrossRef Google Scholar
- Kurtzke, J. (1983). Rating neurologic impairment in multiple sclerosis: an expanded disability status scale (EDSS). Neurology, 33(11), 1444–1452. CrossRef Google Scholar
- MacAllister, W.S., Belman, A.L., Milazzo, M., Weisbrot, D.M., Christodoulou, C., Scherl, W.F., & Krupp, L.B. (2005). Cognitive functioning in children and adolescents with multiple sclerosis. Neurology, 64(8), 1422–1425. Retrieved from http://www.ncbi.nlm.nih.gov/entrez/query.fcgi?cmd=Retrieve&db=PubMed&dopt=Citation&list_uids=15851734 CrossRef Google Scholar PubMed
- MacAllister, W.S., Christodoulou, C., Milazzo, M., & Krupp, L.B. (2007). Longitudinal neuropsychological assessment in pediatric multiple sclerosis. Developmental Neuropsychology, 32(2), 625–644. http://doi.org/10.1080/87565640701375872 CrossRef Google Scholar PubMed
- Marin, S.E., Banwell, B.B., & Till, C. (2013). Cognitive trajectories in 4 patients with pediatric-onset multiple sclerosis: Serial evaluation over a decade. Journal of Child Neurology, 28(12), 1577–1586. Retrieved from http://www.ncbi.nlm.nih.gov/pubmed/23143723 CrossRef Google Scholar
- O’Mahony, J., Marrie, R.A., Laporte, A., Yeh, E.A., Bar-Or, A., Phan, C., & Banwell, B. (2015). Recovery from central nervous system acute demyelination in children. Pediatrics. http://doi.org/10.1542/peds.2015-0028 Google Scholar
- Polman, C.H., Reingold, S.C., Banwell, B., Clanet, M., Cohen, J.A., Filippi, M., & Wolinsky, J.S. (2011). Diagnostic criteria for multiple sclerosis: 2010 Revisions to the McDonald criteria. Annals of Neurology, 69(2), 292–302. http://doi.org/10.1002/ana.22366 CrossRef Google Scholar PubMed
- Reynolds, C.R., & Kamphaus, R.W. (2004).Behavior Assessment System for Children (BASC-2)(2nd ed.). Bloomington: Pearson Assessments. Google Scholar
- Reynolds, C.R., & Voress, J.K. (2007).Test of Memory and Learning (TOMAL-2)(2nd ed.). Austin: Pro-Ed. Google Scholar
- Smerbeck, A.M., Parrish, J., Serafin, D., Yeh, E.A., Weinstock-Guttman, B., Hoogs, M., & Benedict, R.H. (2011). Visual-cognitive processing deficits in pediatric multiple sclerosis. Multiple Sclerosis, 17(4), 449–456. http://doi.org/10.1177/1352458510391689 CrossRef Google Scholar PubMed
- Smith, A. (1982). The Symbol Digit Modalities Test (SDMT) Manual. Los Angeles: Western Publication Services. Google Scholar
- Squillace, M., Ray, S., & Milazzo, M. (2015). Changes in gross grasp strength and fine motor skills in adolescents with pediatric multiple sclerosis. Occupational Therapy in Health Care, 29(1), 77–85. http://doi.org/10.3109/07380577.2014.967441 CrossRef Google Scholar PubMed
- Suppiej, A., Cainelli, E., Casara, G., Cappellari, A., Nosadini, M., & Sartori, S. (2014). Long-term neurocognitive outcome and quality of life in pediatric acute disseminated encephalomyelitis. Pediatric Neurology, 50(4), 363–367. http://doi.org/10.1016/j.pediatrneurol.2013.12.006; 10.1016/j.pediatrneurol.2013.12.006 CrossRef Google Scholar PubMed
- Tenembaum, S., Chamoles, N., & Fejerman, N. (2002). Acute disseminated encephalomyelitis: A long-term follow-up study of 84 pediatric patients. Neurology, 59(8), 1224–1231. CrossRef Google Scholar PubMed
- Till, C., Ghassemi, R., Aubert-Broche, B., Kerbrat, A., Collins, D.L., Narayanan, S., & Banwell, B.L. (2011). MRI correlates of cognitive impairment in childhood onset multiple sclerosis. Neuropsychology, 25(3), 319–332. CrossRef Google Scholar PubMed
- Till, C., Racine, N., Araujo, D., Narayanan, S., Collins, D.L., Aubert-Broche, B., & Banwell, B. (2013). Changes in cognitive performance over a 1-year period in children and adolescents with multiple sclerosis. Neuropsychology, 27(2), 210–219. http://doi.org/10.1037/a0031665 CrossRef Google Scholar
- Varni, J.W., Beaujean, A.A., & Limbers, C.A. (2013). Factorial invariance of pediatric patient self-reported fatigue across age and gender: a multigroup confirmatory factor analysis approach utilizing the PedsQL Multidimensional Fatigue Scale. Quality of Life Research, 22(9), 2581–2594. CrossRef Google Scholar
- Verhey, L.H., Branson, H.M., Laughlin, S., Shroff, M.M., Benseler, S.M., Feldman, B.M., & Banwell, B. (2013). Development of a standardized MRI scoring tool for CNS demyelination in children. AJNR American Journal of Neuroradiology, 34(6), 1271–1277. http://doi.org/10.3174/ajnr.A3382; 10.3174/ajnr.A3382 CrossRef Google Scholar PubMed
- Verhey, L.H., Branson, H.M., Shroff, M.M., Callen, D.J., Sled, J.G., Narayanan, S., . . . Canadian Pediatric Demyelinating Disease Network. (2011). MRI parameters for prediction of multiple sclerosis diagnosis in children with acute CNS demyelination: A prospective national cohort study. Lancet Neurology, 10(12), 1065–1073. http://doi.org/10.1016/S1474-4422(11)70250-2; 10.1016/S1474-4422(11)70250-2 CrossRef Google Scholar PubMed
- Wechsler, D. (1999). Wechsler Abbreviated Scale of Intelligence (WASI). San Antonio: The Psychological Corporation. Google Scholar
- Wingerchuk, D.M., Banwell, B., Bennett, J.L., Cabre, P., Carroll, W., Chitnis, T., & Weinshenker, B.G. (2015). International consensus diagnostic criteria for neuromyelitis optica spectrum disorders. Neurology, 85(2), 177–189. http://doi.org/10.1212/WNL.0000000000001729 CrossRef Google Scholar PubMed
- Woodcock, R.W., McGrew, K.S., & Mather, N. (2001). Woodcock Johnson III NU Tests of Cognitive Abilities. Rolling Meadows: Riverside Publishing. Google Scholar
Over the past several years, the concept of prodromal Parkinson disease (PD) has been increasingly recognized. This term refers to individuals who do not fulfill motor diagnostic criteria for PD, but who have clinical, genetic, or biomarker characteristics suggesting risk of developing PD in the future. Clinical diagnosis of prodromal PD has low specificity, prompting the need for objective biomarkers with higher specificity. In this qualitative review, we discuss objectively defined putative biomarkers for PD and prodromal PD.
We searched Pubmed and Embase for articles pertaining to objective biomarkers for PD and their application in prodromal cohorts. Articles were selected based on relevance and methodology.
Objective biomarkers of demonstrated utility in prodromal PD include ligand-based imaging and transcranial sonography. Development of serum, cerebrospinal fluid, and tissue-based biomarkers is underway, but their application in prodromal PD has yet to meaningfully occur. Combining objective biomarkers with clinical or genetic prodromal features increases the sensitivity and specificity for identifying prodromal PD.
Several objective biomarkers for prodromal PD show promise but require further study, including their application to and validation in prodromal cohorts followed longitudinally. Accurate identification of prodromal PD will likely require a multimodal approach. (JINS, 2016,22, 956–967)
- Ahmed, S.S., Santosh, W., Kumar, S., & Christlet, H.T. (2009). Metabolic profiling of Parkinson’s disease: Evidence of biomarker from gene expression analysis and rapid neural network detection. Journal of Biomedical Science, 16(1), 63. http://doi.org/10.1186/1423-0127-16-63 CrossRef Google Scholar
- Albin, R.L., Koeppe, R.A., Chervin, R.D., Consens, F.B., Wernette, K., Frey, K.A., & Aldrich, M.S. (2000). Decreased striatal dopaminergic innervation in REM sleep behavior disorder. Neurology, 55(9), 1410–1412. http://doi.org/10.1212/WNL.55.9.1410 CrossRef Google Scholar PubMed
- Ascherio, A., LeWitt, P.A., Xu, K., Eberly, S., Watts, A., Matson, W.R., ... Parkinson Study Group DATATOP Investigators. (2009). Urate as a predictor of the rate of clinical decline in Parkinson disease. Archives of Neurology, 66(12), 1460–1468. http://doi.org/10.1001/archneurol.2009.247 CrossRef Google Scholar PubMed
- Beach, T.G., Adler, C.H., Dugger, B.N., Serrano, G., Hidalgo, J., Henry-Watson, J., ... Arizona Parkinson’s Disease Consortium. (2013). Submandibular gland biopsy for the diagnosis of Parkinson disease. Journal of Neuropathology and Experimental Neurology, 72(2), 130–136. http://doi.org/10.1097/NEN.0b013e3182805c72 CrossRef Google Scholar
- Berg, D., Becker, G., Zeiler, B., Tucha, O., Hofmann, E., Preier, M., & Lange, K.W. (1999). Vulnerability of the nigrostriatal system as detected by transcranial ultrasound. Neurology, 53(5), 1026–1026. http://doi.org/10.1212/WNL.53.5.1026 CrossRef Google Scholar PubMed
- Berg, D., Godau, J., Seppi, K., Behnke, S., Liepelt-Scarfone, I., Lerche, S., … PRIPS study group. (2013). The PRIPS study: Screening battery for subjects at risk for Parkinson’s disease. European Journal of Neurology, 20(1), 102–108. http://doi.org/10.1111/j.1468-1331.2012.03798.x CrossRef Google Scholar PubMed
- Berg, D., Marek, K., Ross, G.W., & Poewe, W. (2012). Defining at-risk populations for Parkinson’s disease: Lessons from ongoing studies. Movement Disorders, 27(5), 656–665. http://doi.org/10.1002/mds.24985 CrossRef Google Scholar PubMed
- Berg, D., Postuma, R.B., Adler, C.H., Bloem, B.R., Chan, P., Dubois, B., & Deuschl, G. (2015). MDS research criteria for prodromal Parkinson’s disease. Movement Disorders, 30(12), 1600–1611. http://doi.org/10.1002/mds.26431 CrossRef Google Scholar PubMed
- Berg, D., Roggendorf, W., Schröder, U., Klein, R., Tatschner, T., Benz, P., & Becker, G. (2002). Echogenicity of the substantia nigra: Association with increased iron content and marker for susceptibility to nigrostriatal injury. Archives of Neurology, 59(6), 999–1005. CrossRef Google Scholar PubMed
- Berg, D., Siefker, C., & Becker, G. (2001). Echogenicity of the substantia nigra in Parkinson’s disease and its relation to clinical findings. Journal of Neurology, 248(8), 684–689. CrossRef Google Scholar PubMed
- Bernheimer, H., Birkmayer, W., Hornykiewicz, O., Jellinger, K., & Seitelberger, F. (1973). Brain dopamine and the syndromes of Parkinson and Huntington. Clinical, morphological and neurochemical correlations. Journal of the Neurological Sciences, 20(4), 415–455. CrossRef Google Scholar PubMed
- Boeve, B.F., Silber, M.H., Ferman, T.J., Lin, S.C., Benarroch, E.E., Schmeichel, A.M., & Dickson, D.W. (2013). Clinicopathologic correlations in 172 cases of rapid eye movement sleep behavior disorder with or without a coexisting neurologic disorder. Sleep Medicine, 14(8), 754–762. http://doi.org/10.1016/j.sleep.2012.10.015 CrossRef Google Scholar PubMed
- Bogdanov, M., Matson, W.R., Wang, L., Matson, T., Saunders-Pullman, R., Bressman, S.S., & Beal, M.F. (2008). Metabolomic profiling to develop blood biomarkers for Parkinson’s disease. Brain, 131(2), 389–396. http://doi.org/10.1093/brain/awm304 CrossRef Google Scholar PubMed
- Braak, H., Tredici, K.D., Rüb, U., de Vos, R.A.I., Jansen Steur, E.N.H., & Braak, E. (2003). Staging of brain pathology related to sporadic Parkinson’s disease. Neurobiology of Aging, 24(2), 197–211. http://doi.org/10.1016/S0197-4580(02)00065-9 CrossRef Google Scholar
- Brodacki, B., Staszewski, J., Toczyłowska, B., Kozłowska, E., Drela, N., Chalimoniuk, M., & Stepien, A. (2008). Serum interleukin (IL-2, IL-10, IL-6, IL-4), TNFα, and INFγ concentrations are elevated in patients with atypical and idiopathic parkinsonism. Neuroscience Letters, 441(2), 158–162. http://doi.org/10.1016/j.neulet.2008.06.040 CrossRef Google Scholar
- Brüggemann, N., Hagenah, J., Stanley, K., Klein, C., Wang, C., Raymond, D., & Saunders-Pullman, R. (2011). Substantia nigra hyperechogenicity with LRRK2 G2019S mutations. Movement Disorders, 26(5), 885–888. http://doi.org/10.1002/mds.23644 CrossRef Google Scholar PubMed
- Chahine, L.M., Qiang, J., Ashbridge, E., Minger, J., Yearout, D., Horn, S., & Chen-Plotkin, A. (2013). Clinical and biochemical differences in patients having Parkinson disease with vs without GBA mutations. JAMA Neurology, 70(7), 852–858. http://doi.org/10.1001/jamaneurol.2013.1274 CrossRef Google Scholar PubMed
- Chahine, L.M., Weintraub, D., Hawkins, K.A., Siderowf, A., Eberly, S., Oakes, D., … PARS Investigators. (2015). Cognition in individuals at risk for Parkinson’s: Parkinson associated risk syndrome (PARS) study findings. Movement Disorders, 31, 86–94. http://doi.org/10.1002/mds.26373 CrossRef Google Scholar PubMed
- Chen, H., O’Reilly, E.J., Schwarzschild, M.A., & Ascherio, A. (2008). Peripheral inflammatory biomarkers and risk of Parkinson’s disease. American Journal of Epidemiology, 167(1), 90–95. http://doi.org/10.1093/aje/kwm260 CrossRef Google Scholar PubMed
- Chikina, M.D., Gerald, C.P., Li, X., Ge, Y., Pincas, H., Nair, V.D., & Sealfon, S.C. (2015). Low-variance RNAs identify Parkinson’s disease molecular signature in blood. Movement Disorders, 30(6), 813–821. http://doi.org/10.1002/mds.26205 CrossRef Google Scholar PubMed
- Cochrane, C.J., & Ebmeier, K.P. (2013). Diffusion tensor imaging in parkinsonian syndromes: A systematic review and meta-analysis. Neurology, 80(9), 857–864. http://doi.org/10.1212/WNL.0b013e318284070c CrossRef Google Scholar PubMed
- Constantinescu, R., Rosengren, L., Johnels, B., Zetterberg, H., & Holmberg, B. (2010). Consecutive analyses of cerebrospinal fluid axonal and glial markers in Parkinson’s disease and atypical Parkinsonian disorders. Parkinsonism & Related Disorders, 16(2), 142–145. http://doi.org/10.1016/j.parkreldis.2009.07.007 CrossRef Google Scholar
- Davis, J.W., Grandinetti, A., Waslien, C.I., Ross, G.W., White, L.R., & Morens, D.M. (1996). Observations on serum uric acid levels and the risk of idiopathic Parkinson’s disease. American Journal of Epidemiology, 144(5), 480–484. CrossRef Google Scholar
- de Lau, L.M.L., Koudstaal, P.J., Hofman, A., & Breteler, M.M.B. (2005). Serum uric acid levels and the risk of Parkinson disease. Annals of Neurology, 58(5), 797–800. http://doi.org/10.1002/ana.20663 CrossRef Google Scholar PubMed
- Donadio, V., Incensi, A., Leta, V., Giannoccaro, M.P., Scaglione, C., Martinelli, P., & Liguori, R. (2014). Skin nerve α-synuclein deposits: A biomarker for idiopathic Parkinson disease. Neurology, 82(15), 1362–1369. http://doi.org/10.1212/WNL.0000000000000316 CrossRef Google Scholar PubMed
- Dursun, E., Gezen-Ak, D., Hanağası, H., Bilgiç, B., Lohmann, E., Ertan, S., & Yılmazer, S. (2015). The interleukin 1 alpha, interleukin 1 beta, interleukin 6 and alpha-2-macroglobulin serum levels in patients with early or late onset Alzheimer’s disease, mild cognitive impairment or Parkinson’s disease. Journal of Neuroimmunology, 283, 50–57. http://doi.org/10.1016/j.jneuroim.2015.04.014 CrossRef Google Scholar PubMed
- Eidelberg, D., Moeller, J.R., Dhawan, V., Spetsieris, P., Takikawa, S., Ishikawa, T., & Przedborski, S. (1994). The metabolic topography of parkinsonism. Journal of Cerebral Blood Flow and Metabolism, 14(5), 783–801. http://doi.org/10.1038/jcbfm.1994.99 CrossRef Google Scholar PubMed
- El-Agnaf, O.M.A., Salem, S.A., Paleologou, K.E., Curran, M.D., Gibson, M.J., Court, J.A., & Allsop, D. (2006). Detection of oligomeric forms of alpha-synuclein protein in human plasma as a potential biomarker for Parkinson’s disease. FASEB Journal, 20(3), 419–425. http://doi.org/10.1096/fj.03-1449com CrossRef Google Scholar PubMed
- Faucheux, B.A., Martin, M.-E., Beaumont, C., Hauw, J.-J., Agid, Y., & Hirsch, E.C. (2003). Neuromelanin associated redox-active iron is increased in the substantia nigra of patients with Parkinson’s disease. Journal of Neurochemistry, 86(5), 1142–1148. http://doi.org/10.1046/j.1471-4159.2003.01923.x CrossRef Google Scholar PubMed
- Fearnley, J.M., & Lees, A.J. (1991). Ageing and Parkinson’s disease: Substantia nigra regional selectivity. Brain, 114(5), 2283–2301. http://doi.org/10.1093/brain/114.5.2283 CrossRef Google Scholar PubMed
- Folgoas, E., Lebouvier, T., Leclair-Visonneau, L., Cersosimo, M.-G., Barthelaix, A., Derkinderen, P., & Letournel, F. (2013). Diagnostic value of minor salivary glands biopsy for the detection of Lewy pathology. Neuroscience Letters, 551, 62–64. http://doi.org/10.1016/j.neulet.2013.07.016 CrossRef Google Scholar PubMed
- Foulds, P.G., Mitchell, J.D., Parker, A., Turner, R., Green, G., Diggle, P., & Allsop, D. (2011). Phosphorylated α-synuclein can be detected in blood plasma and is potentially a useful biomarker for Parkinson’s disease. FASEB Journal, 25(12), 4127–4137. http://doi.org/10.1096/fj.10-179192 CrossRef Google Scholar PubMed
- Gaenslen, A., Swid, I., Liepelt-Scarfone, I., Godau, J., & Berg, D. (2011). The patients’ perception of prodromal symptoms before the initial diagnosis of Parkinson’s disease. Movement Disorders, 26(4), 653–658. http://doi.org/10.1002/mds.23499 CrossRef Google Scholar PubMed
- Gaenslen, A., Wurster, I., Brockmann, K., Huber, H., Godau, J., Faust, B., & Berg, D. (2014). Prodromal features for Parkinson’s disease--baseline data from the TREND study. European Journal of Neurology, 21(5), 766–772. http://doi.org/10.1111/ene.12382 CrossRef Google Scholar PubMed
- Gao, L., Tang, H., Nie, K., Wang, L., Zhao, J., Gan, R., & Wang, L. (2015). Cerebrospinal fluid alpha-synuclein as a biomarker for Parkinson’s disease diagnosis: A systematic review and meta-analysis. The International Journal of Neuroscience, 125(9), 645–654. http://doi.org/10.3109/00207454.2014.961454 CrossRef Google Scholar PubMed
- Gold, A., Turkalp, Z.T., & Munoz, D.G. (2013). Enteric alpha-synuclein expression is increased in Parkinson’s disease but not Alzheimer’s disease. Movement Disorders, 28(2), 237–240. http://doi.org/10.1002/mds.25298 CrossRef Google Scholar
- Hall, S., Öhrfelt, A., Constantinescu, R., Andreasson, U., Surova, Y., Bostrom, F., & Hansson, O. (2012). Accuracy of a panel of 5 cerebrospinal fluid biomarkers in the differential diagnosis of patients with dementia and/or parkinsonian disorders. Archives of Neurology, 69(11), 1445–1452. http://doi.org/10.1001/archneurol.2012.1654 CrossRef Google Scholar PubMed
- Herbert, M.K., Eeftens, J.M., Aerts, M.B., Esselink, R.A.J., Bloem, B.R., Kuiperij, H.B., & Verbeek, M.M. (2014). CSF levels of DJ-1 and tau distinguish MSA patients from PD patients and controls. Parkinsonism & Related Disorders, 20(1), 112–115. http://doi.org/10.1016/j.parkreldis.2013.09.003 CrossRef Google Scholar PubMed
- Hong, Z., Shi, M., Chung, K.A., Quinn, J.F., Peskind, E.R., Galasko, D., & Zhang, J. (2010). DJ-1 and α-synuclein in human cerebrospinal fluid as biomarkers of Parkinson’s disease. Brain, 133(3), 713–726. http://doi.org/10.1093/brain/awq008 CrossRef Google Scholar
- Huang, W.-S., Lin, S.-Z., Lin, J.-C., Wey, S.-P., Ting, G., & Liu, R.-S. (2001). Evaluation of early-stage Parkinson’s disease with 99mTc-TRODAT-1 imaging. Journal of Nuclear Medicine, 42(9), 1303–1308. Google Scholar PubMed
- Hughes, A.J., Daniel, S.E., Kilford, L., & Lees, A.J. (1992). Accuracy of clinical diagnosis of idiopathic Parkinson’s disease: A clinico-pathological study of 100 cases. Journal of Neurology, Neurosurgery, and Psychiatry, 55(3), 181–184. CrossRef Google Scholar PubMed
- Iranzo, A., Fernández-Arcos, A., Tolosa, E., Serradell, M., Molinuevo, J.L., Valldeoriola, F., & Santamaría, J. (2014). Neurodegenerative disorder risk in idiopathic REM sleep behavior disorder: Study in 174 patients. PloS One, 9(2), e89741. http://doi.org/10.1371/journal.pone.0089741 CrossRef Google Scholar PubMed
- Iranzo, A., Lomeña, F., Stockner, H., Valldeoriola, F., Vilaseca, I., Salamero, M., … Sleep Innsbruck Barcelona (SINBAR) group. (2010). Decreased striatal dopamine transporter uptake and substantia nigra hyperechogenicity as risk markers of synucleinopathy in patients with idiopathic rapid-eye-movement sleep behaviour disorder: A prospective study [corrected]. The Lancet. Neurology, 9(11), 1070–1077. http://doi.org/10.1016/S1474-4422(10)70216-7 CrossRef Google Scholar
- Jennings, D.L., Seibyl, J.P., Oakes, D., Eberly, S., Murphy, J., & Marek, K. (2004). (123i) β-cit and single-photon emission computed tomographic imaging vs clinical evaluation in parkinsonian syndrome: Unmasking an early diagnosis. Archives of Neurology, 61(8), 1224–1229. http://doi.org/10.1001/archneur.61.8.1224 CrossRef Google Scholar PubMed
- Jennings, D., Siderowf, A., Stern, M., Seibyl, J., Eberly, S., Oakes, D., … PARS Investigators. (2014). Imaging prodromal Parkinson disease The Parkinson Associated Risk Syndrome Study. Neurology, 83(19), 1739–1746. http://doi.org/10.1212/WNL.0000000000000960 CrossRef Google Scholar
- Jiménez-Jiménez, F.J., Alonso-Navarro, H., García-Martín, E., & Agúndez, J.A.G. (2014). Cerebrospinal fluid biochemical studies in patients with Parkinson’s disease: Toward a potential search for biomarkers for this disease. Frontiers in Cellular Neuroscience, 8, 369. http://doi.org/10.3389/fncel.2014.00369 Google Scholar
- Johansen, K.K., Wang, L., Aasly, J.O., White, L.R., Matson, W.R., Henchcliffe, C., & Bogdanov, M. (2009). Metabolomic profiling in LRRK2-related Parkinson’s disease. PLoS ONE, 4(10), e7551. http://doi.org/10.1371/journal.pone.0007551 CrossRef Google Scholar PubMed
- Kang, J.-H., Irwin, D.J., Chen-Plotkin, A.S., Siderowf, A., Caspell, C., Coffey, C.S., … Parkinson’s Progression Markers Initiative. (2013). Association of cerebrospinal fluid β-amyloid 1-42, T-tau, P-tau181, and α-synuclein levels with clinical features of drug-naive patients with early Parkinson disease. JAMA Neurology, 70(10), 1277–1287. http://doi.org/10.1001/jamaneurol.2013.3861 Google Scholar PubMed
- Kashihara, K., Imamura, T., & Shinya, T. (2010). Cardiac 123I-MIBG uptake is reduced more markedly in patients with REM sleep behavior disorder than in those with early stage Parkinson’s disease. Parkinsonism & Related Disorders, 16(4), 252–255. http://doi.org/10.1016/j.parkreldis.2009.12.010 CrossRef Google Scholar PubMed
- Khan, N.L., Valente, E.M., Bentivoglio, A.R., Wood, N.W., Albanese, A., Brooks, D.J., & Piccini, P. (2002). Clinical and subclinical dopaminergic dysfunction in PARK6-linked parkinsonism: An 18F-dopa PET study. Annals of Neurology, 52(6), 849–853. http://doi.org/10.1002/ana.10417 CrossRef Google Scholar
- Lebouvier, T., Chaumette, T., Damier, P., Coron, E., Touchefeu, Y., Vrignaud, S., & Neunlist, M. (2008). Pathological lesions in colonic biopsies during Parkinson’s disease. Gut, 57(12), 1741–1743. http://doi.org/10.1136/gut.2008.162503 CrossRef Google Scholar PubMed
- Liepelt, I., Behnke, S., Schweitzer, K., Wolf, B., Godau, J., Wollenweber, F., & Berg, D. (2011). Pre-motor signs of PD are related to SN hyperechogenicity assessed by TCS in an elderly population. Neurobiology of Aging, 32(9), 1599–1606. http://doi.org/10.1016/j.neurobiolaging.2009.10.004 CrossRef Google Scholar
- Lin, X., Cook, T.J., Zabetian, C.P., Leverenz, J.B., Peskind, E.R., Hu, S.-C., & Zhang, J. (2012). DJ-1 isoforms in whole blood as potential biomarkers of Parkinson disease. Scientific Reports, 2, 954. http://doi.org/10.1038/srep00954 CrossRef Google Scholar PubMed
- Loeffler, D.A., Smith, L.M., Coffey, M.P., Aasly, J.O., & LeWitt, P.A. (2015). CSF Nrf2 and HSPA8 in Parkinson’s disease patients with and without LRRK2 gene mutations. Journal of Neural Transmission (Vienna, Austria: 1996). http://doi.org/10.1007/s00702-015-1479-0 Google Scholar PubMed
- Malek, N., Swallow, D., Grosset, K.A., Anichtchik, O., Spillantini, M., & Grosset, D.G. (2014). Alpha-synuclein in peripheral tissues and body fluids as a biomarker for Parkinson’s disease - A systematic review. Acta Neurologica Scandinavica, 130(2), 59–72. http://doi.org/10.1111/ane.12247 CrossRef Google Scholar PubMed
- Marek, K., Jennings, D., Lasch, S., Siderowf, A., Tanner, C., Simuni, T., & Taylor, P. (2011). The Parkinson Progression Marker Initiative (PPMI). Progress in Neurobiology, 95(4), 629–635. http://doi.org/10.1016/j.pneurobio.2011.09.005 CrossRef Google Scholar
- Miyamoto, T., Miyamoto, M., Inoue, Y., Usui, Y., Suzuki, K., & Hirata, K. (2006). Reduced cardiac 123I-MIBG scintigraphy in idiopathic REM sleep behavior disorder. Neurology, 67(12), 2236–2238. http://doi.org/10.1212/01.wnl.0000249313.25627.2e CrossRef Google Scholar
- Moccia, M., Picillo, M., Erro, R., Vitale, C., Longo, K., Amboni, M., & Pellecchia, M.T. (2014). Is serum uric acid related to non-motor symptoms in de-novo Parkinson’s disease patients? Parkinsonism & Related Disorders, 20(7), 772–775. http://doi.org/10.1016/j.parkreldis.2014.03.016 CrossRef Google Scholar
- Moccia, M., Picillo, M., Erro, R., Vitale, C., Longo, K., Amboni, M., & Pellecchia, M.T. (2015). Presence and progression of non-motor symptoms in relation to uric acid in de novo Parkinson’s disease. European Journal of Neurology, 22(1), 93–98. http://doi.org/10.1111/ene.12533 CrossRef Google Scholar
- Mollenhauer, B., Parnetti, L., Rektorova, I., Kramberger, M.G., Pikkarainen, M., Schulz-Schaeffer, W.J., & Schlossmacher, M.G. (2015). Biological confounders for the values of cerebrospinal fluid proteins in Parkinson’s disease and related disorders. Journal of Neurochemistry, http://doi.org/10.1111/jnc.13390 Google Scholar PubMed
- Nishioka, K., Hayashi, S., Farrer, M.J., Singleton, A.B., Yoshino, H., Imai, H., & Hattori, N. (2006). Clinical heterogeneity of alpha-synuclein gene duplication in Parkinson’s disease. Annals of Neurology, 59(2), 298–309. http://doi.org/10.1002/ana.20753 CrossRef Google Scholar PubMed
- Nishioka, K., Ross, O.A., Ishii, K., Kachergus, J.M., Ishiwata, K., Kitagawa, M., & Hattori, N. (2009). Expanding the clinical phenotype of SNCA duplication carriers. Movement Disorders, 24(12), 1811–1819. http://doi.org/10.1002/mds.22682 CrossRef Google Scholar
- Noyce, A.J., Bestwick, J.P., Silveira-Moriyama, L., Hawkes, C.H., Knowles, C.H., Hardy, J., & Schrag, A. (2014). PREDICT-PD: Identifying risk of Parkinson’s disease in the community: Methods and baseline results. Journal of Neurology, Neurosurgery, and Psychiatry, 85(1), 31–37. http://doi.org/10.1136/jnnp-2013-305420 CrossRef Google Scholar
- Öhman, A., & Forsgren, L. (2015). NMR metabonomics of cerebrospinal fluid distinguishes between Parkinson’s disease and controls. Neuroscience Letters, 594, 36–39. http://doi.org/10.1016/j.neulet.2015.03.051 CrossRef Google Scholar
- Ohtsuka, C., Sasaki, M., Konno, K., Koide, M., Kato, K., Takahashi, J., & Terayama, Y. (2013). Changes in substantia nigra and locus coeruleus in patients with early-stage Parkinson’s disease using neuromelanin-sensitive MR imaging. Neuroscience Letters, 541, 93–98. http://doi.org/10.1016/j.neulet.2013.02.012 CrossRef Google Scholar PubMed
- Orimo, S., Amino, T., Yokochi, M., Kojo, T., Uchihara, T., Takahashi, A., & Mizuno, Y. (2005). Preserved cardiac sympathetic nerve accounts for normal cardiac uptake of MIBG in PARK2. Movement Disorders, 20(10), 1350–1353. http://doi.org/10.1002/mds.20594 CrossRef Google Scholar PubMed
- Ponsen, M.M., Stoffers, D., Twisk, J.W.R., Wolters, E.C., & Berendse, H.W. (2009). Hyposmia and executive dysfunction as predictors of future Parkinson’s disease: A prospective study. Movement Disorders, 24(7), 1060–1065. http://doi.org/10.1002/mds.22534 CrossRef Google Scholar
- Ponsen, M.M., Stoffers, D., Wolters, E.C., Booij, J., & Berendse, H.W. (2010). Olfactory testing combined with dopamine transporter imaging as a method to detect prodromal Parkinson’s disease. Journal of Neurology, Neurosurgery, & Psychiatry, 81(4), 396–399. http://doi.org/10.1136/jnnp.2009.183715 CrossRef Google Scholar
- Postuma, R.B., Aarsland, D., Barone, P., Burn, D.J., Hawkes, C.H., Oertel, W., & Ziemssen, T. (2012). Identifying prodromal Parkinson’s disease: Pre-motor disorders in Parkinson’s disease. Movement Disorders: Official Journal of the Movement Disorder Society, 27(5), 617–626. http://doi.org/10.1002/mds.24996 CrossRef Google Scholar PubMed
- Postuma, R.B., Gagnon, J.-F., Pelletier, A., & Montplaisir, J. (2013). Prodromal autonomic symptoms and signs in Parkinson’s disease and dementia with Lewy bodies. Movement Disorders, 28(5), 597–604. http://doi.org/10.1002/mds.25445 CrossRef Google Scholar PubMed
- Postuma, R.B., Gagnon, J.F., Vendette, M., Fantini, M.L., Massicotte-Marquez, J., & Montplaisir, J. (2009). Quantifying the risk of neurodegenerative disease in idiopathic REM sleep behavior disorder. Neurology, 72(15), 1296–1300. http://doi.org/10.1212/01.wnl.0000340980.19702.6e CrossRef Google Scholar PubMed
- Pouclet, H., Lebouvier, T., Coron, E., Des Varannes, S.B., Neunlist, M., & Derkinderen, P. (2012). A comparison between colonic submucosa and mucosa to detect Lewy pathology in Parkinson’s disease. Neurogastroenterology & Motility, 24(4), e202–e205. http://doi.org/10.1111/j.1365-2982.2012.01887.x CrossRef Google Scholar PubMed
- Pyle, A., Brennan, R., Kurzawa-Akanbi, M., Yarnall, A., Thouin, A., Mollenhauer, B., & Hudson, G. (2015). Reduced CSF mitochondrial DNA is a biomarker for early-stage Parkinson’s disease. Annals of Neurology. http://doi.org/10.1002/ana.24515 CrossRef Google Scholar PubMed
- Qiang, J.K., Wong, Y.C., Siderowf, A., Hurtig, H.I., Xie, S.X., Lee, V.M.-Y., & Chen-Plotkin, A.S. (2013). Plasma Apolipoprotein A1 as a Biomarker for Parkinson’s Disease. Annals of Neurology, 74(1), 119–127. http://doi.org/10.1002/ana.23872 CrossRef Google Scholar
- Quattrone, A., Bagnato, A., Annesi, G., Novellino, F., Morgante, L., Savettieri, G., & Condino, F. (2008). Myocardial 123metaiodobenzylguanidine uptake in genetic Parkinson’s disease. Movement Disorders, 23(1), 21–27. http://doi.org/10.1002/mds.21701 CrossRef Google Scholar PubMed
- Ravina, B., Marek, K., Eberly, S., Oakes, D., Kurlan, R., Ascherio, A., & Shoulson, I. (2012). Dopamine transporter imaging is associated with long-term outcomes in Parkinson’s disease. Movement Disorders, 27(11), 1392–1397. http://doi.org/10.1002/mds.25157 CrossRef Google Scholar
- Rodríguez-Leyva, I., Calderón-Garcidueñas, A.L., Jiménez-Capdeville, M.E., Rentería-Palomo, A.A., Hernandez-Rodriguez, H.G., Valdés-Rodríguez, R., & Castanedo-Cázares, J.P. (2014). α-Synuclein inclusions in the skin of Parkinson’s disease and parkinsonism. Annals of Clinical and Translational Neurology, 1(7), 471–478. http://doi.org/10.1002/acn3.78 CrossRef Google Scholar PubMed
- Ross, G.W., Abbott, R.D., Petrovitch, H., Tanner, C.M., & White, L.R. (2012). Pre-motor features of Parkinson’s disease: the The Honolulu-Asia Aging Study experience. Parkinsonism & Related Disorders, 18(Suppl. 1), S199–S202. http://doi.org/10.1016/S1353-8020(11)70062-1 CrossRef Google Scholar PubMed
- Sako, W., Murakami, N., Izumi, Y., & Kaji, R. (2015). Neurofilament light chain level in cerebrospinal fluid can differentiate Parkinson’s disease from atypical parkinsonism: Evidence from a meta-analysis. Journal of the Neurological Sciences, 352(1-2), 84–87. http://doi.org/10.1016/j.jns.2015.03.041 CrossRef Google Scholar PubMed
- Sasaki, M., Shibata, E., Tohyama, K., Takahashi, J., Otsuka, K., Tsuchiya, K., & Sakai, A. (2006). Neuromelanin magnetic resonance imaging of locus ceruleus and substantia nigra in Parkinson’s disease. Neuroreport, 17(11), 1215–1218. http://doi.org/10.1097/01.wnr.0000227984.84927.a7 CrossRef Google Scholar PubMed
- Sawada, H., Oeda, T., Yamamoto, K., Kitagawa, N., Mizuta, E., Hosokawa, R., & Kawamura, T. (2009). Diagnostic accuracy of cardiac metaiodobenzylguanidine scintigraphy in Parkinson disease. European Journal of Neurology, 16(2), 174–182. http://doi.org/10.1111/j.1468-1331.2008.02372.x CrossRef Google Scholar PubMed
- Schenck, C.H., Boeve, B.F., & Mahowald, M.W. (2013). Delayed emergence of a parkinsonian disorder or dementia in 81% of older men initially diagnosed with idiopathic rapid eye movement sleep behavior disorder: A 16-year update on a previously reported series. Sleep Medicine, 14(8), 744–748. http://doi.org/10.1016/j.sleep.2012.10.009 CrossRef Google Scholar PubMed
- Scherfler, C., Frauscher, B., Schocke, M., Iranzo, A., Gschliesser, V., Seppi, K., … for the SINBAR (Sleep Innsbruck Barcelona) Group. (2011). White and gray matter abnormalities in idiopathic rapid eye movement sleep behavior disorder: A diffusion-tensor imaging and voxel-based morphometry study. Annals of Neurology, 69(2), 400–407. http://doi.org/10.1002/ana.22245 CrossRef Google Scholar
- Scherzer, C.R., Eklund, A.C., Morse, L.J., Liao, Z., Locascio, J.J., Fefer, D., & Gullans, S.R. (2007). Molecular markers of early Parkinson’s disease based on gene expression in blood. Proceedings of the National Academy of Sciences of the United States of America, 104(3), 955–960. http://doi.org/10.1073/pnas.0610204104 CrossRef Google Scholar PubMed
- Schwarzschild, M.A., Schwid, S.R., Marek, K., Watts, A., Lang, A.E., Oakes, D., & Ondrasik, J. (2008). Serum urate as a predictor of clinical and radiographic progression in Parkinson disease. Archives of Neurology, 65(6), 716–723. http://doi.org/10.1001/archneur.2008.65.6.nct70003 CrossRef Google Scholar PubMed
- Seibyl, J.P., Marek, K.L., Quinlan, D., Sheff, K., Zoghbi, S., Zea-Ponce, Y., & van Dyck, C. (1995). Decreased single-photon emission computed tomographic [123I]beta-CIT striatal uptake correlates with symptom severity in Parkinson’s disease. Annals of Neurology, 38(4), 589–598. http://doi.org/10.1002/ana.410380407 CrossRef Google Scholar
- Seibyl, J.P., Marek, K., Sheff, K., Zoghbi, S., Baldwin, R.M., Charney, D.S., & Innis, R.B. (1998). Iodine-123-beta-CIT and iodine-123-FPCIT SPECT measurement of dopamine transporters in healthy subjects and Parkinson’s patients. Journal of Nuclear Medicine, 39(9), 1500–1508. Google Scholar PubMed
- Shannon, K.M., Keshavarzian, A., Dodiya, H.B., Jakate, S., & Kordower, J.H. (2012). Is alpha-synuclein in the colon a biomarker for premotor Parkinson’s disease? Evidence from 3 cases. Movement Disorders, 27(6), 716–719. http://doi.org/10.1002/mds.25020 CrossRef Google Scholar
- Shi, M., Zabetian, C.P., Hancock, A.M., Ginghina, C., Hong, Z., Yearout, D., & Zhang, J. (2010). Significance and confounders of peripheral DJ-1 and alpha-synuclein in Parkinson’s disease. Neuroscience Letters, 480(1), 78–82. http://doi.org/10.1016/j.neulet.2010.06.009 CrossRef Google Scholar
- Siderowf, A., & Lang, A.E. (2012). Premotor Parkinson’s disease: Concepts and definitions. Movement Disorders, 27(5), 608–616. http://doi.org/10.1002/mds.24954 CrossRef Google Scholar PubMed
- Siderowf, A., & Stern, M.B. (2008). Premotor Parkinson’s disease: Clinical features, detection, and prospects for treatment. Annals of Neurology, 64(S2), S139–S147. http://doi.org/10.1002/ana.21462 CrossRef Google Scholar
- Sofic, E., Riederer, P., Heinsen, H., Beckmann, H., Reynolds, G.P., Hebenstreit, G., & Youdim, M.B. (1988). Increased iron (III) and total iron content in post mortem substantia nigra of parkinsonian brain. Journal of Neural Transmission, 74(3), 199–205. CrossRef Google Scholar
- Sprenger, F.S., Stefanova, N., Gelpi, E., Seppi, K., Navarro-Otano, J., Offner, F., & Poewe, W. (2015). Enteric nervous system α-synuclein immunoreactivity in idiopathic REM sleep behavior disorder. Neurology, 85(20), 1761–1768. http://doi.org/10.1212/WNL.0000000000002126 CrossRef Google Scholar
- Stephenson, R., Siderowf, A., & Stern, M.B. (2009). Premotor Parkinson’s disease: Clinical features and detection strategies. Movement Disorders, 24(S2), S665–S670. http://doi.org/10.1002/mds.22403 CrossRef Google Scholar PubMed
- Stern, M.B., & Siderowf, A. (2010). Parkinson’s at risk syndrome: Can Parkinson’s disease be predicted? Movement Disorders, 25(S1), S89–S93. http://doi.org/10.1002/mds.22719 CrossRef Google Scholar PubMed
- Tredici, K.D., Hawkes, C.H., Ghebremedhin, E., & Braak, H. (2010). Lewy pathology in the submandibular gland of individuals with incidental Lewy body disease and sporadic Parkinson’s disease. Acta Neuropathologica, 119(6), 703–713. http://doi.org/10.1007/s00401-010-0665-2 CrossRef Google Scholar PubMed
- Tunc, S., Graf, J., Tadic, V., Brüggemann, N., Schmidt, A., Al-Khaled, M., & Kasten, M. (2015). A population-based study on combined markers for early Parkinson’s disease. Movement Disorders, 30(4), 531–537. http://doi.org/10.1002/mds.26100 CrossRef Google Scholar
- Visanji, N.P., Marras, C., Hazrati, L.-N., Liu, L.W.C., & Lang, A.E. (2014). Alimentary, my dear Watson? The challenges of enteric α-synuclein as a Parkinson’s disease biomarker. Movement Disorders, 29(4), 444–450. http://doi.org/10.1002/mds.25789 CrossRef Google Scholar PubMed
- Visanji, N.P., Marras, C., Kern, D.S., Al Dakheel, A., Gao, A., Liu, L.W.C., & Hazrati, L.-N. (2015). Colonic mucosal a-synuclein lacks specificity as a biomarker for Parkinson disease. Neurology, 84(6), 609–616. http://doi.org/10.1212/WNL.0000000000001240 CrossRef Google Scholar PubMed
- Walter, U., Klein, C., Hilker, R., Benecke, R., Pramstaller, P.P., & Dressler, D. (2004). Brain parenchyma sonography detects preclinical parkinsonism. Movement Disorders, 19(12), 1445–1449. http://doi.org/10.1002/mds.20232 CrossRef Google Scholar PubMed
- Wang, J.-J., Lin, W.-Y., Lu, C.-S., Weng, Y.-H., Ng, S.-H., Wang, C.-H., & Wai, Y.-Y. (2011). Parkinson disease: Diagnostic utility of diffusion kurtosis imaging. Radiology, 261(1), 210–217. http://doi.org/10.1148/radiol.11102277 CrossRef Google Scholar PubMed
- Wang, X., Yu, S., Li, F., & Feng, T. (2015). Detection of α-synuclein oligomers in red blood cells as a potential biomarker of Parkinson’s disease. Neuroscience Letters, 599, 115–119. http://doi.org/10.1016/j.neulet.2015.05.030 CrossRef Google Scholar PubMed
- Waragai, M., Nakai, M., Wei, J., Fujita, M., Mizuno, H., Ho, G., & Hashimoto, M. (2007). Plasma levels of DJ-1 as a possible marker for progression of sporadic Parkinson’s disease. Neuroscience Letters, 425(1), 18–22. http://doi.org/10.1016/j.neulet.2007.08.010 CrossRef Google Scholar PubMed
- Weisskopf, M.G., O’Reilly, E., Chen, H., Schwarzschild, M.A., & Ascherio, A. (2007). Plasma Urate and Risk of Parkinson’s Disease. American Journal of Epidemiology, 166(5), 561–567. http://doi.org/10.1093/aje/kwm127 CrossRef Google Scholar PubMed
- Wing, Y.K., Lam, S.P., Zhang, J., Leung, E., Ho, C.L., Chen, S., & Ho, C.K.W. (2015). Reduced striatal dopamine transmission in REM sleep behavior disorder comorbid with depression. Neurology, 84(5), 516–522. http://doi.org/10.1212/WNL.0000000000001215 CrossRef Google Scholar
- Wu, P., Yu, H., Peng, S., Dauvilliers, Y., Wang, J., Ge, J., & Zuo, C. (2014). Consistent abnormalities in metabolic network activity in idiopathic rapid eye movement sleep behaviour disorder. Brain, 137(12), 3122–3128. http://doi.org/10.1093/brain/awu290 CrossRef Google Scholar
- Zhou, B., Wen, M., Yu, W.-F., Zhang, C.-L., & Jiao, L. (2015). The diagnostic and differential diagnosis utility of cerebrospinal fluid α-Synuclein levels in Parkinson’s disease: A meta-analysis. Parkinson’s Disease, 2015, 567386. http://doi.org/10.1155/2015/567386 Google Scholar PubMed